MLFNet: Multi-Level Fusion Network for Real-Time Semantic Segmentation of Autonomous Driving
IEEE Transactions on Intelligent Vehicles(2023)
摘要
The tradeoff between speed and accuracy is important in semantic segmentation problems, especially for resource-constrained platforms, such as intelligent vehicles. In this paper, we address this issue by proposing a well-deployed real-time semantic segmentation architecture named MLFNet. Specifically, we first build a lightweight backbone (SEFE) with a larger receptive field and multi-scale contextual representation performance to encode the pixel-level features. For better preserving target boundaries and contours, a spatial compensation branch (SPFE) is designed to gradually reduce the dimension of feature maps and refine the low-level specifics. In the decoding phase, we introduce a well-designed multi-branch fusion extractor (MBFD) for integrating the spatial details into high-level layers. Finally, the outputs from the semantic and spatial branches are fused to predict the final segmentation results. Extensive offline and online experiments have shown that our model has a superior speed and accuracy trade-off. On the Cityscapes test dataset, our model (MLFNet-Res18) achieves 71.0% mIoU with 95.1 FPS for 512 × 1024 inputs, and 72.1% mIoU with 72.2 FPS while inferring on MLFNet-Res34 model. Meanwhile, MLFNet-Res18 can reach 24.5 FPS when deployed to NVIDIA Jetson AGX Xavier and 64.0 FPS with an experimental vehicle.
更多查看译文
关键词
Autonomous driving,deep learning,double-branch encoder,real-time semantic segmentation
AI 理解论文
溯源树
样例
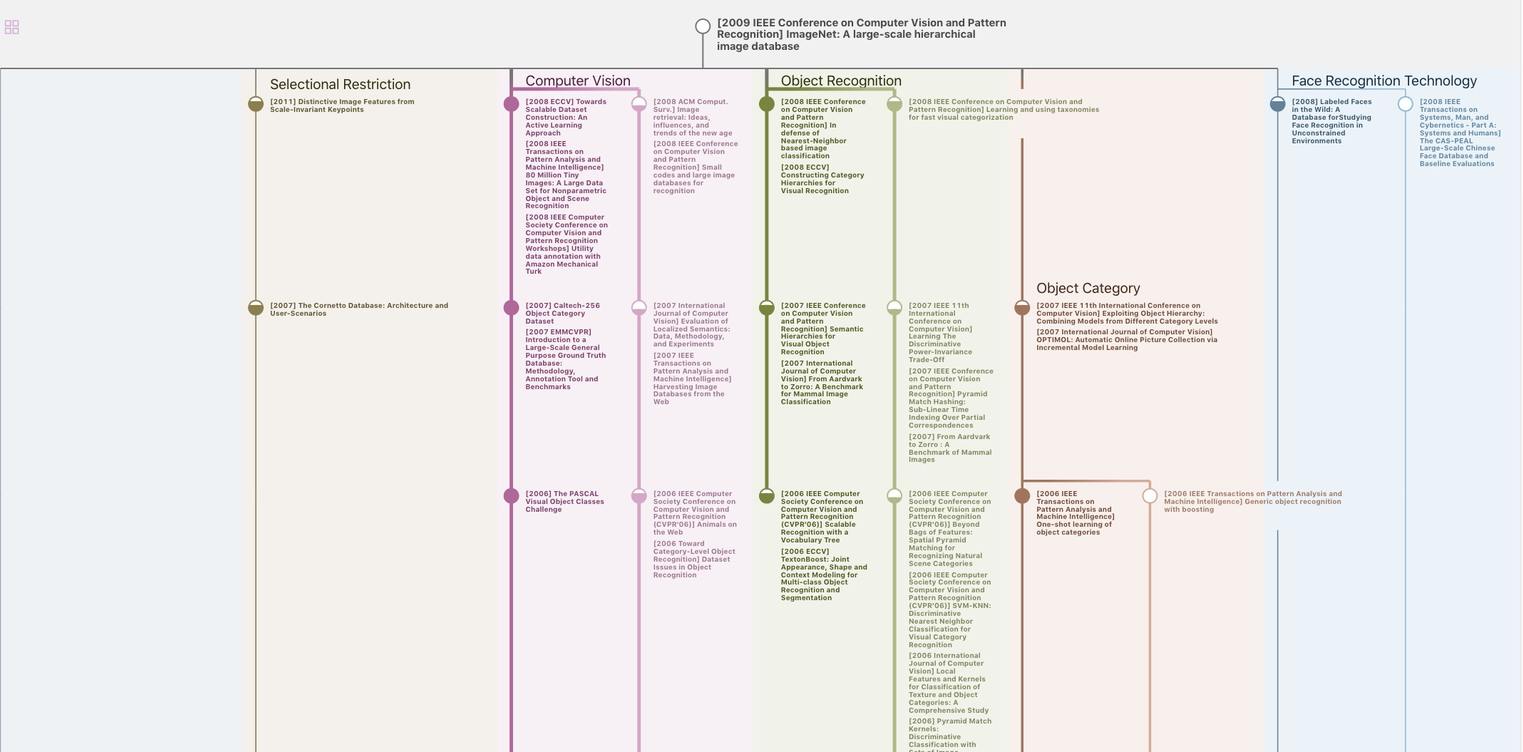
生成溯源树,研究论文发展脉络
Chat Paper
正在生成论文摘要