Evolutionary Computation in Action: Hyperdimensional Deep Embedding Spaces of Gigapixel Pathology Images
IEEE Transactions on Evolutionary Computation(2023)
摘要
One of the main obstacles of adopting digital pathology is the challenge of efficient processing of hyperdimensional digitized biopsy samples, called whole slide images (WSIs). Exploiting deep learning and introducing compact WSI representations are urgently needed to accelerate image analysis and facilitate the visualization and interpretability of pathology results in a postpandemic world. In this article, we introduce a new evolutionary approach for WSI representation based on large-scale multiobjective optimization (LSMOP) of deep embeddings. We start with patch-based sampling to feed KimiaNet, a histopathology-specialized deep network, and to extract a multitude of feature vectors. Coarse multiobjective feature selection uses the reduced search space strategy guided by the classification accuracy and the number of features. In the second stage, the frequent features histogram (FFH), a novel WSI representation, is constructed by multiple runs of coarse LSMOP. Fine evolutionary feature selection is then applied to find a compact (short-length) feature vector based on the FFH and contributes to a more robust deep-learning approach to digital pathology supported by the stochastic power of evolutionary algorithms. We validate the proposed schemes using The Cancer Genome Atlas (TCGA) images in terms of WSI representation, classification accuracy, and feature quality. Furthermore, a novel decision space for multicriteria decision making in the LSMOP field is introduced. Finally, a patch-level visualization approach is proposed to increase the interpretability of deep features. The proposed evolutionary algorithm finds a very compact feature vector to represent a WSI (almost 14000 times smaller than the original feature vectors) with 8% higher accuracy compared to the codes provided by the state-of-the-art methods
更多查看译文
关键词
Deep neural network (DNN),digital pathology,dimension reduction,evolutionary computation (EC),feature selection,image representation,innovization,large-scale multiobjective optimization (LSMOP),transfer learning
AI 理解论文
溯源树
样例
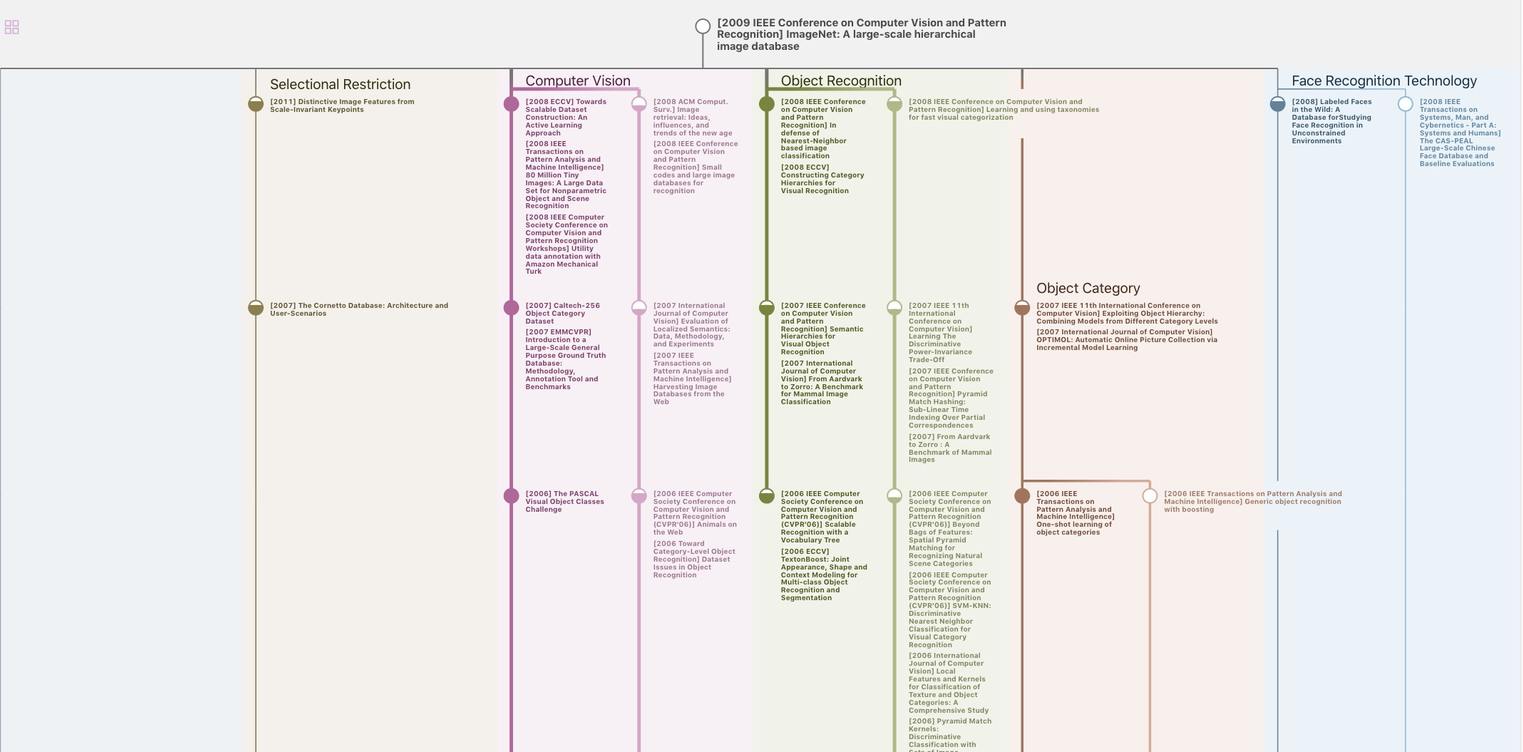
生成溯源树,研究论文发展脉络
Chat Paper
正在生成论文摘要