Detecting bots in social-networks using node and structural embeddings
PROCEEDINGS OF THE 11TH INTERNATIONAL CONFERENCE ON DATA SCIENCE, TECHNOLOGY AND APPLICATIONS (DATA)(2023)
摘要
Users on social networks such as Twitter interact with each other without much knowledge of the real-identity behind the accounts they interact with. This anonymity has created a perfect environment for bot accounts to influence the network by mimicking real-user behaviour. Although not all bot accounts have malicious intent, identifying bot accounts in general is an important and difficult task. In the literature there are three distinct types of feature sets one could use for building machine learning models for classifying bot accounts. These feature-sets are: user profile metadata, natural language features ( NLP ) extracted from user tweets and finally features extracted from the the underlying social network. Profile metadata and NLP features are typically explored in detail in the bot-detection literature. At the same time less attention has been given to the predictive power of features that can be extracted from the underlying network structure. To fill this gap we explore and compare two classes of embedding algorithms that can be used to take advantage of information that network structure provides. The first class are classical embedding techniques, which focus on learning proximity information. The second class are structural embedding algorithms, which capture the local structure of node neighbourhood. We show that features created using structural embeddings have higher predictive power when it comes to bot detection. This supports the hypothesis that the local social network formed around bot accounts on Twitter contains valuable information that can be used to identify bot accounts.
更多查看译文
关键词
Detecting bots,Node and structural embeddings,Social networks
AI 理解论文
溯源树
样例
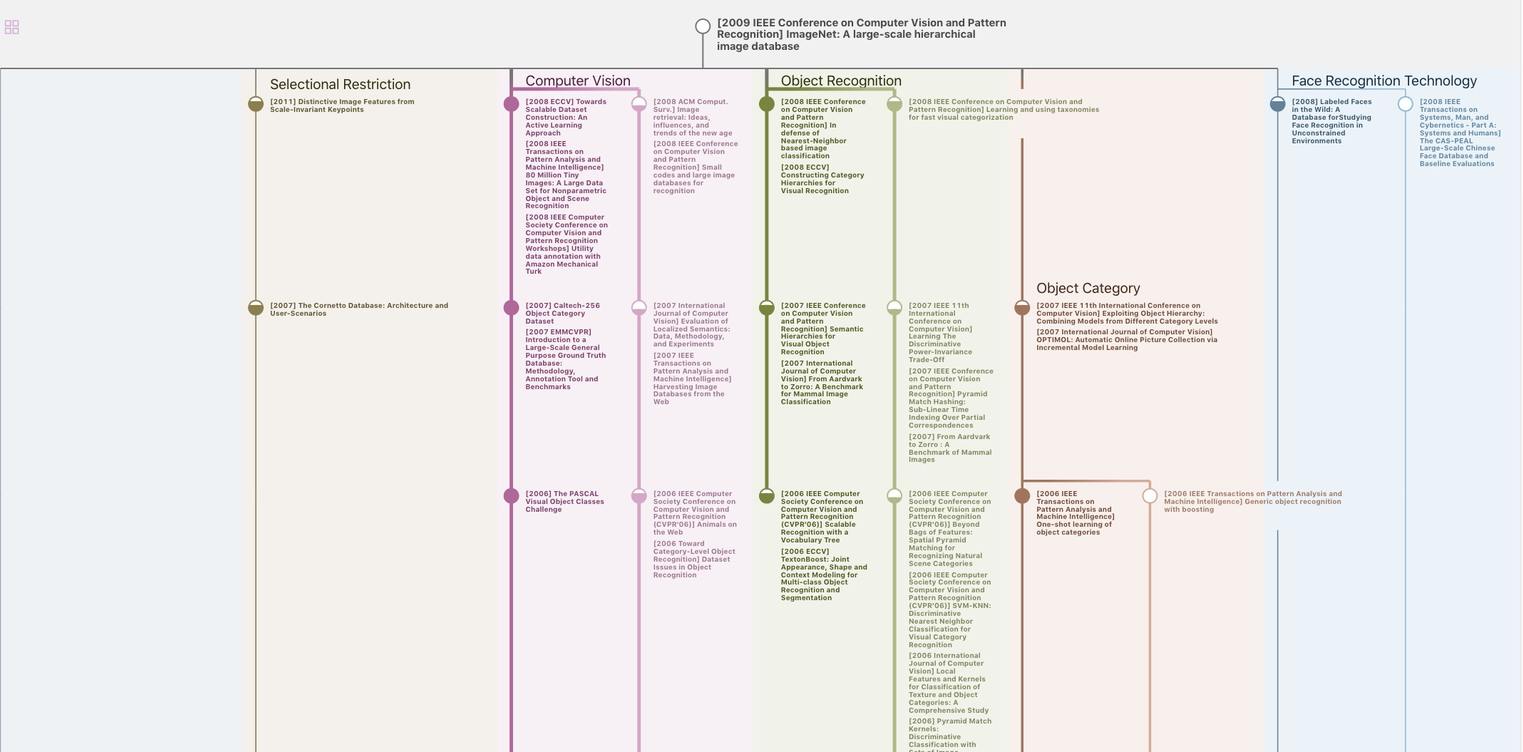
生成溯源树,研究论文发展脉络
Chat Paper
正在生成论文摘要