Selecting the Best Part From Multiple Laplacian Autoencoders for Multi-View Subspace Clustering
IEEE Transactions on Knowledge and Data Engineering(2023)
摘要
The multi-view subspace clustering attracts much attention in recent years. Most methods follow the framework of fusing the affinity graph learned in each view. In this framework, both the fusion strategy and built graph of each view are very important. In this paper, we propose novel methods for multi-view subspace clustering to address these two aspects. On the one hand, we adopt the autoencoders with Laplacian regularization to construct the affinity graph in each view. Compared with previous work employing the autoencoders, the Laplacian term in our method can guide the learned latent representation favoring affinity extraction. Besides, we also discuss the reasons for adding Laplacian regularization. On the other hand, we propose a novel fusion strategy distinguished from the related literature. If the affinity graph of some view is not extracted well, the performance of previous fusion strategies will be seriously affected. Since our strategy can choose the best part from each affinity graph, it can overcome this limitation to some extent. Extensive experimental results on multiple benchmark data sets confirm the effectiveness of our method.
更多查看译文
关键词
Laplace equations,Neural networks,Deep learning,Faces,Clustering methods,Sun,Feature extraction,Multi-view,subspace clustering,autoencoders,Laplacian operator,novel fusion strategy
AI 理解论文
溯源树
样例
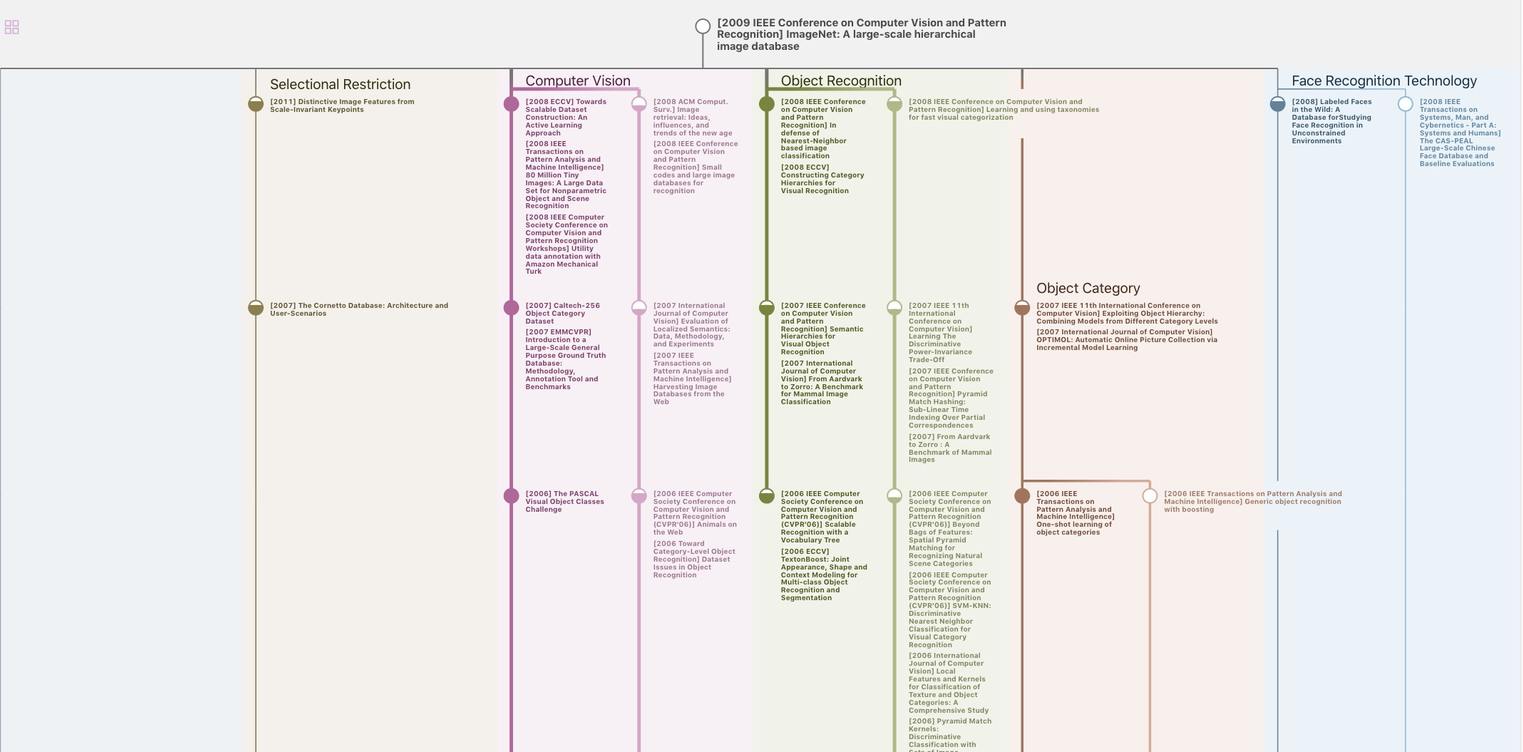
生成溯源树,研究论文发展脉络
Chat Paper
正在生成论文摘要