Analog synaptic devices applied to spiking neural networks for reinforcement learning applications
SEMICONDUCTOR SCIENCE AND TECHNOLOGY(2022)
摘要
In this work, we implement hardware-based spiking neural network (SNN) using the thin-film transistor (TFT)-type flash synaptic devices. A hardware-based SNN architecture with synapse arrays and integrate-and-fire (I&F) neuron circuits is presented for executing reinforcement learning (RL). Two problems were used to evaluate the applicability of the proposed hardware-based SNNs to off-chip RL: the Cart Pole balancing problem and the Rush Hour problem. The neural network was trained using a deep Q-learning algorithm. The proposed hardware-based SNNs using the synapse model with measured characteristics successfully solve the two problems and show high performance, implying that the networks are suitable for executing RL. Furthermore, the effect of variations in non-ideal synaptic devices and neurons on the performance was investigated.
更多查看译文
关键词
hardware-based spiking neural networks (SNNs), reinforcement learning (RL), deep Q-learning algorithm, neuromorphic, TFT-type flash synaptic device
AI 理解论文
溯源树
样例
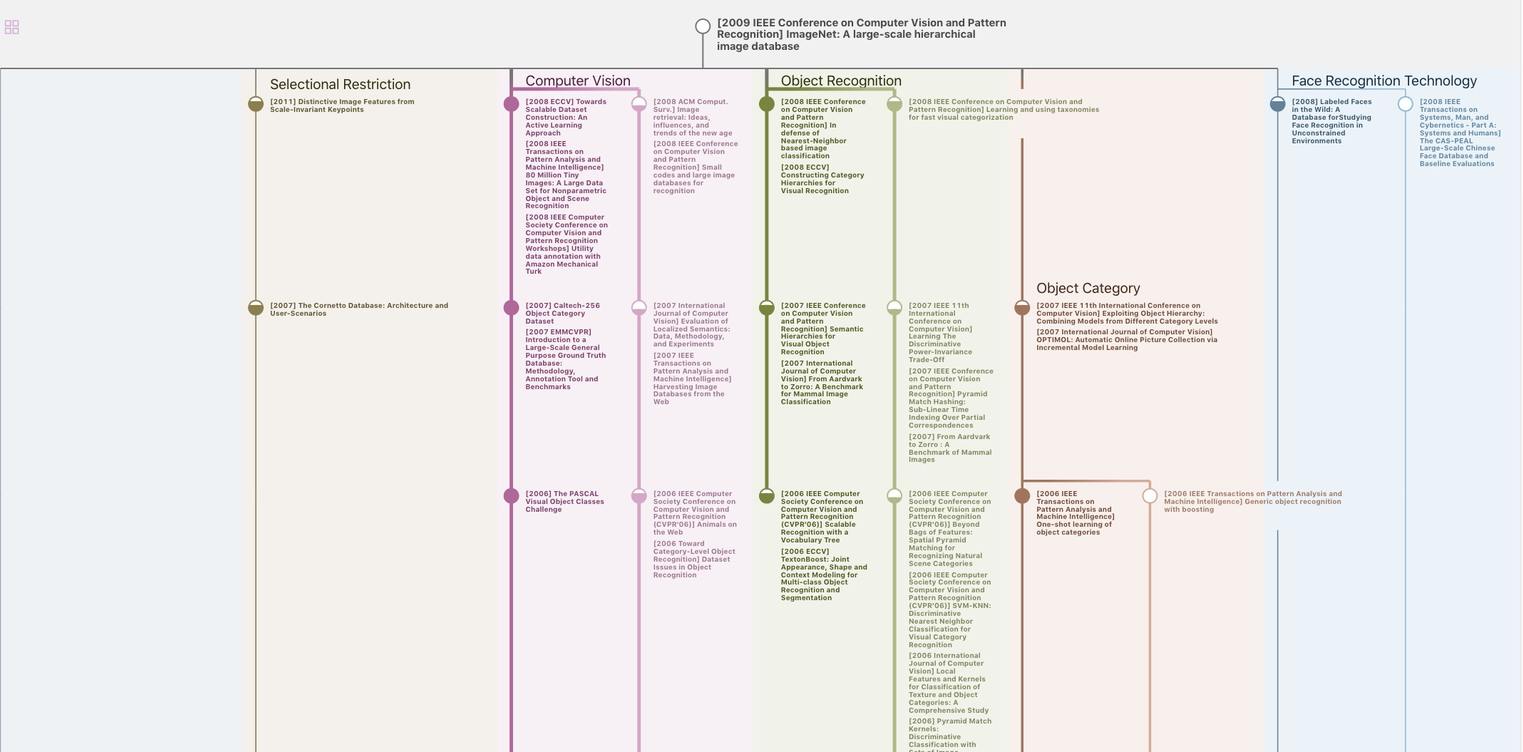
生成溯源树,研究论文发展脉络
Chat Paper
正在生成论文摘要