Deep Learning Based Over-the-Air Channel Estimation Using a ZYNQ SDR Platform
IEEE ACCESS(2022)
摘要
Deep learning based channel estimation techniques have recently found an overwhelming interest owing to data-driven learning-based adaptability compared to conventional estimation techniques which rely on model-based approach. This paper exploits the availability of low cost software defined radio (SDR) devices to implement and test over-the-air (OTA) deep learning driven channel estimation solutions in realistic settings for 5G and beyond wireless communications. We have designed and implemented a ZYNQ SDR-based deep learning driven channel estimation platform which utilises real-world 5G new radio (NR) signals to develop and test the performance of a deep learning solution for wireless channel estimators. To this end, we have considered the time-frequency response of an OTA communication channel for single-input-single-output (SISO) and single-input-multiple-output (SIMO) networks under various line-of-sight and non-line-of-sight scenarios as a two-dimensional image. We have utilised convolutional deep learning technique for channel estimation. The main purpose is to use OTA samples obtained via the SDR platform to determine the unknown values of the channel response using known values at the pilot locations and evaluate performance compared to conventional technique. Our results show that the performance of the deep learning channel estimator using OTA data is as good as that of conventional methods with the flexibility to adapt based on data analytics of the time-varying nature of the channel.
更多查看译文
关键词
Channel estimation, Symbols, OFDM, Wireless communication, Deep learning, Convolutional neural networks, 5G mobile communication, Channel estimation, single-input-single-output, single-input-multiple-output, convolutional neural networks, deep learning, OTA transmission, Zynq FPGA
AI 理解论文
溯源树
样例
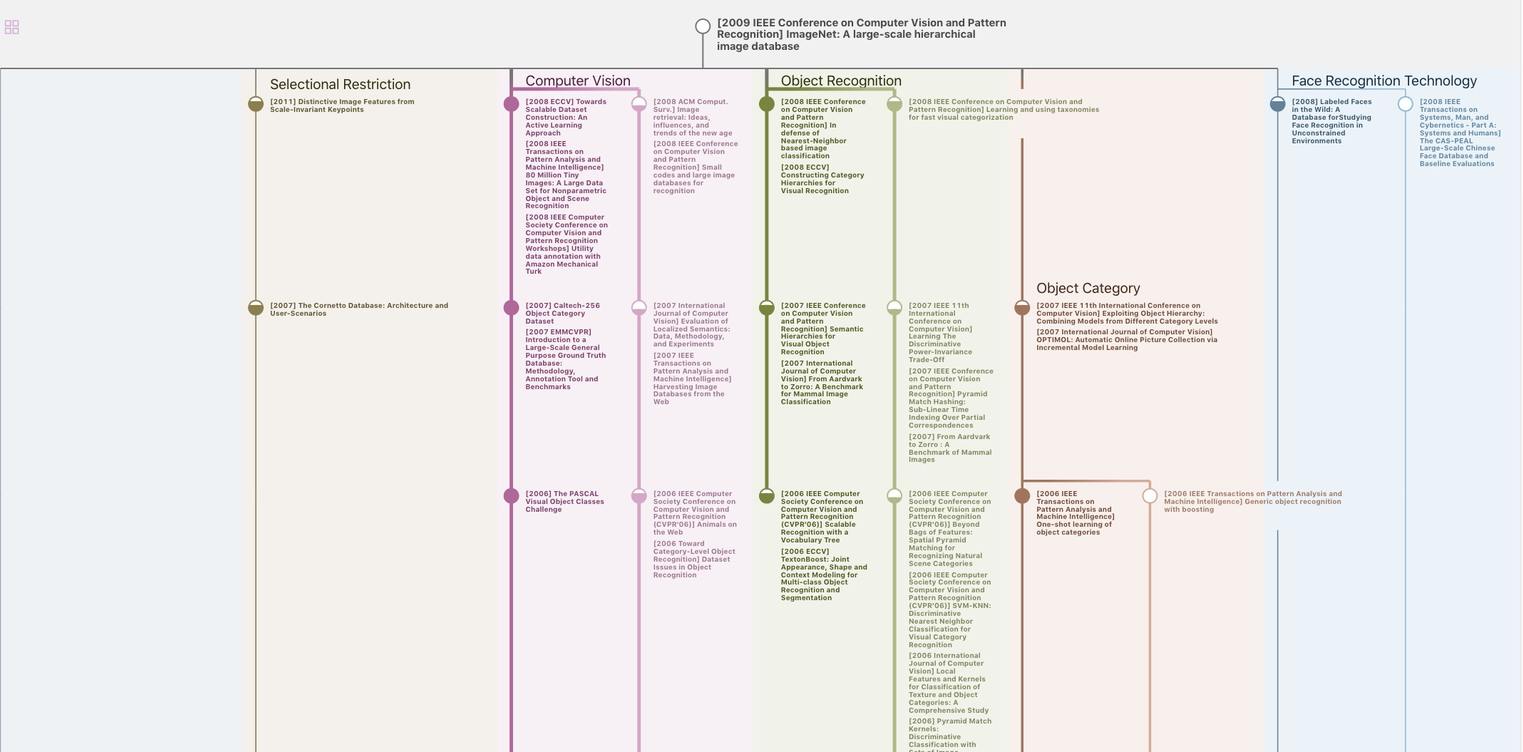
生成溯源树,研究论文发展脉络
Chat Paper
正在生成论文摘要