Cluster-Based Analogue Ensembles for Hindcasting with Multistations
COMPUTATION(2022)
摘要
The Analogue Ensemble (AnEn) method enables the reconstruction of meteorological observations or deterministic predictions for a certain variable and station by using data from the same station or from other nearby stations. However, depending on the dimension and granularity of the historical datasets used for the reconstruction, this method may be computationally very demanding even if parallelization is used. In this work, the classical AnEn method is modified so that analogues are determined using K-means clustering. The proposed combined approach allows the use of several predictors in a dependent or independent way. As a result of the flexibility and adaptability of this new approach, it is necessary to define several parameters and algorithmic options. The effects of the critical parameters and main options were tested on a large dataset from real-world meteorological stations. The results show that adequate monitoring and tuning of the new method allows for a considerable improvement of the computational performance of the reconstruction task while keeping the accuracy of the results. Compared to the classical AnEn method, the proposed variant is at least 15-times faster when processing is serial. Both approaches benefit from parallel processing, with the K-means variant also being always faster than the classic method under that execution regime (albeit its performance advantage diminishes as more CPU threads are used).
更多查看译文
关键词
dcasting, meteorological dataset, analogue ensemble, K-means, time-series
AI 理解论文
溯源树
样例
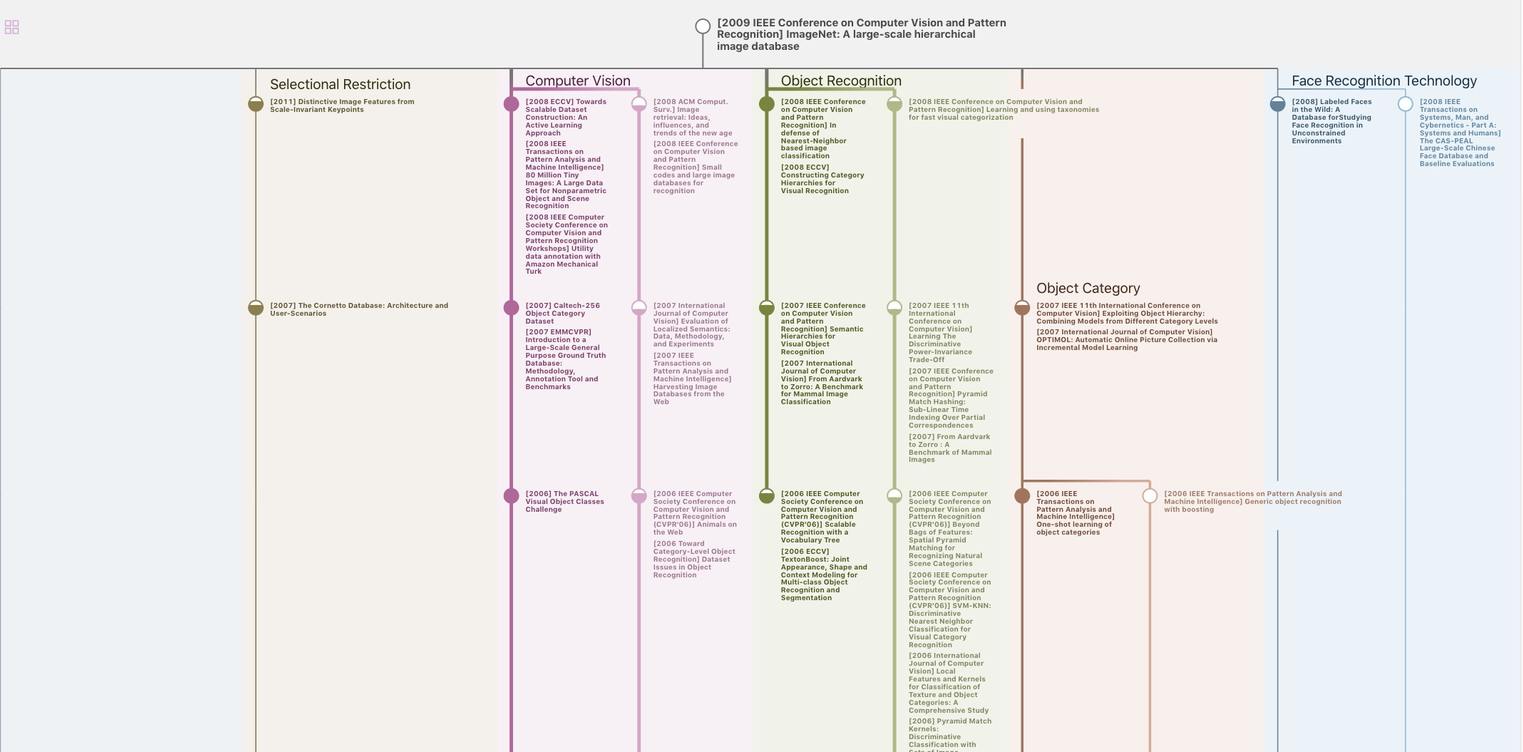
生成溯源树,研究论文发展脉络
Chat Paper
正在生成论文摘要