A data-driven prediction model for aircraft taxi time by considering time series about gate and real-time factors
TRANSPORTMETRICA A-TRANSPORT SCIENCE(2023)
摘要
The prediction of taxi time plays a primary role. To improve the accuracy and portability of prediction, the Informer-RFR (Informer-Random Forest Regression) model was proposed. First, each gate's average taxi time series is clustered, and then an Informer model is trained for each cluster. Second, the RFR model was used to predict the taxi time of flight with the result of Informer and real-time factors. The proposed model can be directly trained by the historical database of a new airport, which can reduce the data processing time. Finally, the model was trained and verified using the data for Beijing Capital International Airport, China (PEK). The results show that the gates of PEK can be divided into four clusters, and the time series of each cluster differ in peak value and changing trend. Our model's accuracy of predicting within +/- 5 min is 96.62%, and the mean absolute error is 147.59 s.
更多查看译文
关键词
Aircraft taxiing time, long-term and short-term, Informer, Random Forest Regression
AI 理解论文
溯源树
样例
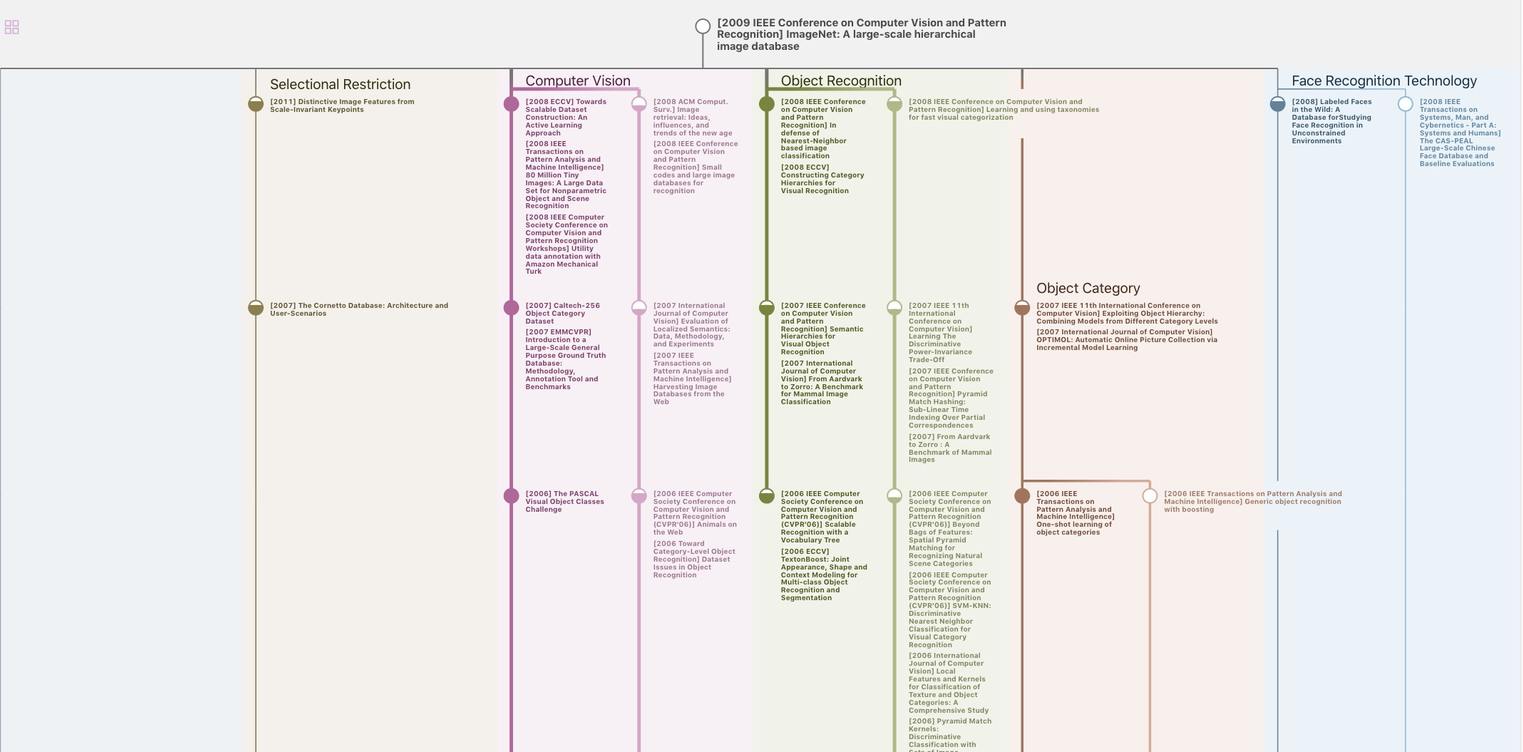
生成溯源树,研究论文发展脉络
Chat Paper
正在生成论文摘要