Linear Substitution Pruning: Consider All Filters Together
Proceedings of the 8th International Conference on Computing and Artificial Intelligence(2022)
摘要
Filter (neuron) pruning is a neural network compression approach. In previous work, the importance of each filter is generally considered individually or pairwisely. This paper shows that considering the linear relation among all filters can help us prune more efficiently. Based on this intuition, we propose a new filter pruning method, named Linear Substitution Pruning (LSP). Similar to LSP, we also propose a model compensation method, called Linear Substitution Compensation (LSC), which restores the model performance after pruning by using all remaining filters to compensate for the error caused by pruning. The experiments show that our method outperforms the state-of-the-art filter pruning methods. LSP achieves a reduction of 61.04% in flops on ResNet110 with an increase of 0.96% in top-1 accuracy at the same time, and it achieves a reduction of 51.84% in flops on ResNet50 with also an increase of 0.05% in top-1 accuracy.
更多查看译文
AI 理解论文
溯源树
样例
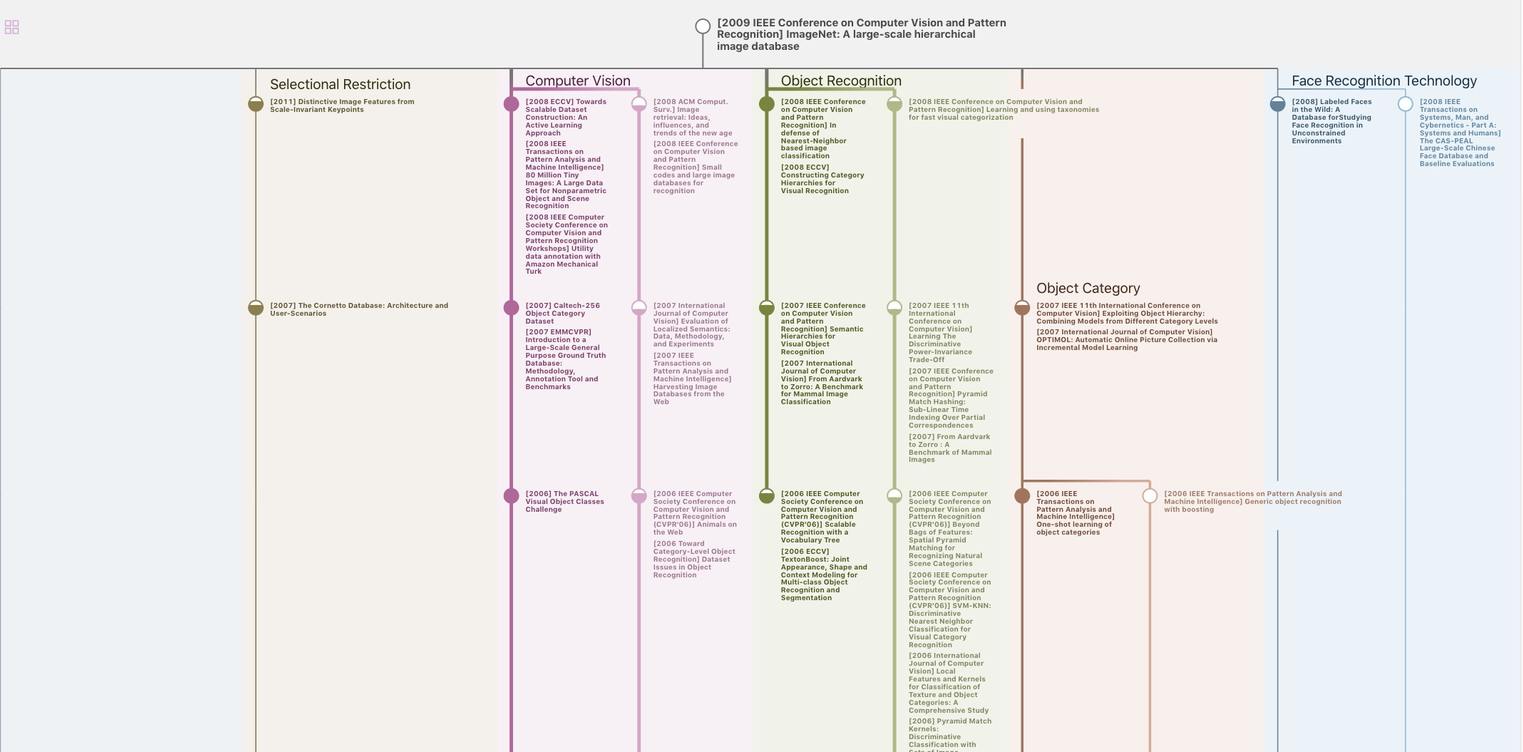
生成溯源树,研究论文发展脉络
Chat Paper
正在生成论文摘要