A Deep Learning Framework for Wind Turbine Repair Action Prediction Using Alarm Sequences and Long Short Term Memory Algorithms
MODEL-BASED SAFETY AND ASSESSMENT, IMBSA 2022(2022)
摘要
With an increasing emphasis on driving down the costs of Operations and Maintenance (O&M) in the Offshore Wind (OSW) sector, comes the requirement to explore new methodology and applications of Deep Learning (DL) to the domain. Condition-based monitoring (CBM) has been at the forefront of recent research developing alarmbased systems and data-driven decision making. This paper provides a brief insight into the research being conducted in this area, with a specific focus on alarm sequence modelling and the associated challenges faced in its implementation. The paper proposes a novel idea to predict a set of relevant repair actions from an input sequence of alarm sequences, comparing Long Short-term Memory (LSTM) and Bidirectional LSTM (biLSTM) models. Achieving training accuracy results of up to 80.23%, and test accuracy results of up to 76.01% with biLSTM gives a strong indication to the potential benefits of the proposed approach that can be furthered in future research. The paper introduces a framework that integrates the proposed approach into O&M procedures and discusses the potential benefits which include the reduction of a confusing plethora of alarms, as well as unnecessary vessel transfers to the turbines for fault diagnosis and correction.
更多查看译文
关键词
Condition-based monitoring (cbm), Deep Learning (dl), Long Short-term Memory (lstm), Offshore Wind Farm (osw), Repair action prediction, Supervisory Control and Data Acquisition (scada)
AI 理解论文
溯源树
样例
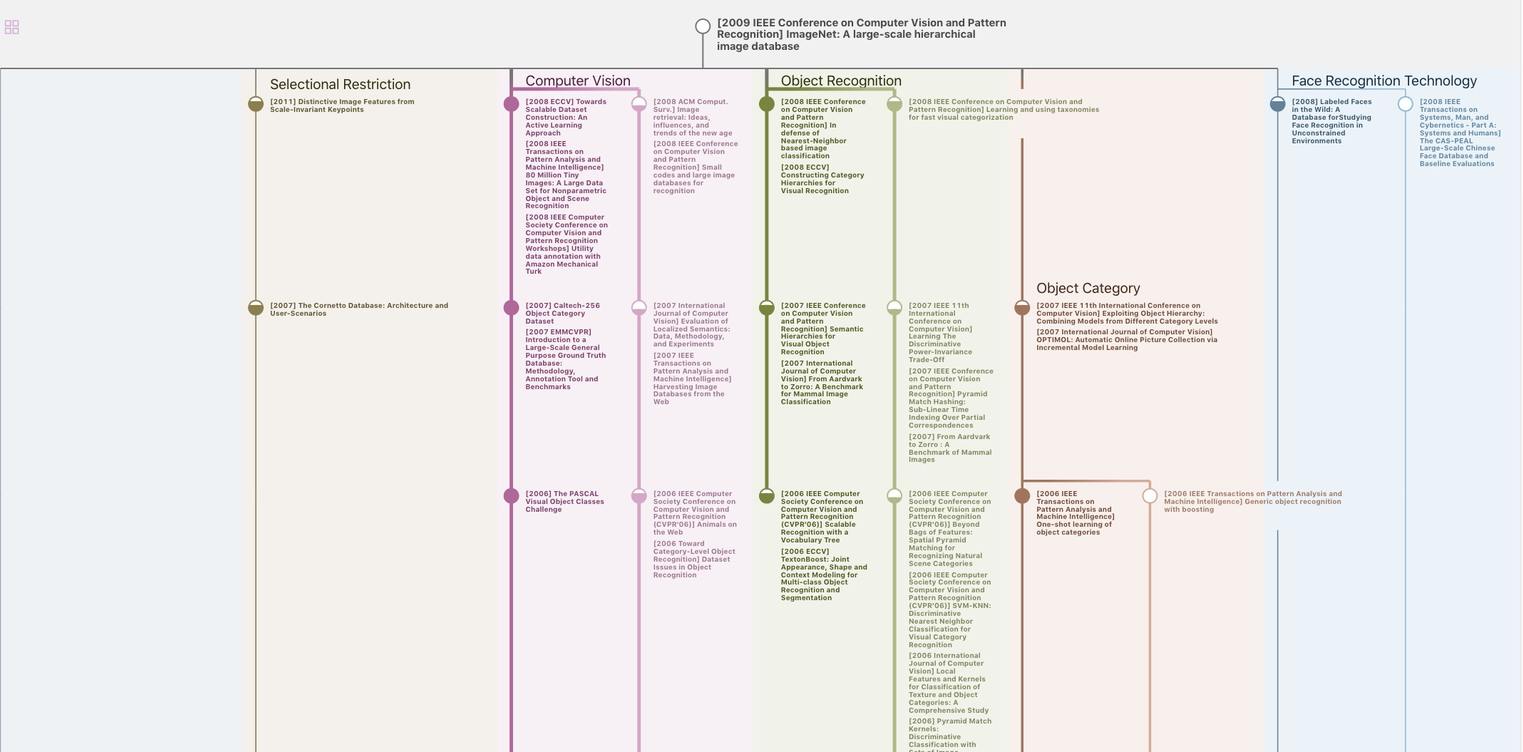
生成溯源树,研究论文发展脉络
Chat Paper
正在生成论文摘要