Imbalanced Data Classification of Pathological Speech Using PCA, SMOTE, and Expectation Maximization
COMMUNICATIONS, SIGNAL PROCESSING, AND SYSTEMS, VOL. 1(2022)
摘要
Imbalance can affect the classification. In this study, a new algorithm to classify the pathology by considering the imbalance between classes has been built. This algorithm used principal component analysis (PCA), synthetic minority oversampling technique (SMOTE), and cluster membership degrees. Both PCA and Expectation-Maximization algorithms are used to give new features combined with the existing features. This proposed method is associated with Support Vector Machine (SVM) and Naive Bayes (NB) for severity classification of UA speech and TORGO speech databases. Another point in this work revealed the importance of articulatory of the TORGO database. The evaluation of this method on the two databases showed the significant results where the increase for TORGO database articulatory features, auditory features, and their combination was respectively 8.03%, 16.68%, and 17.79% compared to SVM performance and 3.04%, 23.67%, and 13.5% compared to the NB performance. The increase for UA speech was 5.13% and 24.63% compared to SVM and NB performance respectively. Additionally, the proposed method has outperformed four well-known imbalanced classification algorithms.
更多查看译文
关键词
Dysarthria, Imbalance, PCA, SMOTE, Expectation-Maximization
AI 理解论文
溯源树
样例
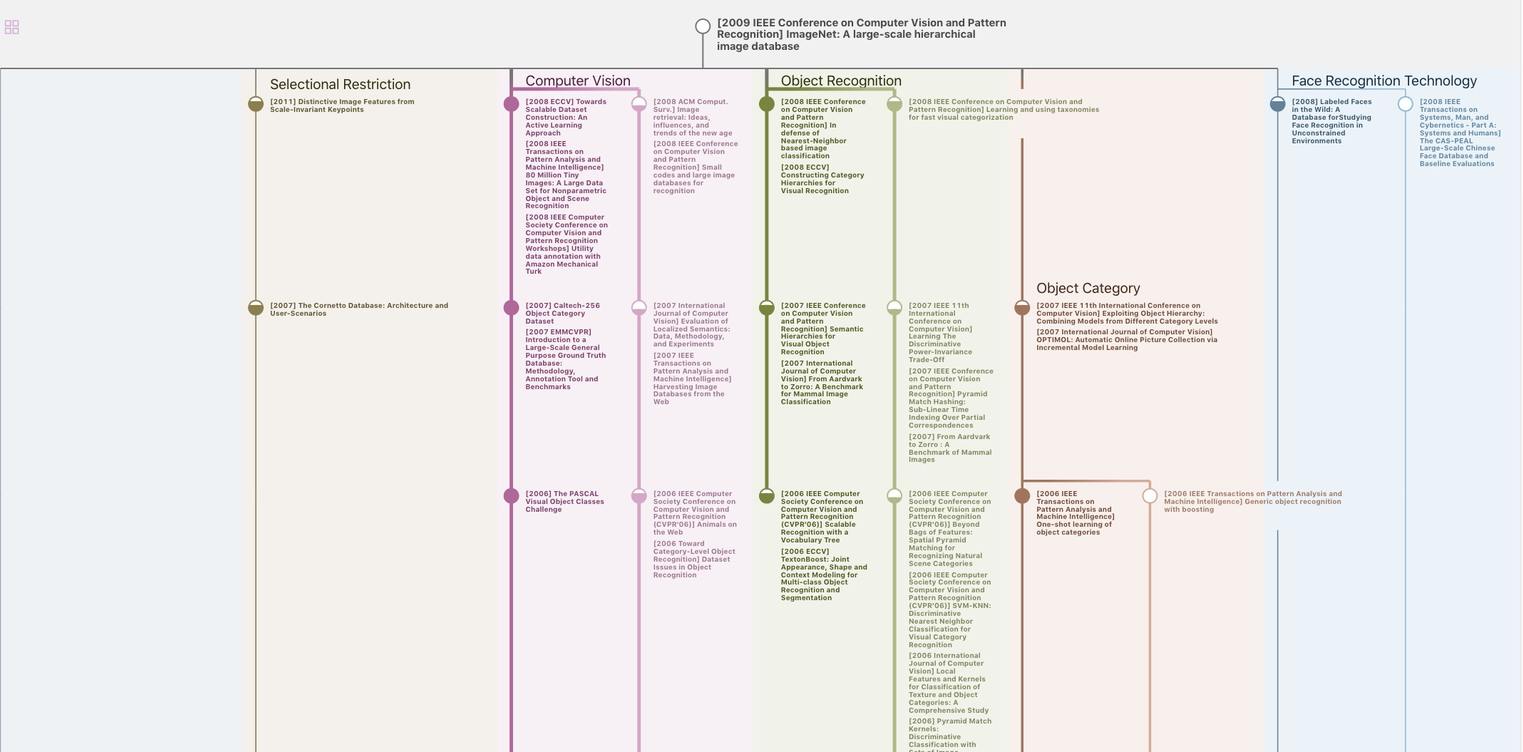
生成溯源树,研究论文发展脉络
Chat Paper
正在生成论文摘要