Radio galaxies classification system using machine learning techniques in the IoT Era
JOURNAL OF EXPERIMENTAL & THEORETICAL ARTIFICIAL INTELLIGENCE(2024)
摘要
Astronomy and astrophysics data sets have been increasing over the last decade as many new telescopes and detectors have been launched. High-redshift radio galaxies are powerful radio sources that are the ideal targets to discuss the evolution of Hi; thus, they are one of the key points to understand the universe evolution and formation. The cloud computing systems are applicable with IoT considering the processing time over training data using ANN. Machine learning is a subfield of AI and it is used by scientists for prediction or classification purposes considering the input data. Machine learning algorithms have become increasingly popular among astronomers and are now used for a wide range of astrophysical calculations and fields. This paper proposes five types of machine learning algorithms, namely back-propagation neural networks (BPNN), decision tree algorithm (DT), gradient boosting classifier algorithm (GBA), radial basis function neural network (RBFNN), and support vector machine (SVM). The machine learning models are implemented to classify and compare the results of high-redshift radio galaxies by their location in the sky in ELAIS-N1, ELAIS-N2, the Lockman hole, VIMOS fields, in order to increase the performance efficiency, accuracy and improve our confidence considering the critical nature of the calculations in redshift galaxies. When 100 instances were considered, back-propagation neural networks achieved an accuracy rate of 70%; however, when 200 instances were considered, radial basis function neural networks achieved an accuracy rate of 88.2%.
更多查看译文
关键词
IoT,machine learning,radio galaxies,astrophysics
AI 理解论文
溯源树
样例
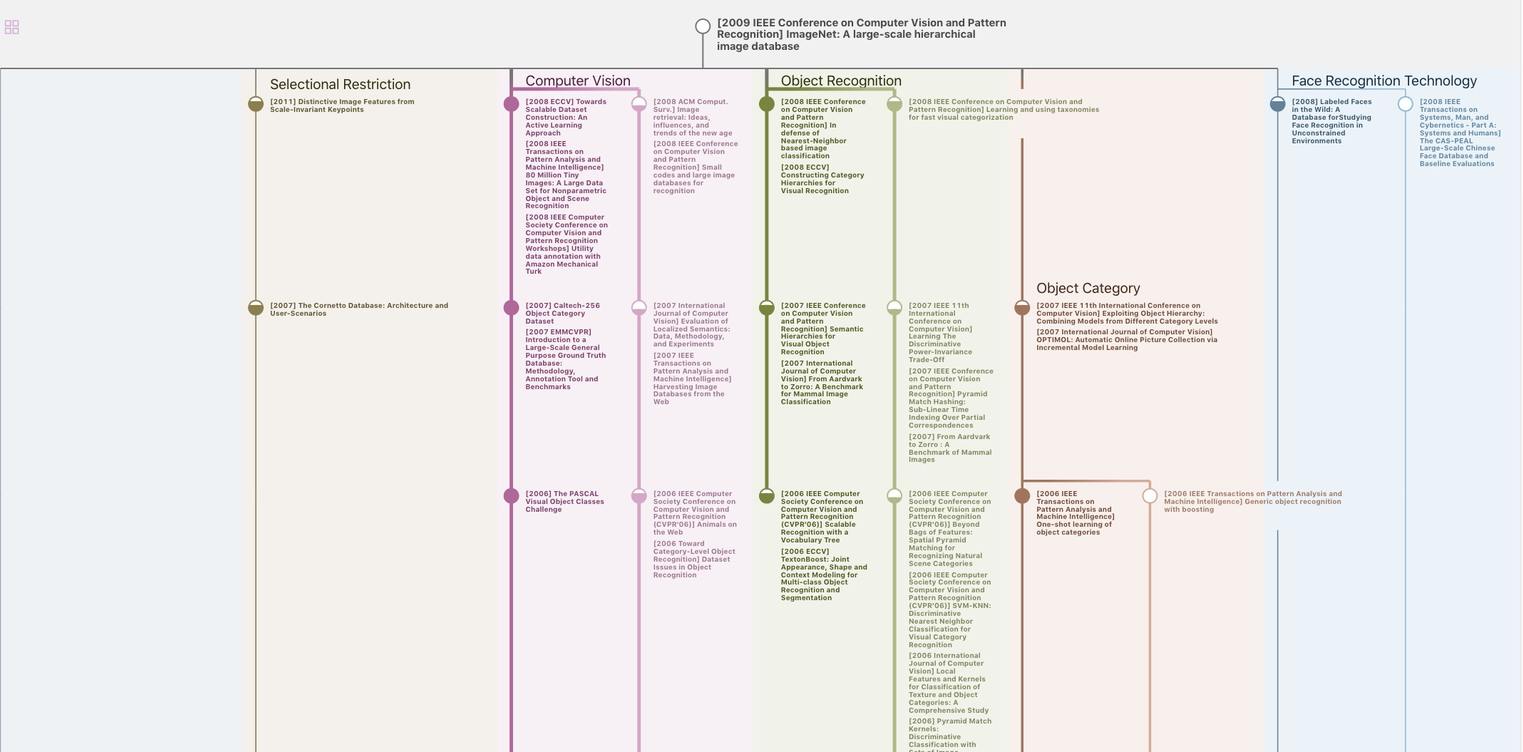
生成溯源树,研究论文发展脉络
Chat Paper
正在生成论文摘要