Light Weighted CNN Model to Detect DDoS Attack over Distributed Scenario
SECURITY AND COMMUNICATION NETWORKS(2022)
摘要
The minimal-degree distributed denial-of-service attack takes advantage of flaws in the adaptive mechanisms of network protocols, which could have a big impact on network service quality. It is very hard to find, has a low attack rate, and comes at a set time. Detection methods that have been used before have problems because they only use one type of detection and are not very good at identifying the object. In the end, a way to detect many sorts of minimal DDoS assaults that use deep hybrid learning is suggested. To construct multi-type limited DDoS threat data sets and mimic diverse sorts DDoS assaults and legitimate traffic in varying situations in the 5G setting, collect congestion at the networking entry and extract flow feature info are considered. From a statistical threshold and feature engineering point of view, these data sets show how many sorts of minimal DDoS assaults are there. This study aims to develop a deep hybrid learning-based multi-type low-rate DDoS attack detection solution for 5G networks which is the novel model that is recently deployed, and a hybrid deep learning algorithm was used to train the algorithm offline, and the algorithm's performance was compared to that of the LSTM-Light GBM and LSTM-RF algorithms. The CNN-RF revealing model was then used to detect minimal DDoS assaults at the gateway, so that multiple attacks could be detected at the same time. It can identify 4 sorts of low-rate DDoS assaults like Slow-Headers, Slow-Body, Slow-Read, and Shrew assaults, in a 120-second window. The false intercept rate is 11.03 percent. This means that 96.22 percent of traffic could be found. Using the strategy suggested can help cut down on the traffic concentration of minimal DDoS attacks at the net ingress. It can also be used in real-world situations.
更多查看译文
关键词
light weighted cnn model,ddos attack
AI 理解论文
溯源树
样例
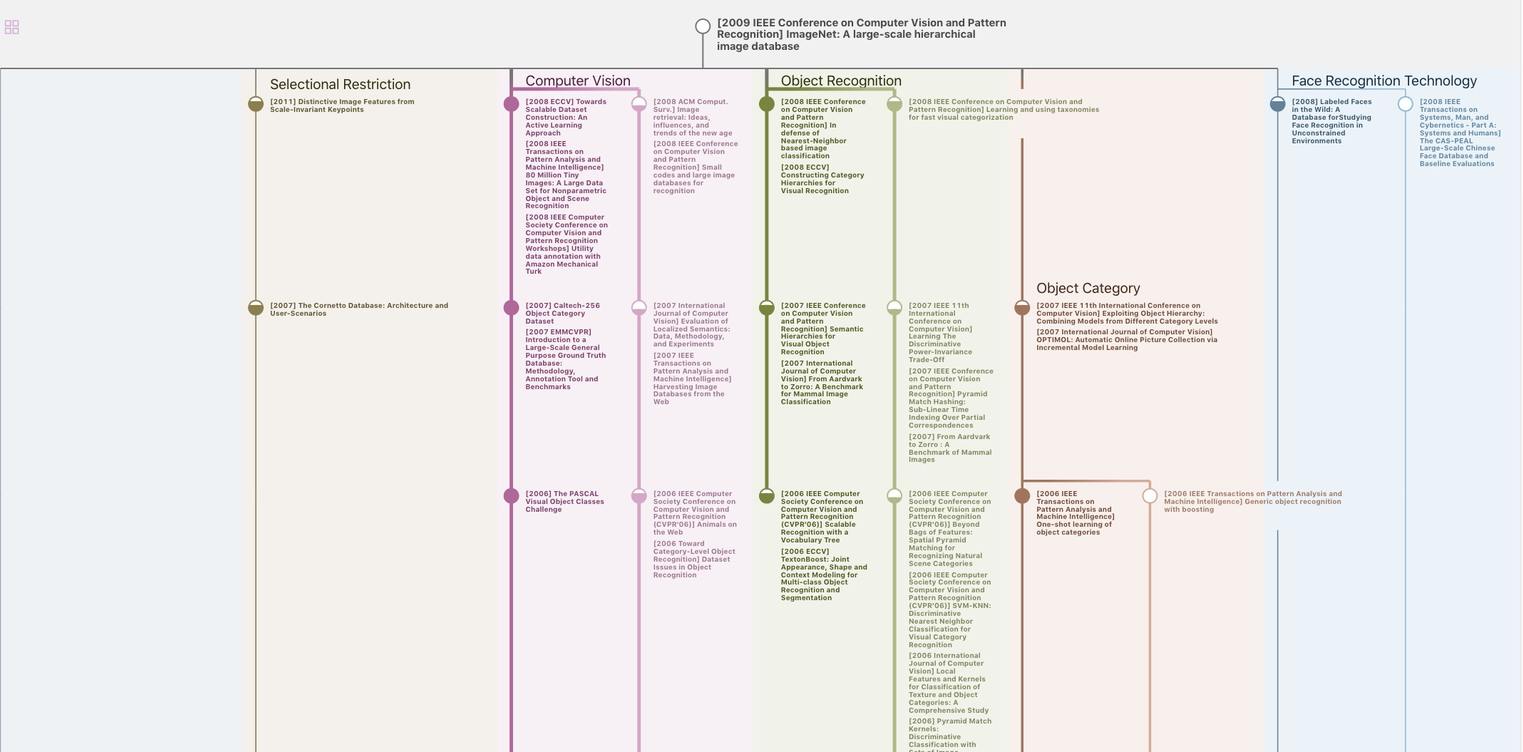
生成溯源树,研究论文发展脉络
Chat Paper
正在生成论文摘要