Neural Interferometry: Image Reconstruction from Astronomical Interferometers Using Transformer-Conditioned Neural Fields.
AAAI Conference on Artificial Intelligence(2022)
摘要
Astronomical interferometry enables a collection of telescopes to achieve angular resolutions comparable to that of a single, much larger telescope. This is achieved by combining simultaneous observations from pairs of telescopes such that the signal is mathematically equivalent to sampling the Fourier domain of the object. However, reconstructing images from such sparse sampling is a challenging and ill-posed problem, with current methods requiring precise tuning of parameters and manual, iterative cleaning by experts. We present a novel deep learning approach in which the representation in the Fourier domain of an astronomical source is learned implicitly using a neural field representation. Data-driven priors can be added through a transformer encoder. Results on synthetically observed galaxies show that transformer-conditioned neural fields can successfully reconstruct astronomical observations even when the number of visibilities is very sparse.
更多查看译文
关键词
Computer Vision (CV),Domain(s) Of Application (APP)
AI 理解论文
溯源树
样例
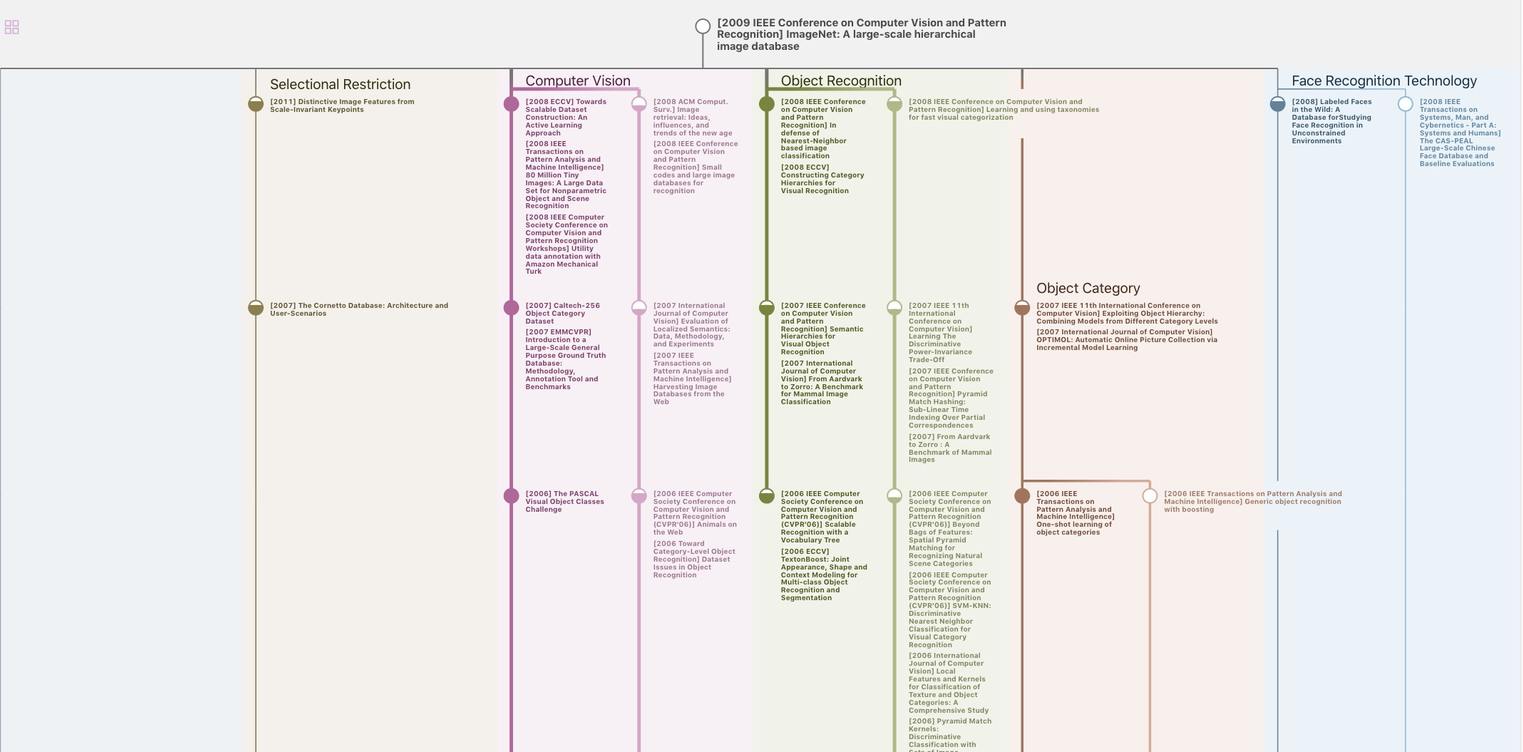
生成溯源树,研究论文发展脉络
Chat Paper
正在生成论文摘要