Defect Transformer: An Efficient Hybrid Transformer Architecture for Surface Defect Detection
arxiv(2022)
摘要
Surface defect detection is an extremely crucial step to ensure the quality of industrial products. Nowadays, convolutional neural networks (CNNs) based on encoder-decoder architecture have achieved tremendous success in various defect detection tasks. However, due to the intrinsic locality of convolution, they commonly exhibit a limitation in explicitly modeling long-range interactions, critical for pixel-wise defect detection in complex cases, e.g., cluttered background and illegible pseudo-defects. Recent transformers are especially skilled at learning global image dependencies but with limited local structural information necessary for detailed defect location. To overcome the above limitations, we propose an efficient hybrid transformer architecture, termed Defect Transformer (DefT), for surface defect detection, which incorporates CNN and transformer into a unified model to capture local and non-local relationships collaboratively. Specifically, in the encoder module, a convolutional stem block is firstly adopted to retain more detailed spatial information. Then, the patch aggregation blocks are used to generate multi-scale representation with four hierarchies, each of them is followed by a series of DefT blocks, which respectively include a locally position-aware block for local position encoding, a lightweight multi-pooling self-attention to model multi-scale global contextual relationships with good computational efficiency, and a convolutional feed-forward network for feature transformation and further location information learning. Finally, a simple but effective decoder module is proposed to gradually recover spatial details from the skip connections in the encoder. Extensive experiments on three datasets demonstrate the superiority and efficiency of our method compared with other CNN- and transformer-based networks.
更多查看译文
关键词
Surface defect detection,Transformer,Convolutional neural network,Global contextual relationship,Local position encoding
AI 理解论文
溯源树
样例
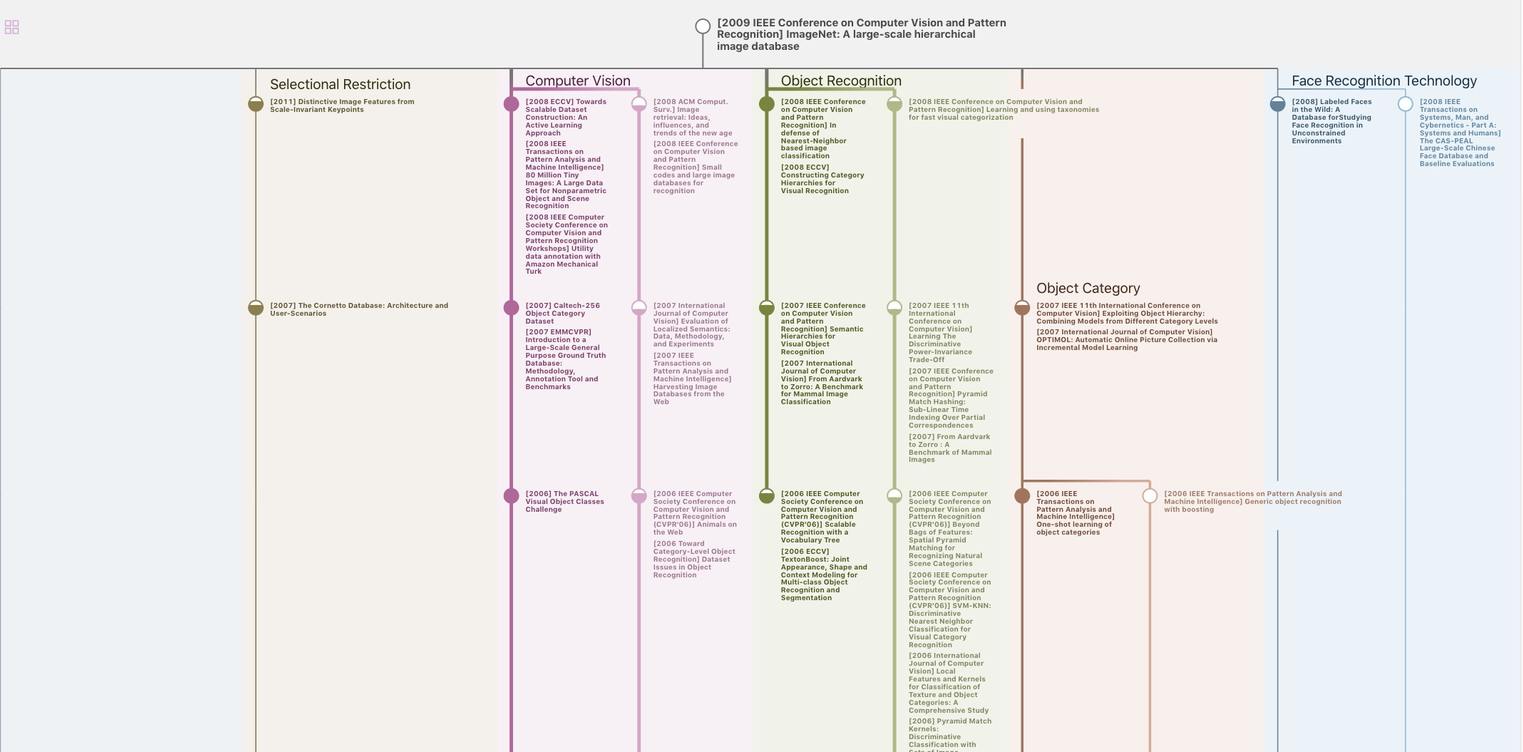
生成溯源树,研究论文发展脉络
Chat Paper
正在生成论文摘要