Recognition of multi-cognitive tasks from EEG signals using EMD methods
NEURAL COMPUTING & APPLICATIONS(2022)
摘要
Mental task classification (MTC), based on the electroencephalography (EEG) signals is a demanding brain–computer interface (BCI). It is independent of all types of muscular activity. MTC-based BCI systems are capable to identify cognitive activity of human. The success of BCI system depends upon the efficient feature representation from raw EEG signals for classification of mental activities. This paper mainly presents on a novel feature representation (formation of most informative features) of the EEG signal for the both, binary as well as multi MTC, using a combination of some statistical, uncertainty and memory- based coefficient. In this work, the feature formation is carried out in the two stages. In the first stage, the signal is split into different oscillatory functions with the help of three well-known empirical mode decomposition (EMD) algorithms, and a new set of eight parameters (features) are calculated from the oscillatory function in the second stage of feature vector construction. Support vector machine (SVM) is used to classify the feature vectors obtained corresponding to the different mental tasks. This study consists the problem formulation of two variants of MTC; two-class and multi-class MTC. The suggested scheme outperforms the existing work for the both types of mental tasks classification.
更多查看译文
关键词
Brain–computer interface,Mental tasks classification,Feature extraction,EMD Algorithms,Parameters
AI 理解论文
溯源树
样例
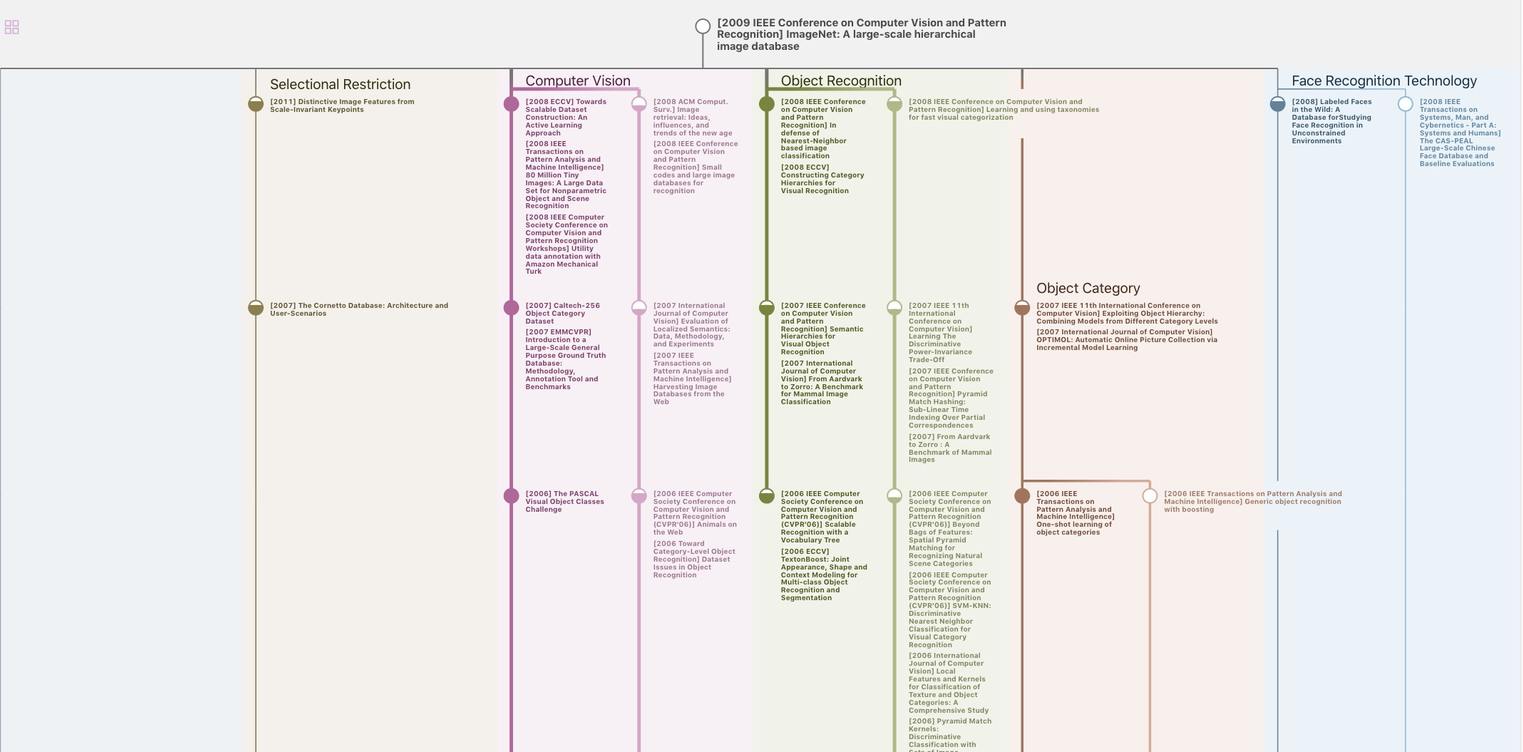
生成溯源树,研究论文发展脉络
Chat Paper
正在生成论文摘要