A Swapping Target Q-Value Technique for Data Augmentation in Offline Reinforcement Learning
IEEE ACCESS(2022)
摘要
Offline reinforcement learning (RL) is applied to fixed datasets of logged interactions pertaining to actual applications in healthcare, autonomous vehicles, and robotics. In limited and fixed dataset settings, data augmentation can be beneficial in developing better policies. Several online RL methods for data augmentation have recently been utilized to enhance sampling efficiency and generalization. Here, a novel, simple data-augmentation technique referred to as Swapping Target Q-Value (SQV) is introduced to enhance offline RL algorithms and enable robust pixel-based learning without auxiliary loss. Our method matches the current Q-value of a transformed image to the target Q-value of the next original image, whereby the current Q-value of the original image is matched to the target Q-value of the next transformed image. The proposed method considers similar states as the same and different states as more distinct. Furthermore, the approach ties unseen data (lacking in the dataset) to similar states in the seen data. After training, these effects were observed to increase the performance of the offline RL algorithm. The method was tested on 23 games in the Atari 2600 game domain. As a result, the performance of our method improved in 18 out of 23 games, with an average performance improvement of 144% compared with batch-constrained deep Q-learning (BCQ), which is the latest offline RL method. The implementation can be found at https://github.com/hotaekjoo/SQV.
更多查看译文
关键词
Behavioral sciences, Games, Artificial intelligence, Training, Q-learning, Medical services, Licenses, Offline reinforcement learning, data augmentation, generalization, Atari games
AI 理解论文
溯源树
样例
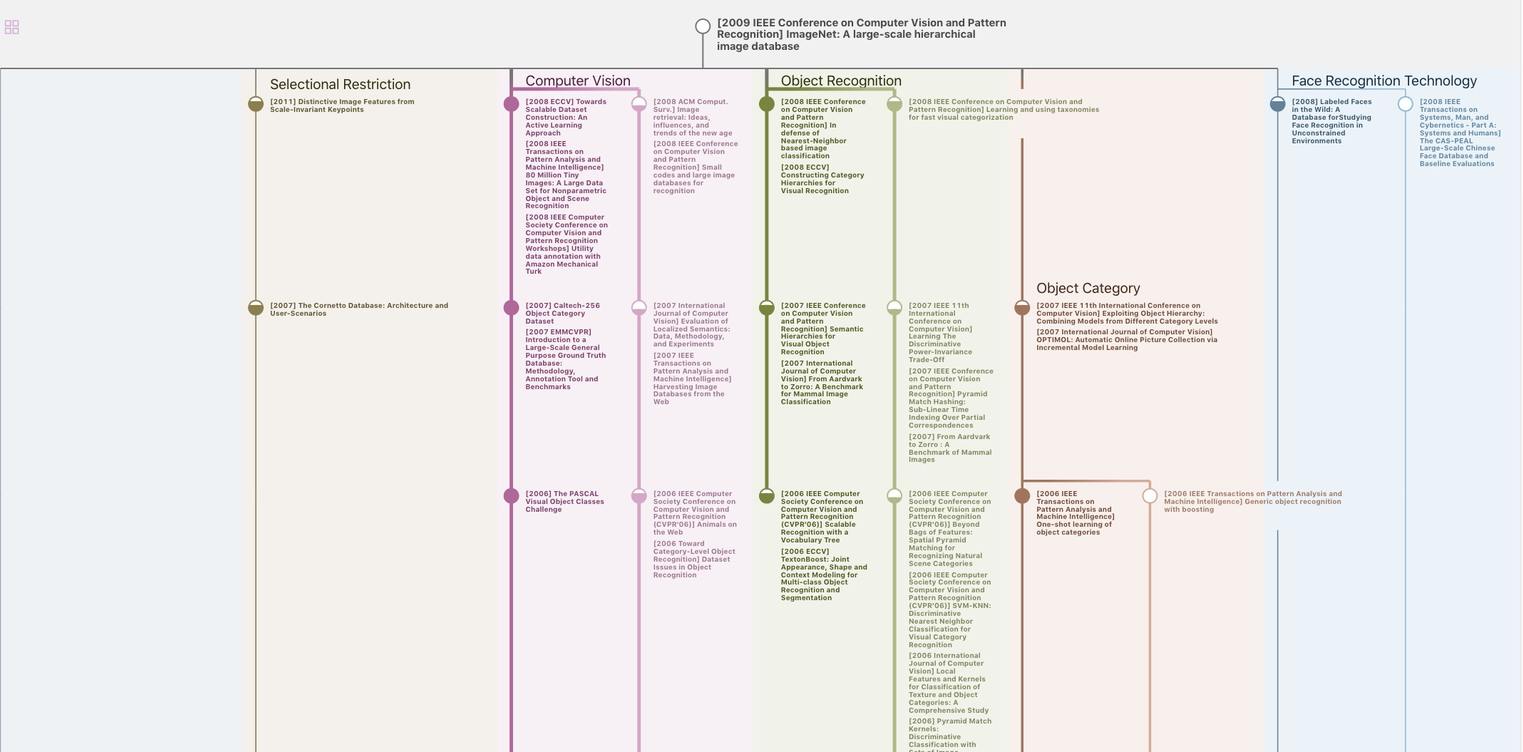
生成溯源树,研究论文发展脉络
Chat Paper
正在生成论文摘要