A scalable anticipatory policy for the dynamic pickup and delivery problem
COMPUTERS & OPERATIONS RESEARCH(2022)
摘要
Dynamic vehicle dispatching and routing problems can be tackled by using either reactive policies (that optimize the overall inconvenience on the pending requests) or anticipatory policies (that consider the possible future demands). The anticipatory policies reported in the literature are typically unsuitable for the large instances often encountered in the real-world, where the inter-arrival time can be as little as a few seconds. In this article, we present a new scalable anticipatory policy for the Dynamic Pickup and Delivery Problem which amounts to design routes for a fleet of vehicles that must service a set of pickup and delivery requests, characterized by different priority classes, arriving according to an unknown (possibly time-varying) stochastic process. The algorithm utilizes a parametric policy function approximation in which the best parameter setting is chosen on-line on the basis of a mapping between instance features and policy parameters learned off-line by using simulation experiments. Computational results on large-scale randomly-generated instances indicate that our anticipatory procedure outperforms two reactive approaches while keeping the computational burden at a level suitable for real-world usage.
更多查看译文
关键词
Routing,Dynamic pickup and delivery,Anticipatory policies,Parametric policy function approximation,Supervised learning
AI 理解论文
溯源树
样例
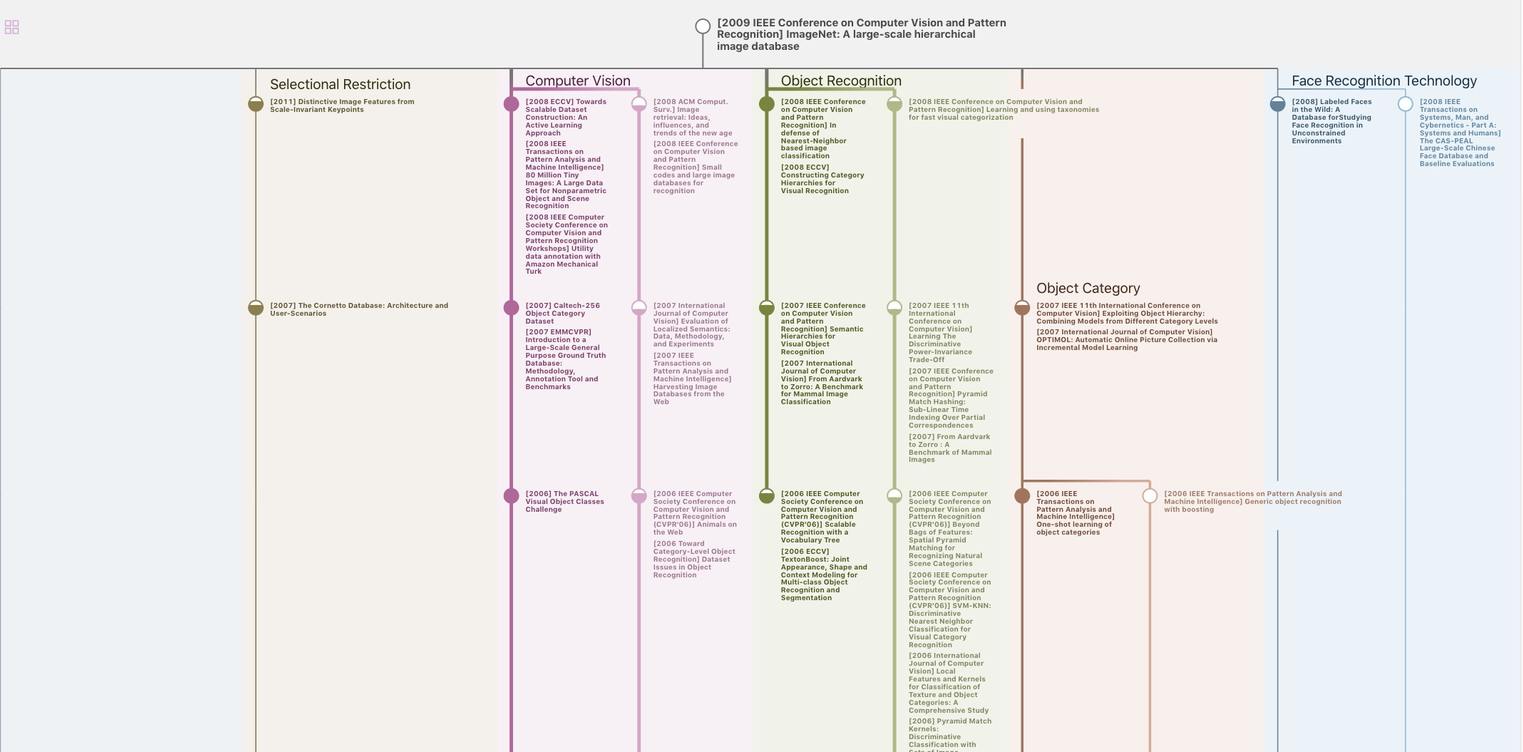
生成溯源树,研究论文发展脉络
Chat Paper
正在生成论文摘要