Weighted Schatten p-norm minimization with logarithmic constraint for subspace clustering
Signal Processing(2022)
摘要
Rank minimization-based subspace clustering methods have been widely developed in the past decades. Although some smooth surrogates, such as the nuclear norm and Schatten-p norm mitigate the NP-hard issue to some extend, these existing methods may yield unsatisfactory results, due to the singular values of the coefficient matrix not being further suppressed. To tackle this, in this paper, we propose a novel non-convex low-rank approximation based on weighted Schatten-p norm jointed logarithmic constraint, which can suppress the small and large singular values flexibly with a tighter relaxation. Specifically, we firstly proposed a low-rank approximation termed SLog by utilizing the logarithmic to tighten the Schatten-p norm, which can shrink the large singular values in a similar trend of the real rank minimization. Furthermore, to suppress the small singular values simultaneously, usually considered noise, we propose a weighted Schatten p-norm minimization named WSLog based on SLog by introducing the weight w, which behaves more robust to the sparse noise, especially w<1. Compared with recent-proposed methods, extensive experiments in subspace clustering on real datasets demonstrate the effective performance of our methods.
更多查看译文
关键词
Subspace clustering,Low-rank representation,Schatten-p norm,Logarithmic constraint
AI 理解论文
溯源树
样例
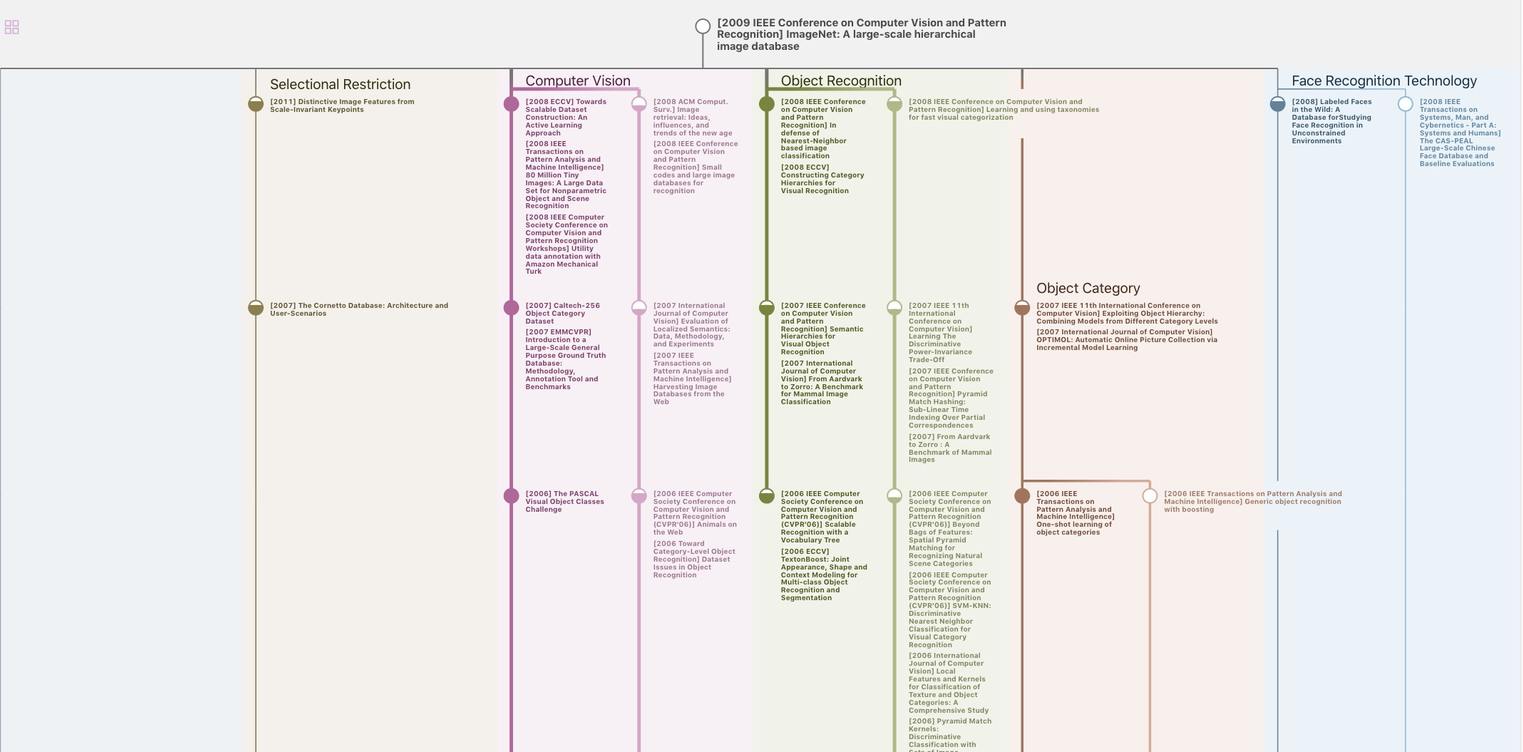
生成溯源树,研究论文发展脉络
Chat Paper
正在生成论文摘要