AdaSL: An Unsupervised Domain Adaptation framework for Arabic multi-dialectal Sequence Labeling
Information Processing & Management(2022)
摘要
Dialectal Arabic (DA) refers to varieties of everyday spoken languages in the Arab world. These dialects differ according to the country and region of the speaker, and their textual content is constantly growing with the rise of social media networks and web blogs. Although research on Natural Language Processing (NLP) on standard Arabic, namely Modern Standard Arabic (MSA), has witnessed remarkable progress, research efforts on DA are rather limited. This is due to numerous challenges, such as the scarcity of labeled data as well as the nature and structure of DA. While some recent works have reached decent results on several DA sentence classification tasks, other complex tasks, such as sequence labeling, still suffer from weak performances when it comes to DA varieties with either a limited amount of labeled data or unlabeled data only. Besides, it has been shown that zero-shot transfer learning from models trained on MSA does not perform well on DA. In this paper, we introduce AdaSL, a new unsupervised domain adaptation framework for Arabic multi-dialectal sequence labeling, leveraging unlabeled DA data, labeled MSA data, and existing multilingual and Arabic Pre-trained Language Models (PLMs). The proposed framework relies on four key components: (1) domain adaptive fine-tuning of multilingual/MSA language models on unlabeled DA data, (2) sub-word embedding pooling, (3) iterative self-training on unlabeled DA data, and (4) iterative DA and MSA distribution alignment. We evaluate our framework on multi-dialectal Named Entity Recognition (NER) and Part-of-Speech (POS) tagging tasks.
更多查看译文
关键词
Dialectal Arabic,Arabic natural language processing,Domain adaptation,Multi-dialectal sequence labeling,Named entity recognition,Part-of-speech tagging,Zero-shot transfer learning
AI 理解论文
溯源树
样例
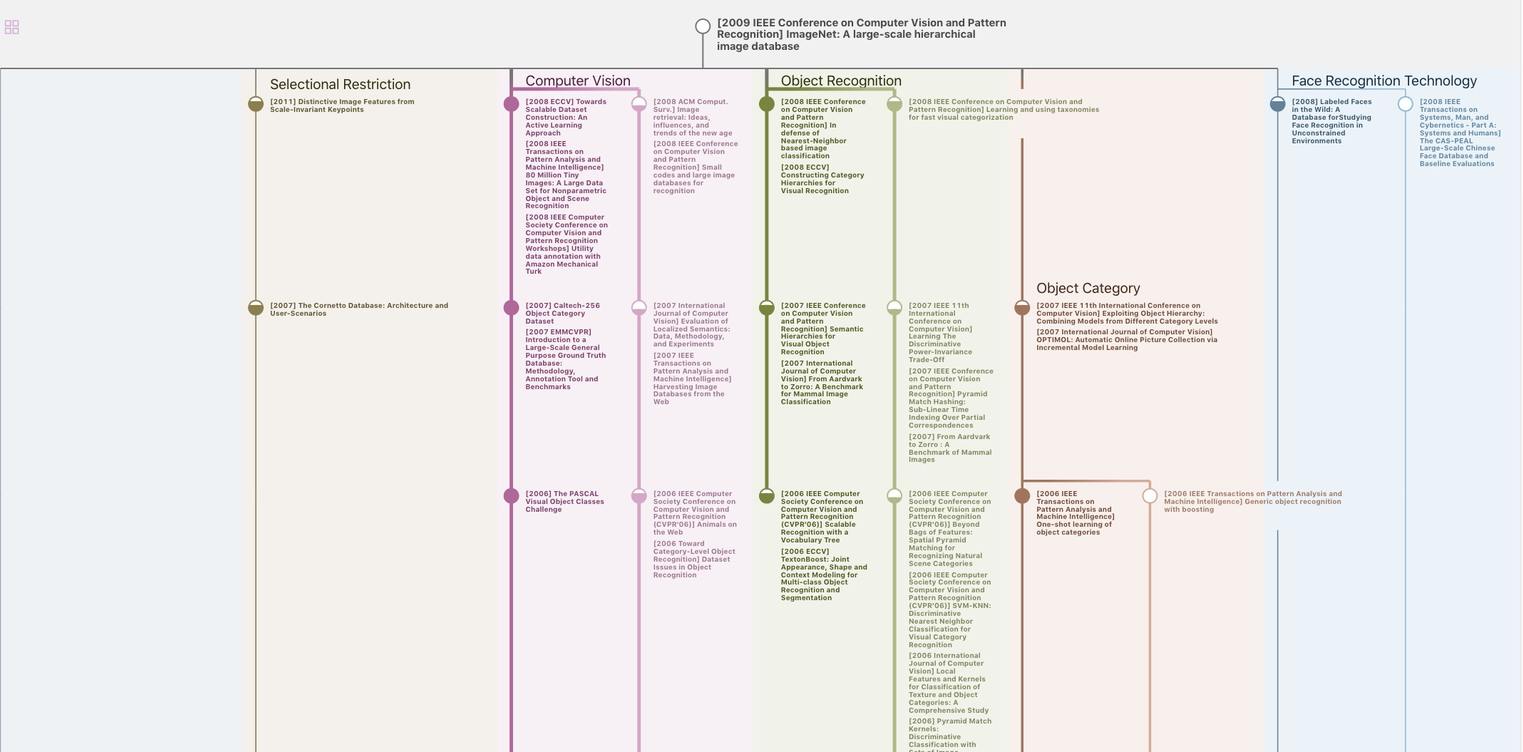
生成溯源树,研究论文发展脉络
Chat Paper
正在生成论文摘要