Vision transformer-based autonomous crack detection on asphalt and concrete surfaces
Automation in Construction(2022)
摘要
Previous research has shown the high accuracy of convolutional neural networks (CNNs) in asphalt and concrete crack detection in controlled conditions. Yet, human-like generalisation remains a significant challenge for industrial applications where the range of conditions varies significantly. Given the intrinsic biases of CNNs, this paper proposes a vision transformer (ViT)-based framework for crack detection on asphalt and concrete surfaces. With transfer learning and the differentiable intersection over union (IoU) loss function, the encoder-decoder network equipped with ViT could achieve an enhanced real-world crack segmentation performance. Compared to the CNN-based models (DeepLabv3+ and U-Net), TransUNet with a CNN-ViT backbone achieved up to ~61% and ~3.8% better mean IoU on the original images of the respective datasets with very small and multi-scale crack semantics. Moreover, ViT assisted the encoder-decoder network to show a robust performance against various noisy signals where the mean Dice score attained by the CNN-based models significantly dropped (<10%).
更多查看译文
关键词
Crack detection,Deep learning,Vision transformer,Convolutional neural network,Human recognition system
AI 理解论文
溯源树
样例
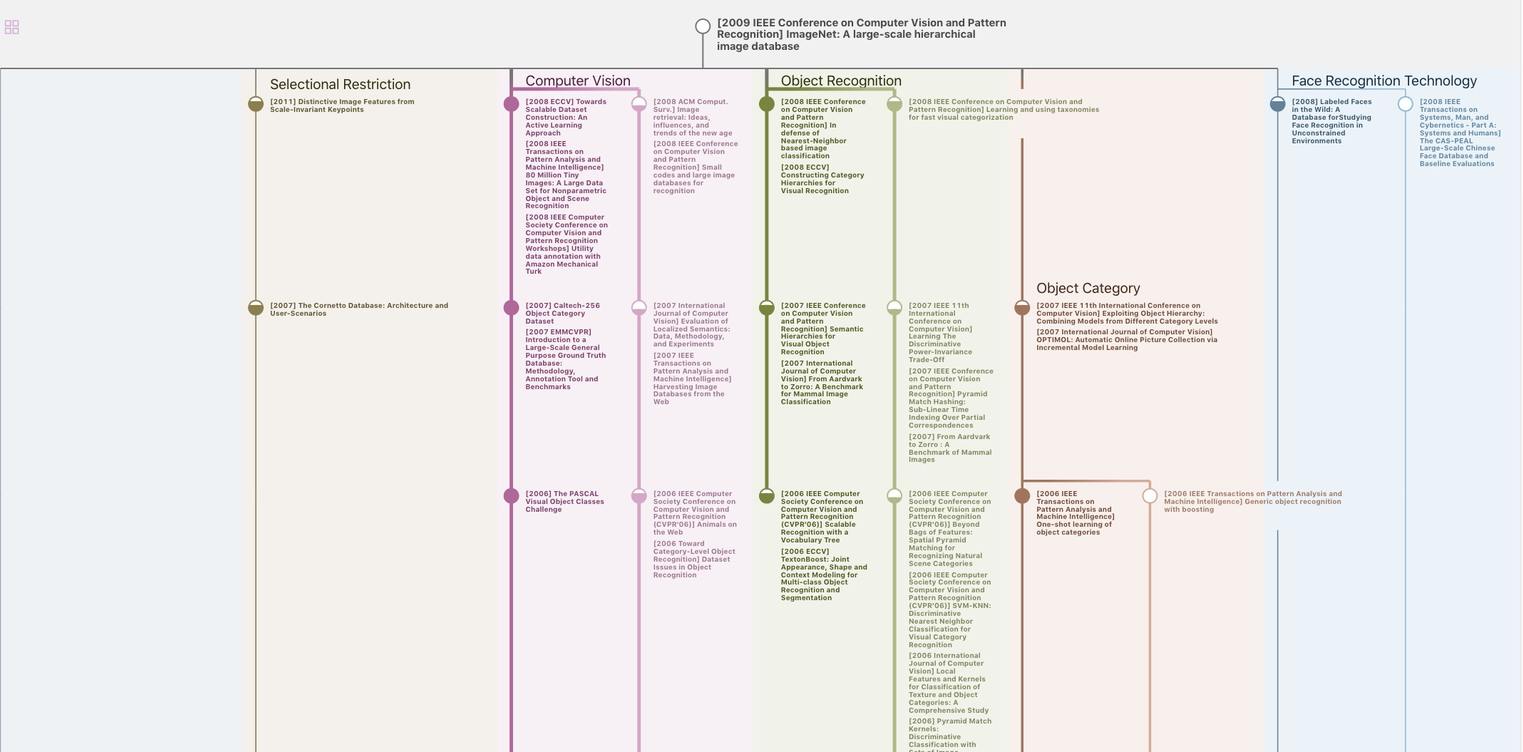
生成溯源树,研究论文发展脉络
Chat Paper
正在生成论文摘要