A dynamic "predict, then optimize" preventive maintenance approach using operational intervention data
EUROPEAN JOURNAL OF OPERATIONAL RESEARCH(2022)
摘要
We investigate whether historical machine failures and maintenance records may be used to derive future machine failure estimates and, in turn, prescribe advancements of scheduled preventive maintenance interventions. We model the problem using a sequential predict, then optimize approach. In our prescriptive optimization model, we use a finite horizon Markov decision process with a variable order Markov chain, in which the chain length varies depending on the time since the last preventive maintenance action was performed. The model therefore captures the dependency of a machine's failures on both recent failures as well as preventive maintenance actions, via our prediction model. We validate our model using an original equipment manufacturer data set and obtain policies that prescribe when to deviate from the planned periodic maintenance schedule. To improve our predictions for machine failure behavior with limited to no past data, we pool our data set over different machine classes by means of a Poisson generalized linear model. We find that our policies can supplement and improve on those currently applied by 5%, on average. (C) 2022 The Author(s). Published by Elsevier B.V.
更多查看译文
关键词
Maintenance, Data-driven decision making, Markov decision process, Data pooling
AI 理解论文
溯源树
样例
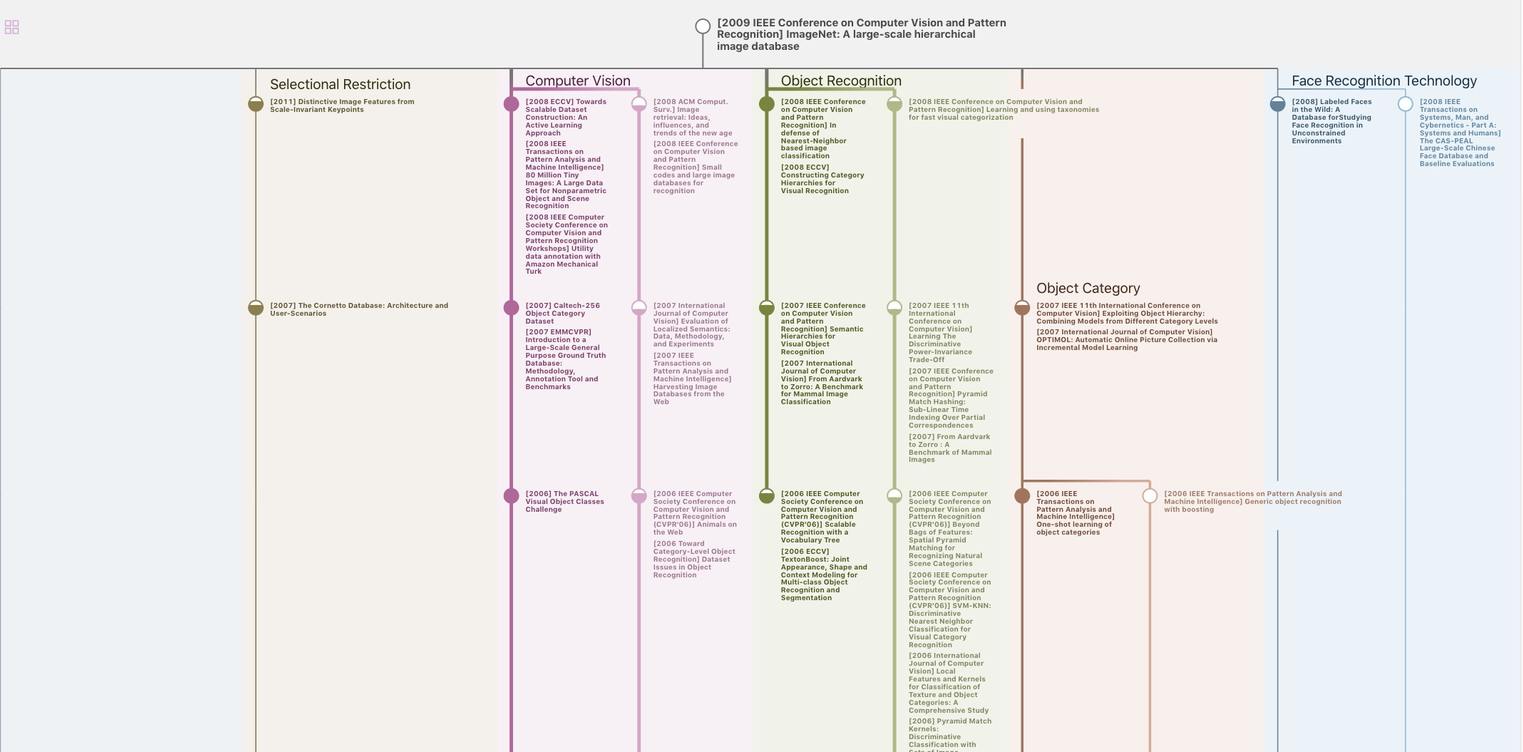
生成溯源树,研究论文发展脉络
Chat Paper
正在生成论文摘要