Efficient and Lightweight Framework for Real-Time Ore Image Segmentation Based on Deep Learning
MINERALS(2022)
摘要
Image segmentation approaches have been utilized to determine the particle size distribution of crushed ores in the past decades. It is not possible to deploy large and high-powered computing equipment due to the complex working environment, so existing algorithms are difficult to apply in practical engineering. This article presents a novel efficient and lightweight framework for ore image segmentation to discern full and independent ores. First, a lightweight backbone is introduced for feature extraction while reducing computational complexity. Then, we propose a compact pyramid network to process the data obtained from the backbone to reduce unnecessary semantic information and computation. Finally, an optimized detection head is proposed to obtain the feature to maintain accuracy. Extensive experimental results demonstrate the effectiveness of our method, which achieves 40 frames per second on our new ore image dataset with a very small model size . Meanwhile, our method maintains a high level of accuracy-67.68% in AP(50)(box) and 46.73% in AP(20)(mask)-compared with state-of-the-art approaches.
更多查看译文
关键词
real-time, deep learning, ore images, instance segmentation
AI 理解论文
溯源树
样例
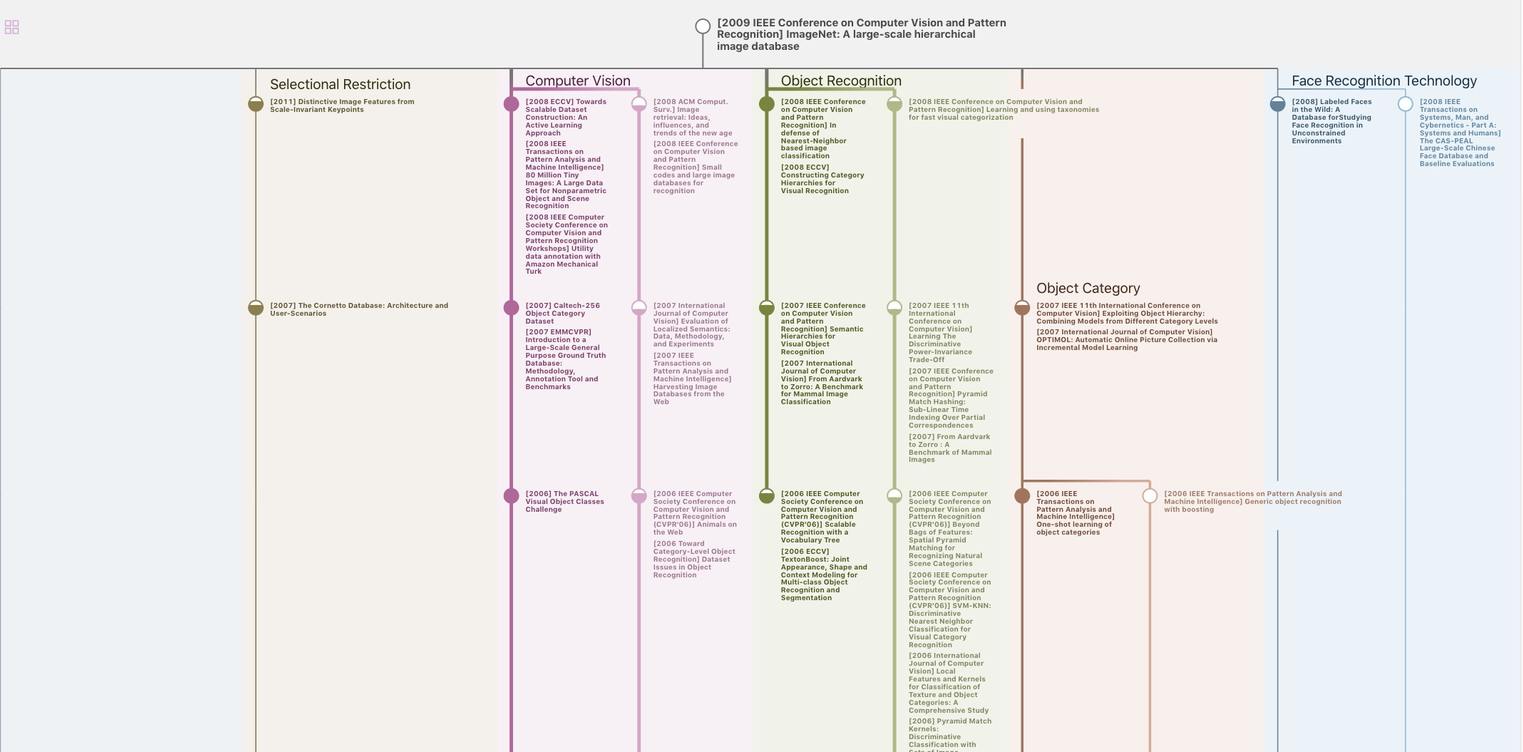
生成溯源树,研究论文发展脉络
Chat Paper
正在生成论文摘要