Collaborative Learning in Kernel-Based Bandits for Distributed Users
IEEE TRANSACTIONS ON SIGNAL PROCESSING(2023)
摘要
We study collaborative learning among distributed clients facilitated by a central server. Each client is interested in maximizing a personalized objective function that is a weighted sum of its local objective and a global objective. Each client has direct access to random bandit feedback on its local objective, but only has a partial view of the global objective and relies on information exchange with other clients for collaborative learning. We adopt the kernel-based bandit framework where the objective functions belong to a reproducing kernel Hilbert space. We propose an algorithm based on surrogate Gaussian process (GP) models and establish its order-optimal regret performance (up to polylogarithmic factors). We also show that the sparse approximations of the GP models can be employed to reduce the communication overhead across clients.
更多查看译文
关键词
Distributed learning,kernel-based bandits,personalization
AI 理解论文
溯源树
样例
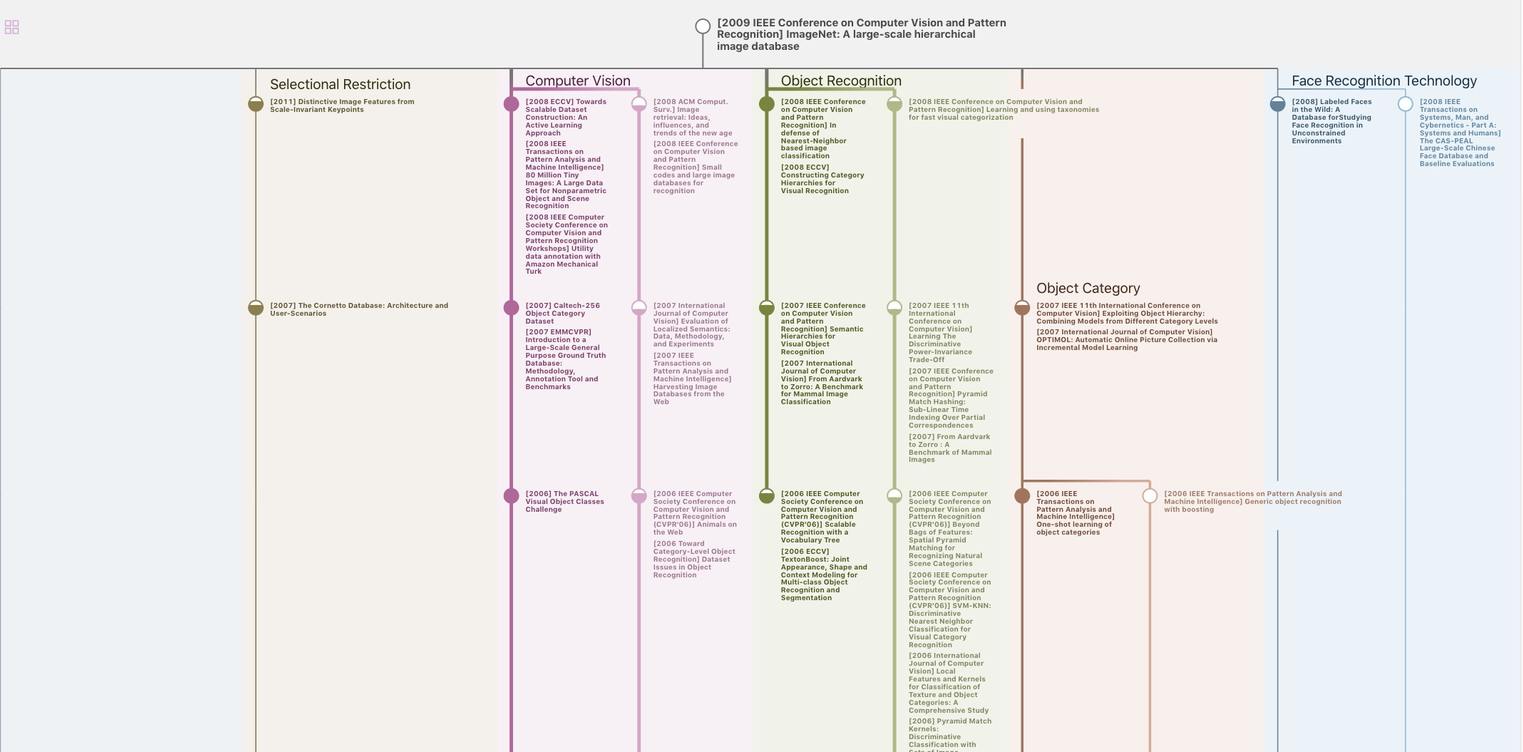
生成溯源树,研究论文发展脉络
Chat Paper
正在生成论文摘要