A Compute-in-Memory Hardware Accelerator Design With Back-End-of-Line (BEOL) Transistor Based Reconfigurable Interconnect
IEEE Journal on Emerging and Selected Topics in Circuits and Systems(2022)
摘要
Compute-in-memory (CIM) paradigm using ferroelectric field effect transistor (FeFET) as the weight element is projected to exhibit excellent energy efficiency for accelerating deep neural network (DNN) inference. However, two challenges exist. On the technology level, the chip area scaling is stalled due to the lack of logic voltage compatible FeFET at leading-edge technology node, e. g. 7nm. On the system level, CIM-based inference engine designs are usually customized for a specific DNN model, lacking the flexibility to support different DNN models. Besides, communication latency varies across different DNN models and can bound the total inference latency. Therefore, a reconfigurable interconnect is desired to be adaptive to different workloads, which can induce high area cost due to the reconfigurable circuit modules. To solve these issues, in this work, a system-technology co-design (STCO) of a monolithic 3D (M3D) reconfigurable CIM accelerator is performed, where back-end-of-line (BEOL) compatible oxide channel MOSFET and FeFET technologies are utilized. On the technology level, W-doped indium oxide (IWO) NMOS is utilized to design area-efficient M3D write circuit. On the system level, a reconfigurable interconnect design that inserts workload-specific express link is proposed, where the IWO-based NMOS and FeFET are adopted as the building element of the mux and crossbar switch in the router. The algorithm for interconnect configuration is also devised to achieve optimal latency for different workloads. From the system-level evaluation results, M3D IWO FeFET design (utilizing a hybrid 22nm/7nm M3D partition) shows
$3.1\times $
times higher energy efficiency than a 7nm 2D SRAM design with comparable chip area. With the proposed reconfigurable interconnect scheme, the interconnect latency is reduced by 9%~32% compared to the baseline with a regular mesh network.
更多查看译文
关键词
Back-end-of-line transistor,compute-in-memory,deep neural network,monolithic 3D integration,system-technology co-design
AI 理解论文
溯源树
样例
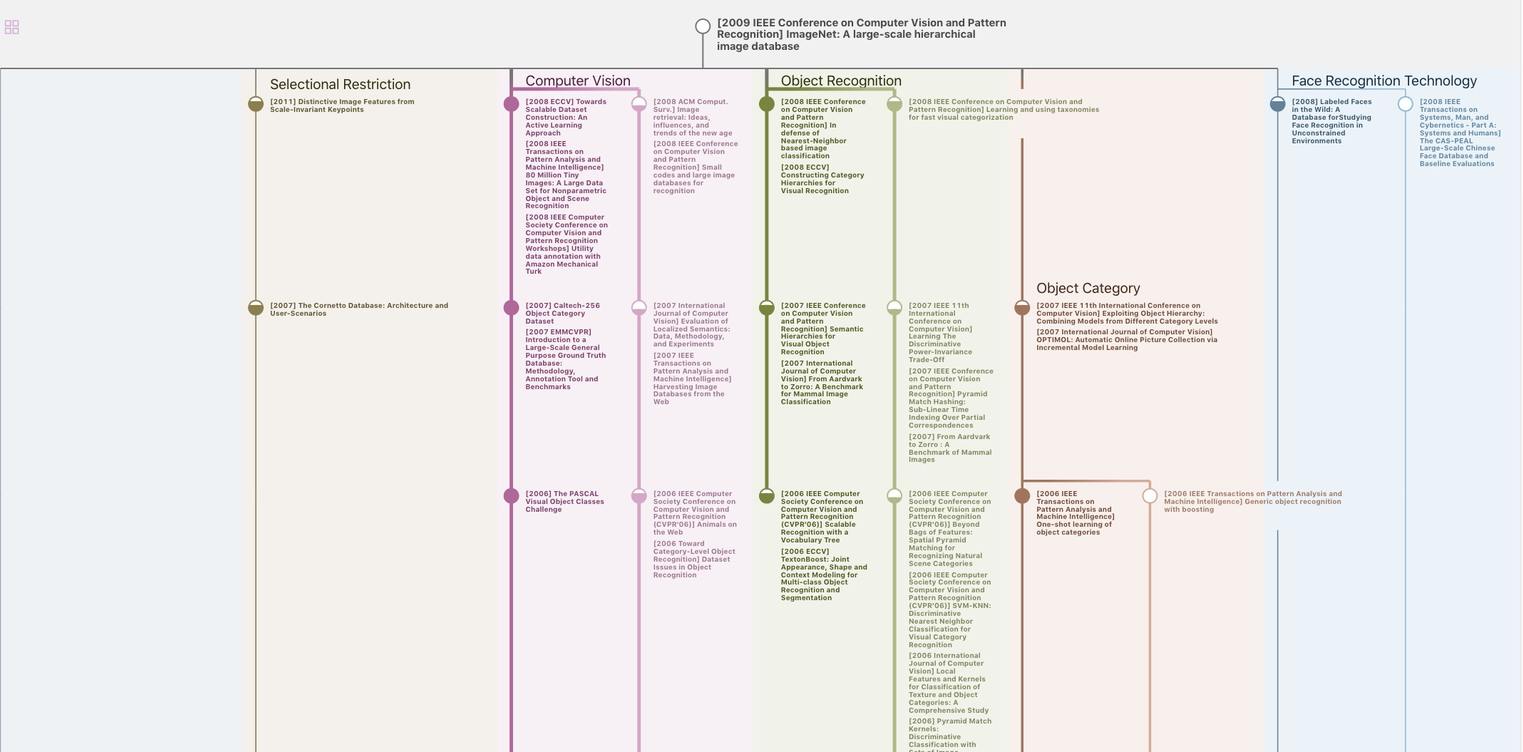
生成溯源树,研究论文发展脉络
Chat Paper
正在生成论文摘要