Improved stereo matching framework with embedded multilevel attention
JOURNAL OF ELECTRONIC IMAGING(2022)
摘要
The recent advent of deep convolutional neural networks (CNNs) in stereo matching has led to significant improvements. However, current CNN methods still face challenges in incorporating hierarchical context information with global dependencies and lacking the discriminative ability of feature representation to resolve matching ambiguities in ill-conditioned regions. To address the aforementioned problems, we propose an improved stereo matching framework that joins a stereo backbone network and an embedded independent multilevel attention subnetwork in an end-to-end trainable pipeline. The stereo backbone network applies a residual atrous spatial pyramid pooling integrated with channelwise attention to capture richer multiscale contextual information and selectively enhance discriminative features. This is followed by unary feature concatenation to construct cost volume for disparity prediction. To further improve performance, the embedded multilevel attention subnetwork learns global coherent contextual information to generate three attention streams, which are used to boost the unary feature representations with spatial encoding, enhance the quality of cost volume, and refine the disparity map, respectively. We show that appending the proposed multilevel attention subnetwork to the stereo backbone network produces significant improvements in matching accuracy. The experimental results on Scene Flow and KITTI 2012/2015 demonstrate that our method can achieve competitive performance in stereo matching. (C) 2022 SPIE and IS&T
更多查看译文
关键词
stereo matching, multilevel attention, global coherence, disparity optimization, deep learning
AI 理解论文
溯源树
样例
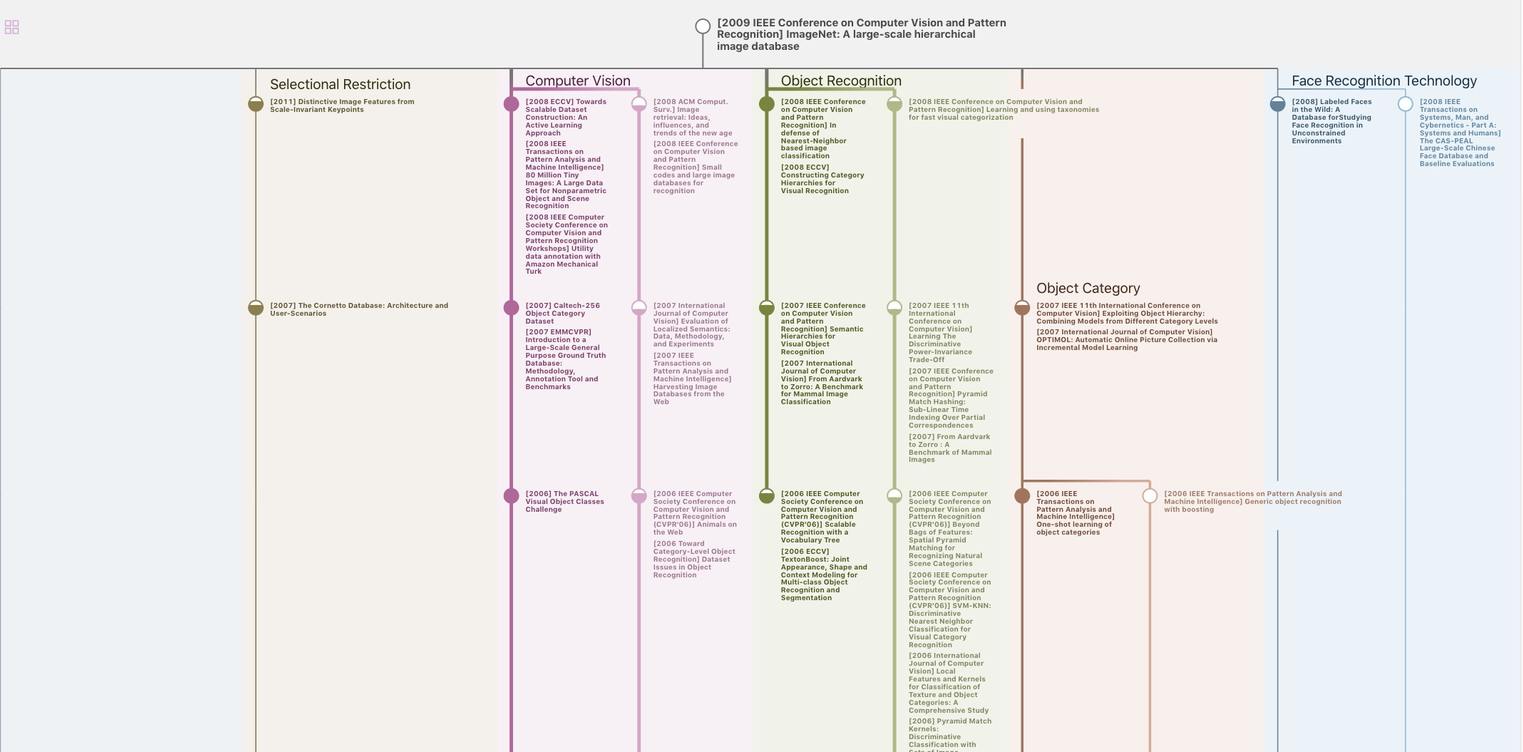
生成溯源树,研究论文发展脉络
Chat Paper
正在生成论文摘要