HFMOEA: a hybrid framework for multi-objective feature selection
JOURNAL OF COMPUTATIONAL DESIGN AND ENGINEERING(2022)
摘要
In this data-driven era, where a large number of attributes are often publicly available, redundancy becomes a major problem, which leads to large storage and computational resource requirement. Feature selection is a method for reducing the dimensionality of the data by removing such redundant or misleading attributes. This leads to a selection of optimal feature subsets that can be used for further computation like the classification of data. Learning algorithms, when fitted on such optimal subsets of reduced dimensions, perform more efficiently and storing data also becomes easier. However, there exists a trade-off between the number of features selected and the accuracy obtained and the requirement for different tasks may vary. Thus, in this paper, a hybrid filter multi-objective evolutionary algorithm (HFMOEA) has been proposed based on the nondominated sorting genetic algorithm (NSGA-II) coupled with filter-based feature ranking methods for population initialization to obtain an optimal trade-off solution set to the problem. The two competing objectives for the algorithm are the minimization of the number of selected features and the maximization of the classification accuracy. The filter ranking methods used for population initialization help in faster convergence of the NSGA-II algorithm to the PF. The proposed HFMOEA method has been evaluated on 18 UCI datasets and 2 deep feature sets (features extracted from image datasets using deep learning models) to justify the viability of the approach with respect to the state-of-the-art. The relevant codes of the proposed approach are available at https://github.corn/Rohit-Kundu/HFMOEA.
更多查看译文
关键词
hybrid optimization, multi-objective optimization problem (MOOP), feature selection, filter ranking
AI 理解论文
溯源树
样例
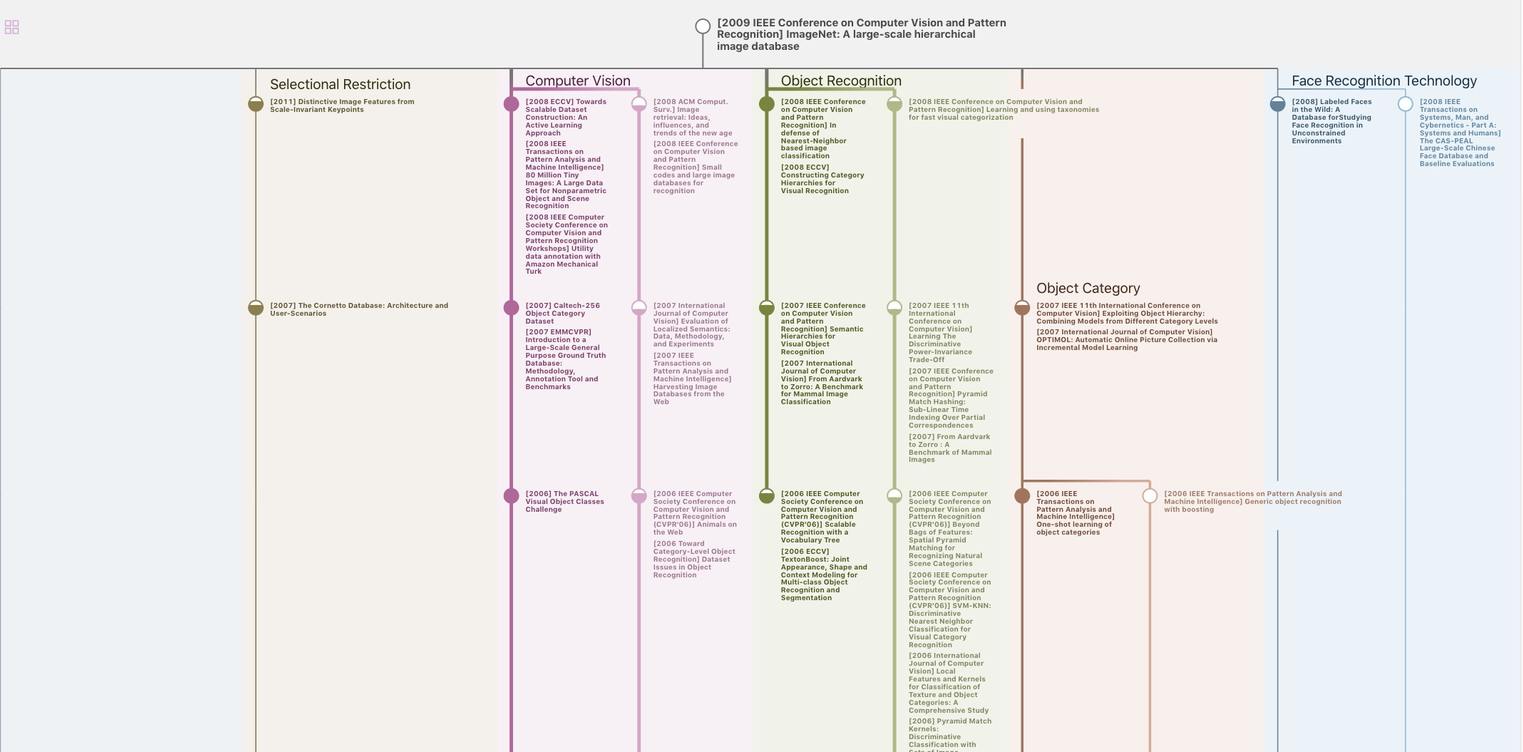
生成溯源树,研究论文发展脉络
Chat Paper
正在生成论文摘要