Low-Energy Electron-Track Imaging for a Liquid Argon Time-Projection-Chamber Telescope Concept using Probabilistic Deep Learning
arxiv(2022)
摘要
The GammaTPC is an MeV-scale single-phase liquid argon time-projection-chamber gamma-ray telescope concept with a novel dual-scale pixel-based charge-readout system. It promises to enable a significant improvement in sensitivity to MeV-scale gamma-rays over previous telescopes. The novel pixel-based charge readout allows for imaging of the tracks of electrons scattered by Compton interactions of incident gamma-rays. The two primary contributors to the accuracy of a Compton telescope in reconstructing an incident gamma-ray's original direction are its energy and position resolution. In this work, we focus on using deep learning to optimize the reconstruction of the initial position and direction of electrons scattered in Compton interactions, including using probabilistic models to estimate predictive uncertainty. We show that the deep learning models are able to predict locations of Compton scatters of MeV-scale gamma-rays from simulated pixel-based data to better than 0.6 mm RMS error, and are sensitive to the initial direction of the scattered electron. We compare and contrast different deep learning uncertainty estimation algorithms for reconstruction applications. Additionally, we show that event-by-event estimates of the uncertainty of the locations of the Compton scatters can be used to select those events that were reconstructed most accurately, leading to improvement in locating the origin of gamma-ray sources on the sky.
更多查看译文
关键词
Gamma-ray telescopes,Gamma-ray transient sources,Convolutional neural networks,Gamma-ray detectors,Astronomy image processing
AI 理解论文
溯源树
样例
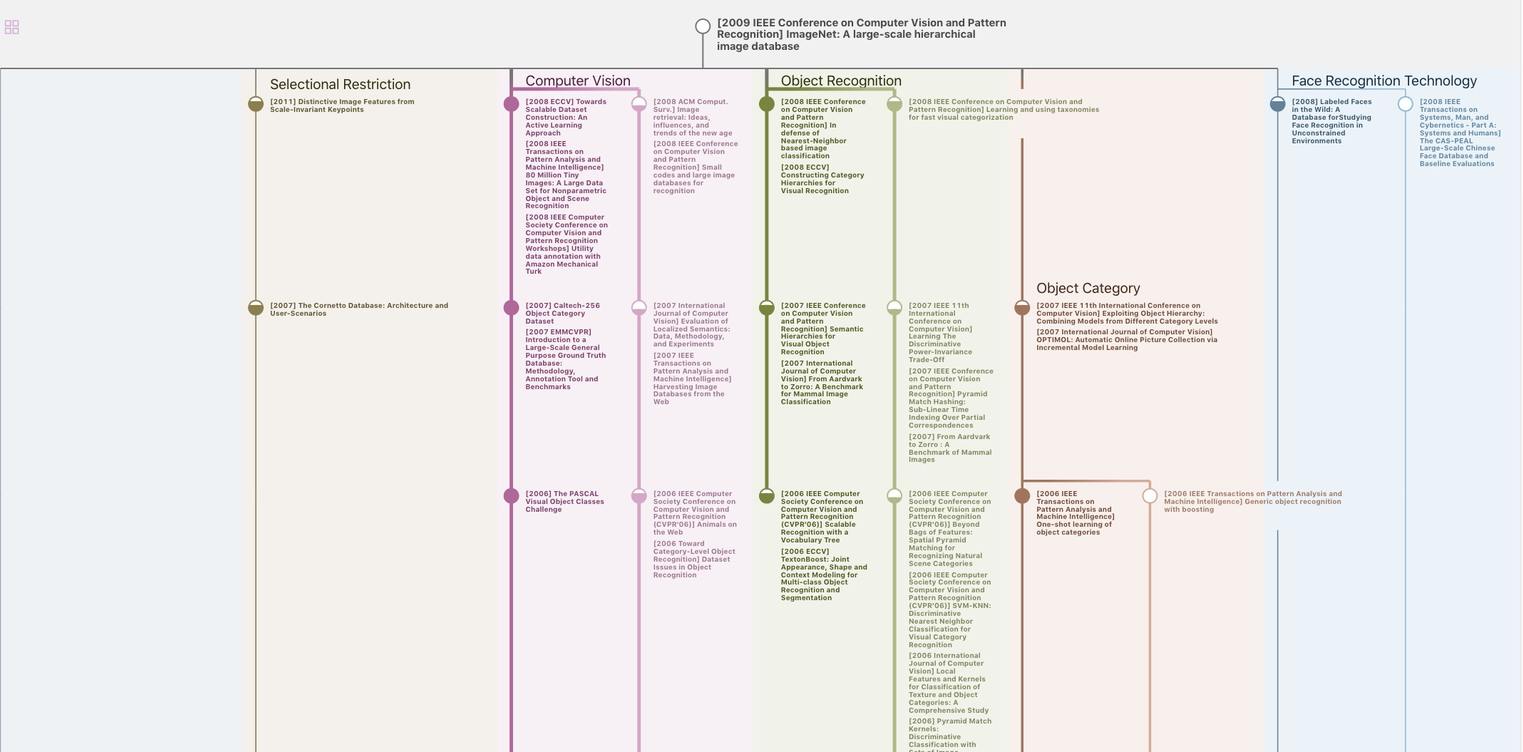
生成溯源树,研究论文发展脉络
Chat Paper
正在生成论文摘要