Using Model-Based Trees with Boosting to Fit Low-Order Functional ANOVA Models
arxiv(2023)
摘要
Low-order functional ANOVA (fANOVA) models have been rediscovered in the machine learning (ML) community under the guise of inherently interpretable machine learning. Explainable Boosting Machines or EBM (Lou et al. 2013) and GAMI-Net (Yang et al. 2021) are two recently proposed ML algorithms for fitting functional main effects and second-order interactions. We propose a new algorithm, called GAMI-Tree, that is similar to EBM, but has a number of features that lead to better performance. It uses model-based trees as base learners and incorporates a new interaction filtering method that is better at capturing the underlying interactions. In addition, our iterative training method converges to a model with better predictive performance, and the embedded purification ensures that interactions are hierarchically orthogonal to main effects. The algorithm does not need extensive tuning, and our implementation is fast and efficient. We use simulated and real datasets to compare the performance and interpretability of GAMI-Tree with EBM and GAMI-Net.
更多查看译文
关键词
models,trees,model-based,low-order
AI 理解论文
溯源树
样例
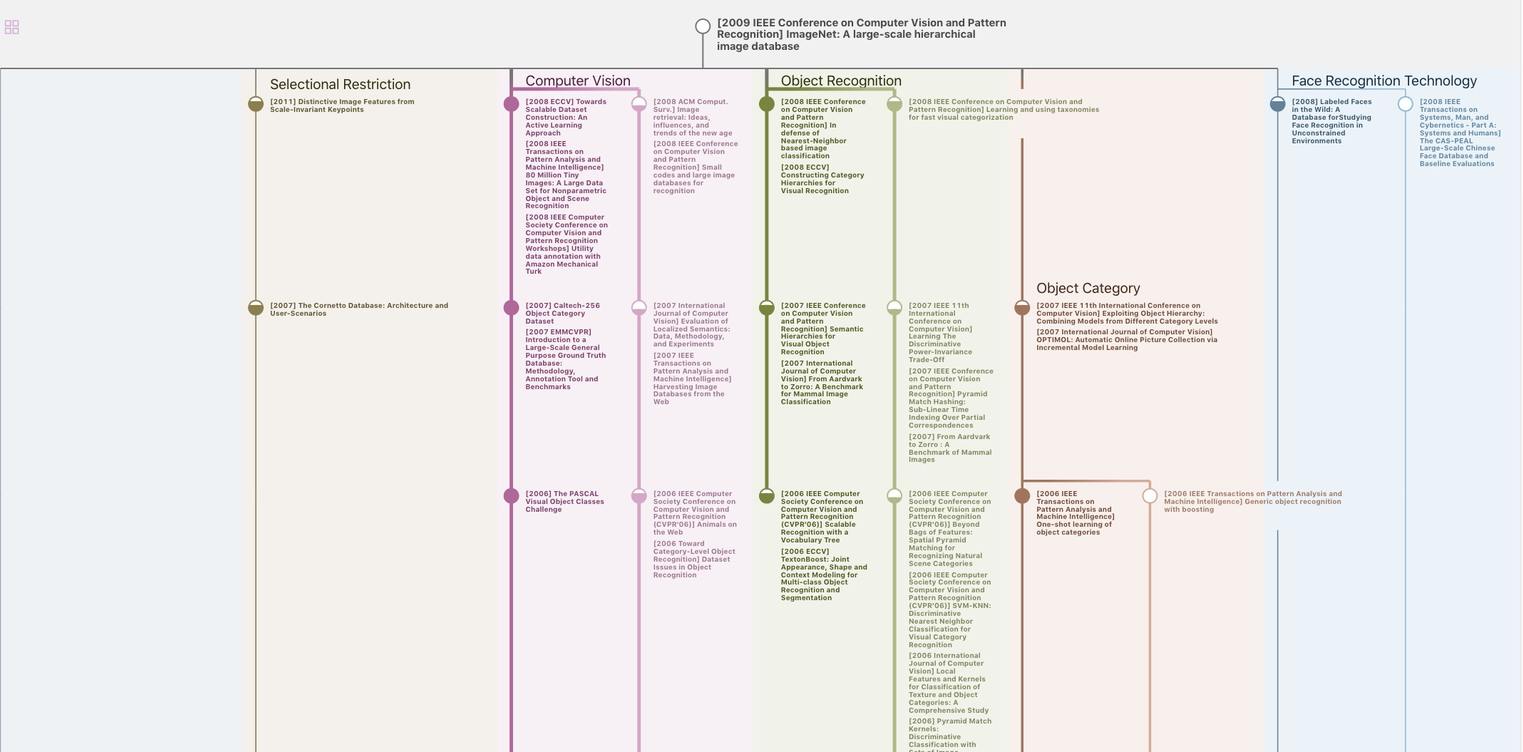
生成溯源树,研究论文发展脉络
Chat Paper
正在生成论文摘要