DGPO: Discovering Multiple Strategies with Diversity-Guided Policy Optimization
AAAI 2024(2024)
摘要
Most reinforcement learning algorithms seek a single optimal strategy that solves a given task. However, it can often be valuable to learn a diverse set of solutions, for instance, to make an agent's interaction with users more engaging, or improve the robustness of a policy to an unexpected perturbance. We propose Diversity-Guided Policy Optimization (DGPO), an on-policy algorithm that discovers multiple strategies for solving a given task. Unlike prior work, it achieves this with a shared policy network trained over a single run. Specifically, we design an intrinsic reward based on an information-theoretic diversity objective. Our final objective alternately constraints on the diversity of the strategies and on the extrinsic reward. We solve the constrained optimization problem by casting it as a probabilistic inference task and use policy iteration to maximize the derived lower bound. Experimental results show that our method efficiently discovers diverse strategies in a wide variety of reinforcement learning tasks. Compared to baseline methods, DGPO achieves comparable rewards, while discovering more diverse strategies, and often with better sample efficiency.
更多查看译文
关键词
ML: Reinforcement Learning,ML: Deep Learning Algorithms
AI 理解论文
溯源树
样例
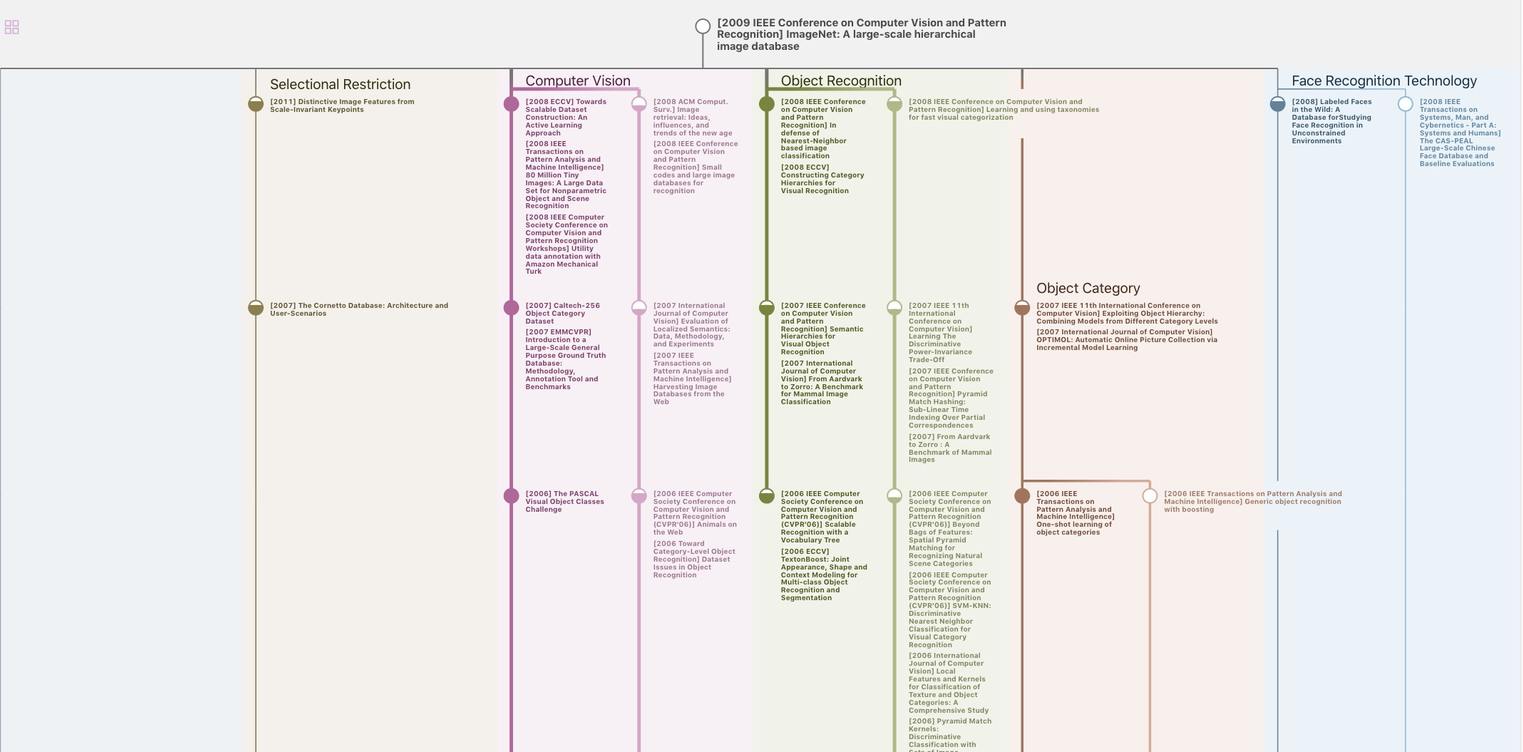
生成溯源树,研究论文发展脉络
Chat Paper
正在生成论文摘要