Multimodal Open-Vocabulary Video Classification via Pre-Trained Vision and Language Models
arxiv(2022)
摘要
Utilizing vision and language models (VLMs) pre-trained on large-scale image-text pairs is becoming a promising paradigm for open-vocabulary visual recognition. In this work, we extend this paradigm by leveraging motion and audio that naturally exist in video. We present \textbf{MOV}, a simple yet effective method for \textbf{M}ultimodal \textbf{O}pen-\textbf{V}ocabulary video classification. In MOV, we directly use the vision encoder from pre-trained VLMs with minimal modifications to encode video, optical flow and audio spectrogram. We design a cross-modal fusion mechanism to aggregate complimentary multimodal information. Experiments on Kinetics-700 and VGGSound show that introducing flow or audio modality brings large performance gains over the pre-trained VLM and existing methods. Specifically, MOV greatly improves the accuracy on base classes, while generalizes better on novel classes. MOV achieves state-of-the-art results on UCF and HMDB zero-shot video classification benchmarks, significantly outperforming both traditional zero-shot methods and recent methods based on VLMs. Code and models will be released.
更多查看译文
关键词
classification,video,language,open-vocabulary,pre-trained
AI 理解论文
溯源树
样例
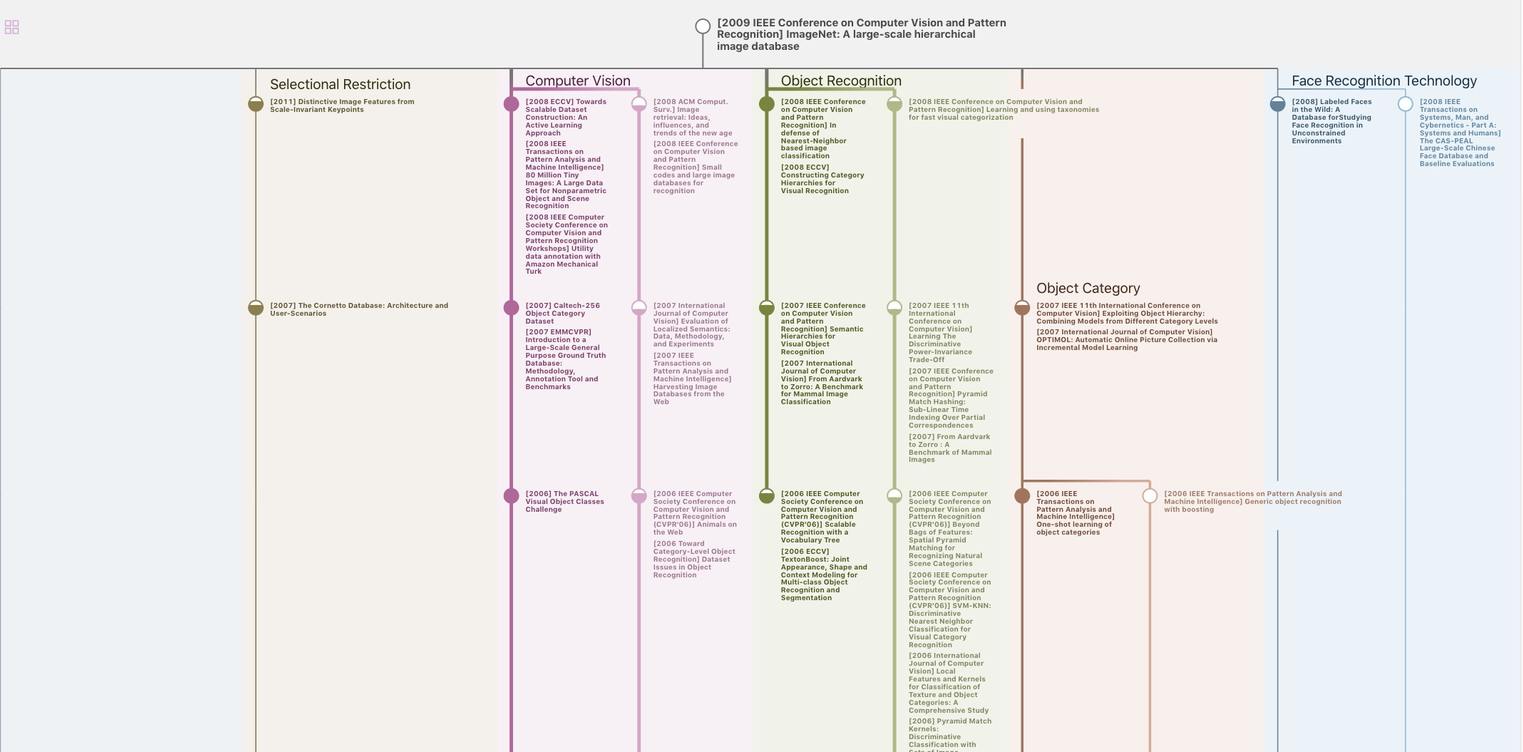
生成溯源树,研究论文发展脉络
Chat Paper
正在生成论文摘要