An Estimated-Travel-Time Data Scraping and Analysis Framework for Time-Dependent Route Planning
DATA(2022)
摘要
Generally, a courier company needs to employ a fleet of vehicles to travel through a number of locations in order to provide efficient parcel delivery services. The route planning of these vehicles can be formulated as a vehicle routing problem (VRP). Most existing VRP algorithms assume that the traveling durations between locations are time invariant; thus, they normally use only a set of estimated travel times (ETTs) to plan the vehicles' routes; however, this is not realistic because the traffic pattern in a city varies over time. One solution to tackle the problem is to use different sets of ETTs for route planning in different time periods, and these data are collectively called the time-dependent estimated travel times (TD-ETTs). This paper focuses on a low-cost and robust solution to effectively scrape, process, clean, and analyze the TD-ETT data from free web-mapping services in order to gain the knowledge of the traffic pattern in a city in different time periods. To achieve the abovementioned goal, our proposed framework contains four phases, namely, (i) Full Data Scraping, (ii) Data Pre-Processing and Analysis, (iii) Fast Data Scraping, and (iv) Data Patching and Maintenance. In our experiment, we used the above framework to obtain the TD-ETT data across 68 locations in Penang, Malaysia, for six months. We then fed the data to a VRP algorithm for evaluation. We found that the performance of our low-cost approach is comparable with that of using the expensive paid data.
更多查看译文
关键词
time-dependent route planning, estimated travel time, data scraping, data preprocessing, data analysis
AI 理解论文
溯源树
样例
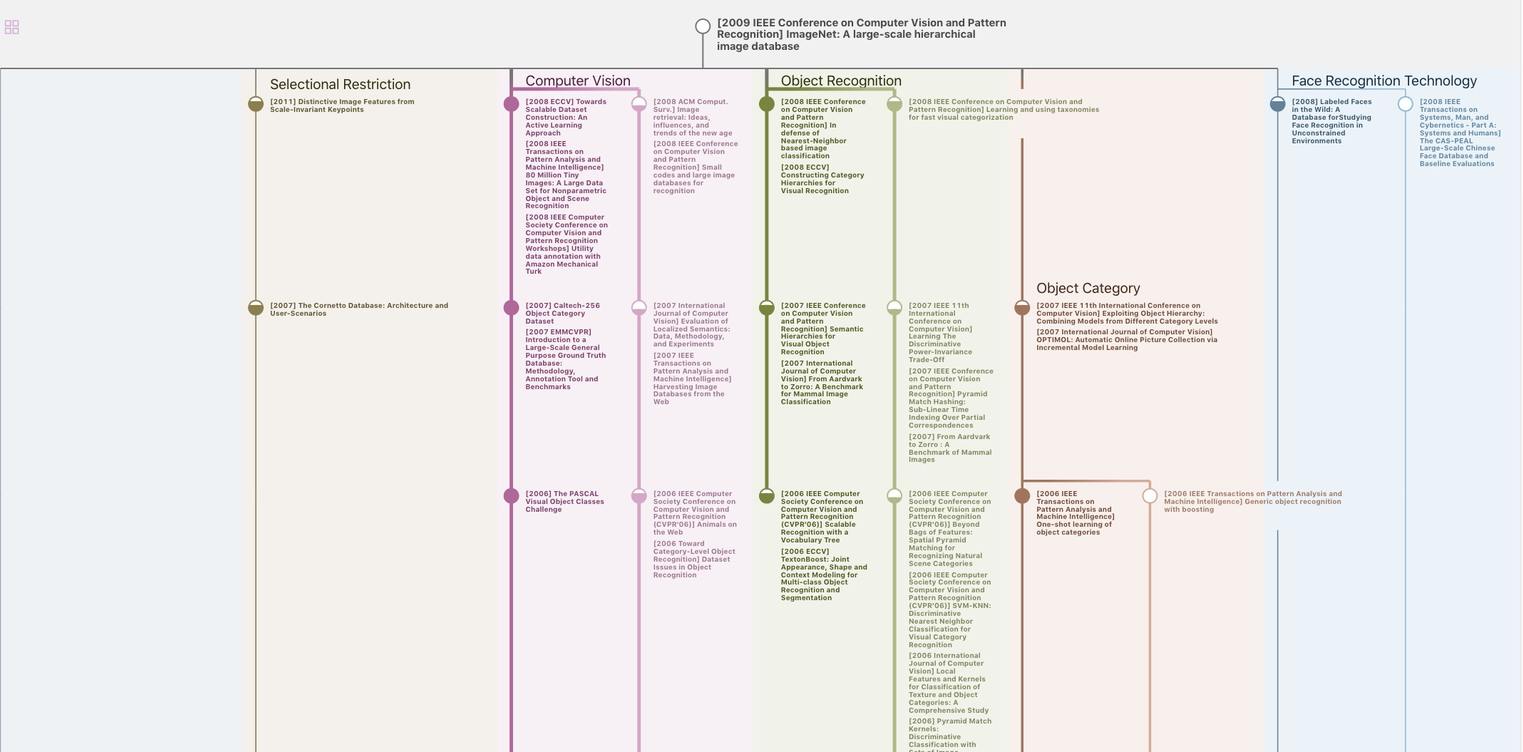
生成溯源树,研究论文发展脉络
Chat Paper
正在生成论文摘要