FELIDS: Federated learning-based intrusion detection system for Internet of
JOURNAL OF PARALLEL AND DISTRIBUTED COMPUTING(2022)
摘要
In this paper, we propose a federated learning-based intrusion detection system, named FELIDS, for securing agricultural-IoT infrastructures. Specifically, the FELIDS system protects data privacy through local learning, where devices benefit from the knowledge of their peers by sharing only updates from their model with an aggregation server that produces an improved detection model. In order to prevent Agricultural IoTs attacks, the FELIDS system employs three deep learning classifiers, namely, deep neural networks, convolutional neural networks, and recurrent neural networks. We study the performance of the proposed IDS on three different sources, including, CSE-CIC-IDS2018, MQTTset, and InSDN. The results demonstrate that the FELIDS system outperforms the classic/centralized versions of machine learning (non-federated learning) in protecting the privacy of IoT devices data and achieves the highest accuracy in detecting attacks.
更多查看译文
关键词
Federated learning,Deep learning,Internet of Things,Security,Privacy
AI 理解论文
溯源树
样例
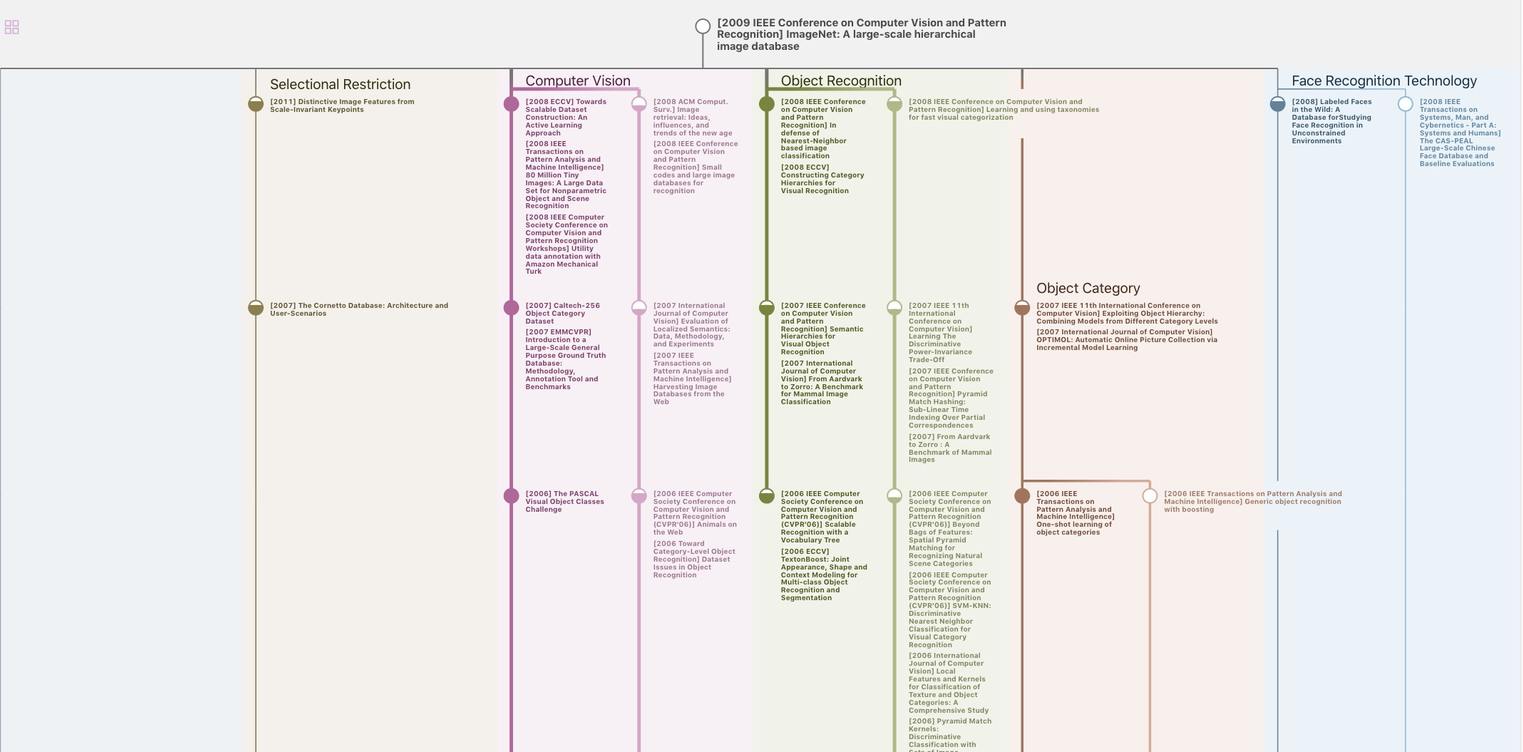
生成溯源树,研究论文发展脉络
Chat Paper
正在生成论文摘要