COVID-19 Tweets Classification Based on a Hybrid Word Embedding Method
BIG DATA AND COGNITIVE COMPUTING(2022)
摘要
In March 2020, the World Health Organisation declared that COVID-19 was a new pandemic. This deadly virus spread and affected many countries in the world. During the outbreak, social media platforms such as Twitter contributed valuable and massive amounts of data to better assess health-related decision making. Therefore, we propose that users' sentiments could be analysed with the application of effective supervised machine learning approaches to predict disease prevalence and provide early warnings. The collected tweets were prepared for preprocessing and categorised into: negative, positive, and neutral. In the second phase, different features were extracted from the posts by applying several widely used techniques, such as TF-IDF, Word2Vec, Glove, and FastText to capture features' datasets. The novelty of this study is based on hybrid features extraction, where we combined syntactic features (TF-IDF) with semantic features (FastText and Glove) to represent posts accurately, which helps in improving the classification process. Experimental results show that FastText combined with TF-IDF performed better with SVM than the other models. SVM outperformed the other models by 88.72%, as well as for XGBoost, with an 85.29% accuracy score. This study shows that the hybrid methods proved their capability of extracting features from the tweets and increasing the performance of classification.
更多查看译文
关键词
COVID-19, features extraction, machine learning, sentiment analysis, text classification, Twitter
AI 理解论文
溯源树
样例
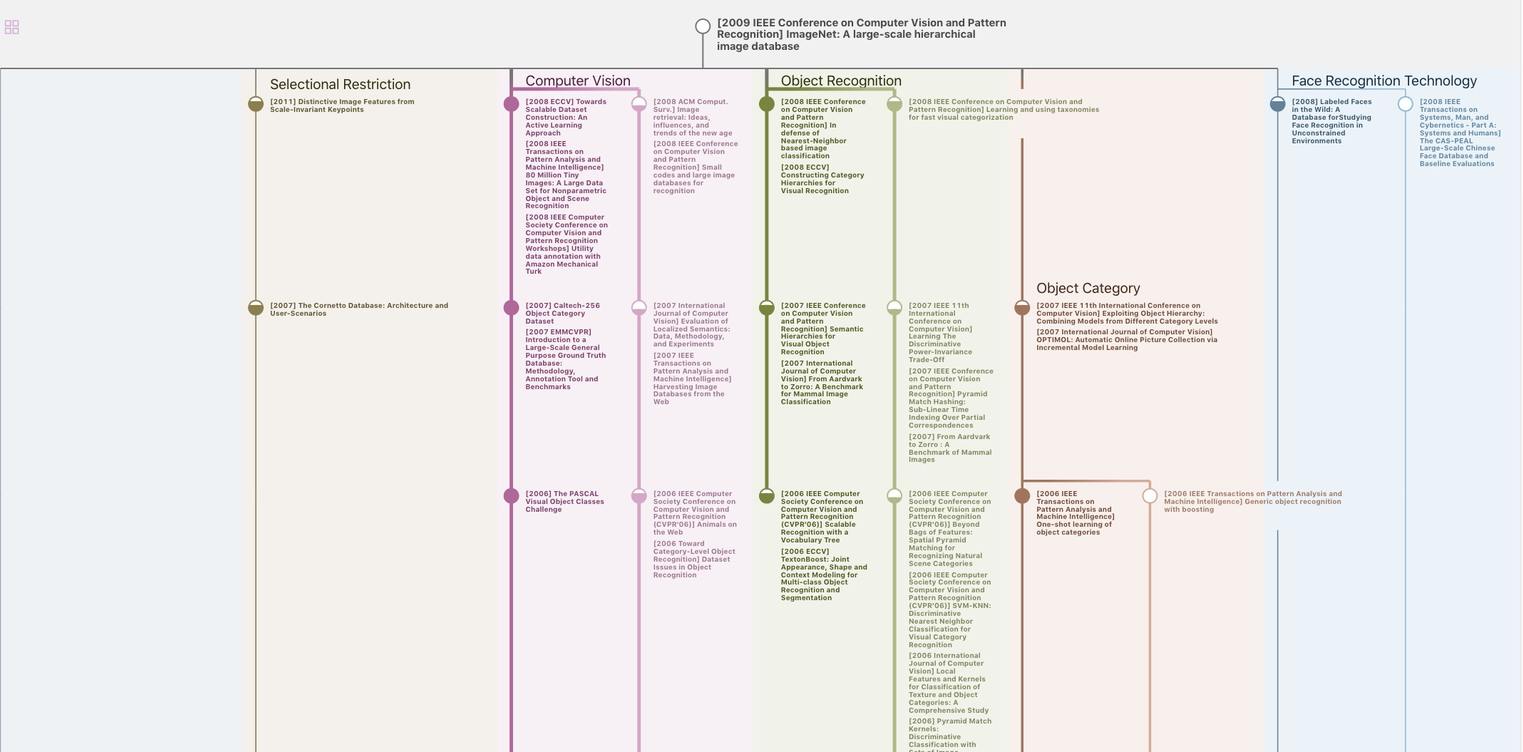
生成溯源树,研究论文发展脉络
Chat Paper
正在生成论文摘要