Multi-View Graph Clustering by Adaptive Manifold Learning
MATHEMATICS(2022)
摘要
Graph-oriented methods have been widely adopted in multi-view clustering because of their efficiency in learning heterogeneous relationships and complex structures hidden in data. However, existing methods are typically investigated based on a Euclidean structure instead of a more suitable manifold topological structure. Hence, it is expected that a more suitable manifold topological structure will be adopted to carry out intrinsic similarity learning. In this paper, we explore the implied adaptive manifold for multi-view graph clustering. Specifically, our model seamlessly integrates multiple adaptive graphs into a consensus graph with the manifold topological structure considered. We further manipulate the consensus graph with a useful rank constraint so that its connected components precisely correspond to distinct clusters. As a result, our model is able to directly achieve a discrete clustering result without any post-processing. In terms of the clustering results, our method achieves the best performance in 22 out of 24 cases in terms of four evaluation metrics on six datasets, which demonstrates the effectiveness of the proposed model. In terms of computational performance, our optimization algorithm is generally faster or in line with other state-of-the-art algorithms, which validates the efficiency of the proposed algorithm.
更多查看译文
关键词
multi-view learning, graph learning, clustering, manifold learning
AI 理解论文
溯源树
样例
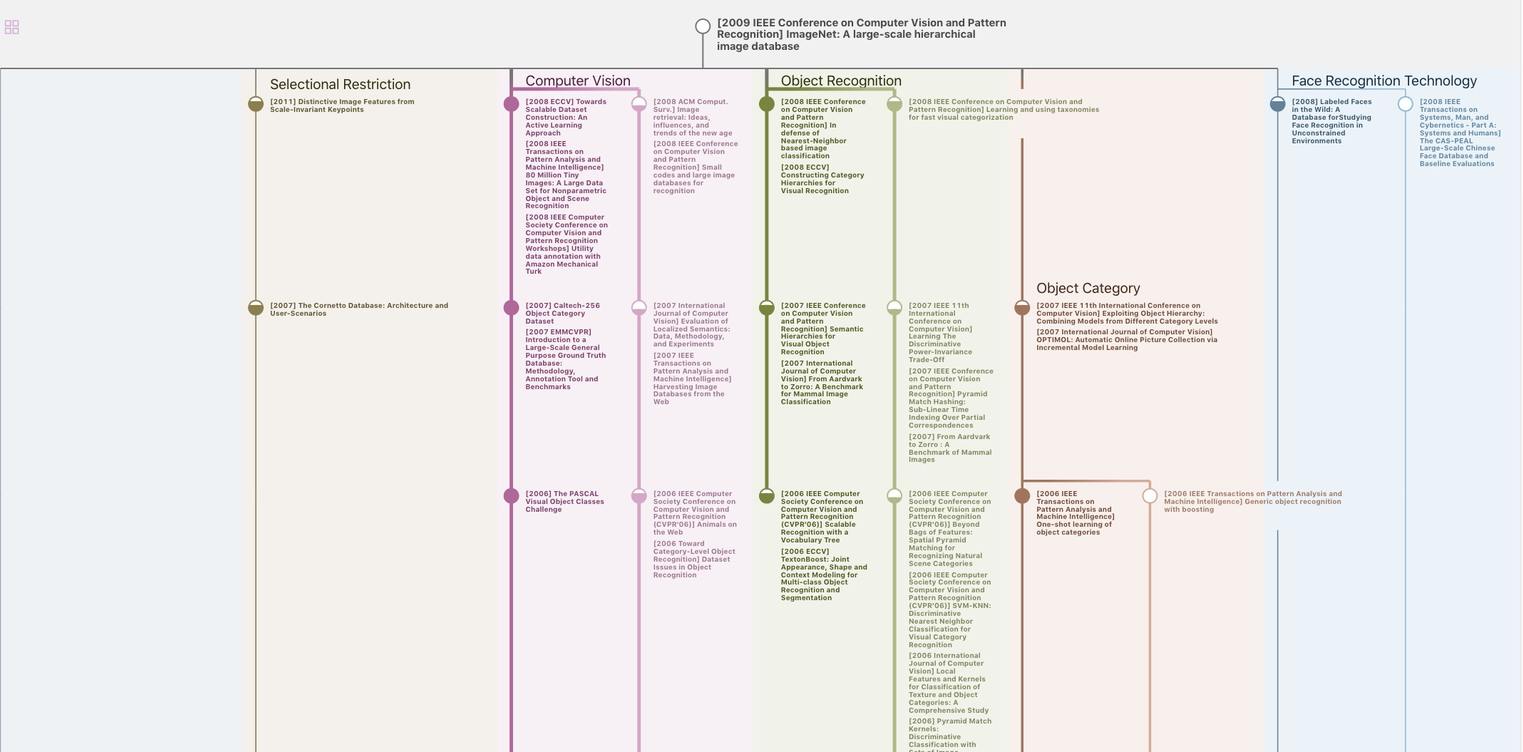
生成溯源树,研究论文发展脉络
Chat Paper
正在生成论文摘要