Robust Incremental Bayesian Learning Based Online Flux Linkage Estimation for PMSM Drives
IEEE Transactions on Transportation Electrification(2022)
摘要
For the permanent magnet synchronous machine (PMSM), parameter estimation can be greatly affected by the measurement uncertainty, but few efforts are made to reduce the uncertainty level for estimation performance improvement. Therefore, this article proposes an efficient and robust incremental Bayesian learning approach for PMSM parameter estimation. The measurement uncertainty is evaluated to guide the selection of informative measurements, and the estimation uncertainty is provided to indicate the confidence in using the estimated results. Specifically, a Bayesian learning strategy with a layered noise model is proposed for nonlinear flux linkage estimation. The measurement uncertainty level is estimated from the proposed Bayesian learning model, which is utilized to adaptively select the most informative data and delete the noninformative data for parameter estimation. This contributes to improving estimation accuracy and computation efficiency. Moreover, the estimation uncertainty is also determined by the proposed model, which can be used to indicate if the estimated results can be trusted and utilized in practical applications. The proposed approach is evaluated on a laboratory interior PMSM under various operating conditions.
更多查看译文
关键词
Data selection,measurement uncertainty,parameter estimation,permanent magnet synchronous machine (PMSM),robust incremental Bayesian learning
AI 理解论文
溯源树
样例
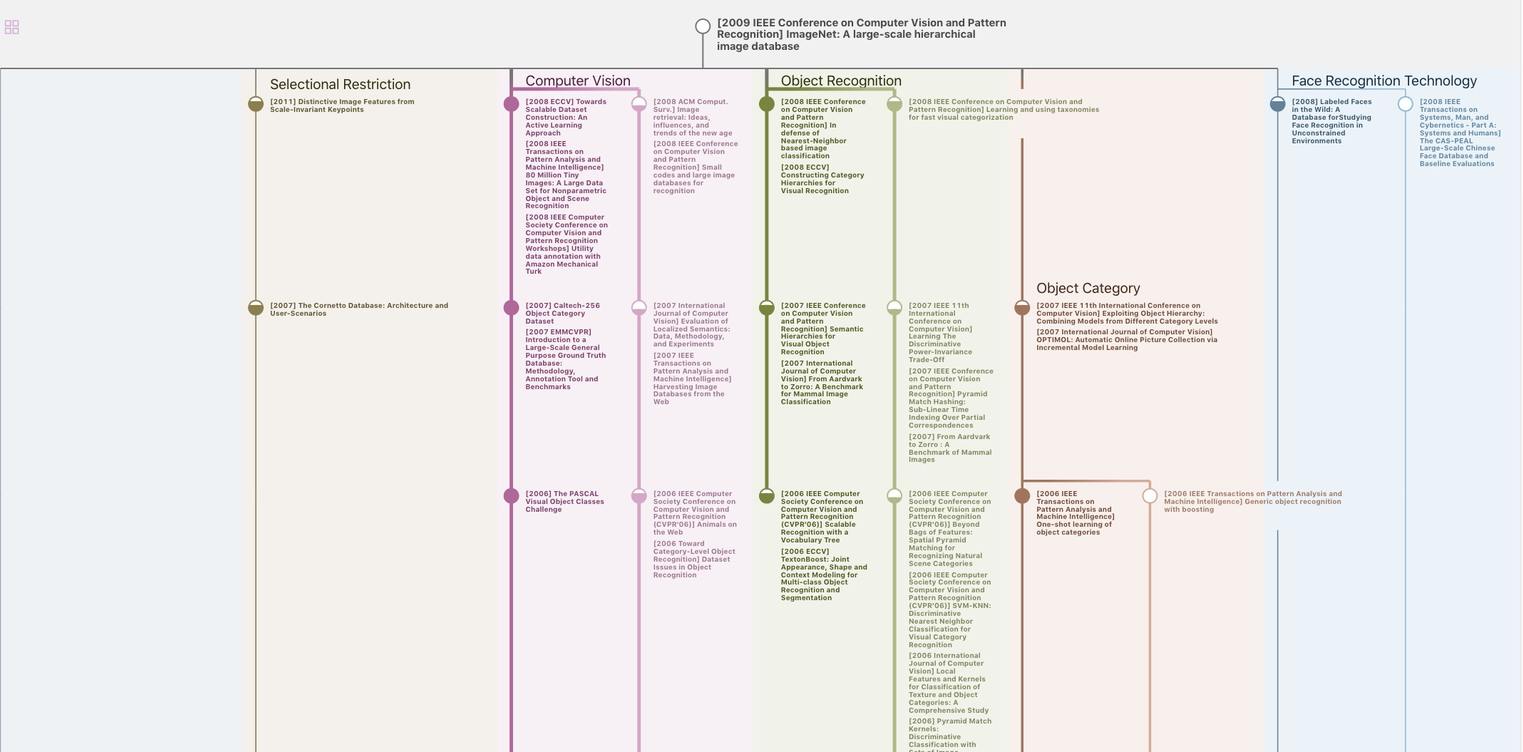
生成溯源树,研究论文发展脉络
Chat Paper
正在生成论文摘要