An improved recommendation based on graph convolutional network
JOURNAL OF INTELLIGENT INFORMATION SYSTEMS(2022)
摘要
Graph convolutional network is a recently developed artificial neural network method commonly used in recommendation system research. This paper points out three shortcomings of existing recommendation systems based on the graph convolutional network. 1. Existing models that take the one-hot encoding based on node ordinal numbers in the graph or encoding based on original entity attributes as input may not fully utilize the information carried by the attribute interactions. 2. Previous models update the node embeddings only by the first-order neighbors in the graph convolution layer, which is easily affected by noise. 3. Existing models do not take into account differences in user opinions. We propose an improved graph convolutional network-based collaborative filtering model to address these drawbacks. We identify inner and cross interaction between user attributes and item attributes, and then we take the vector representations of aggregated attributes graph as input. In the convolutional layer, we aggregate the second-order collaborative signals and incorporate the different user opinions. The experiments on three public datasets show that our model outperforms state-of-the-art models.
更多查看译文
关键词
Graph convolutional network,High-order collaborative signal,Collaborative filtering,Recommendation system
AI 理解论文
溯源树
样例
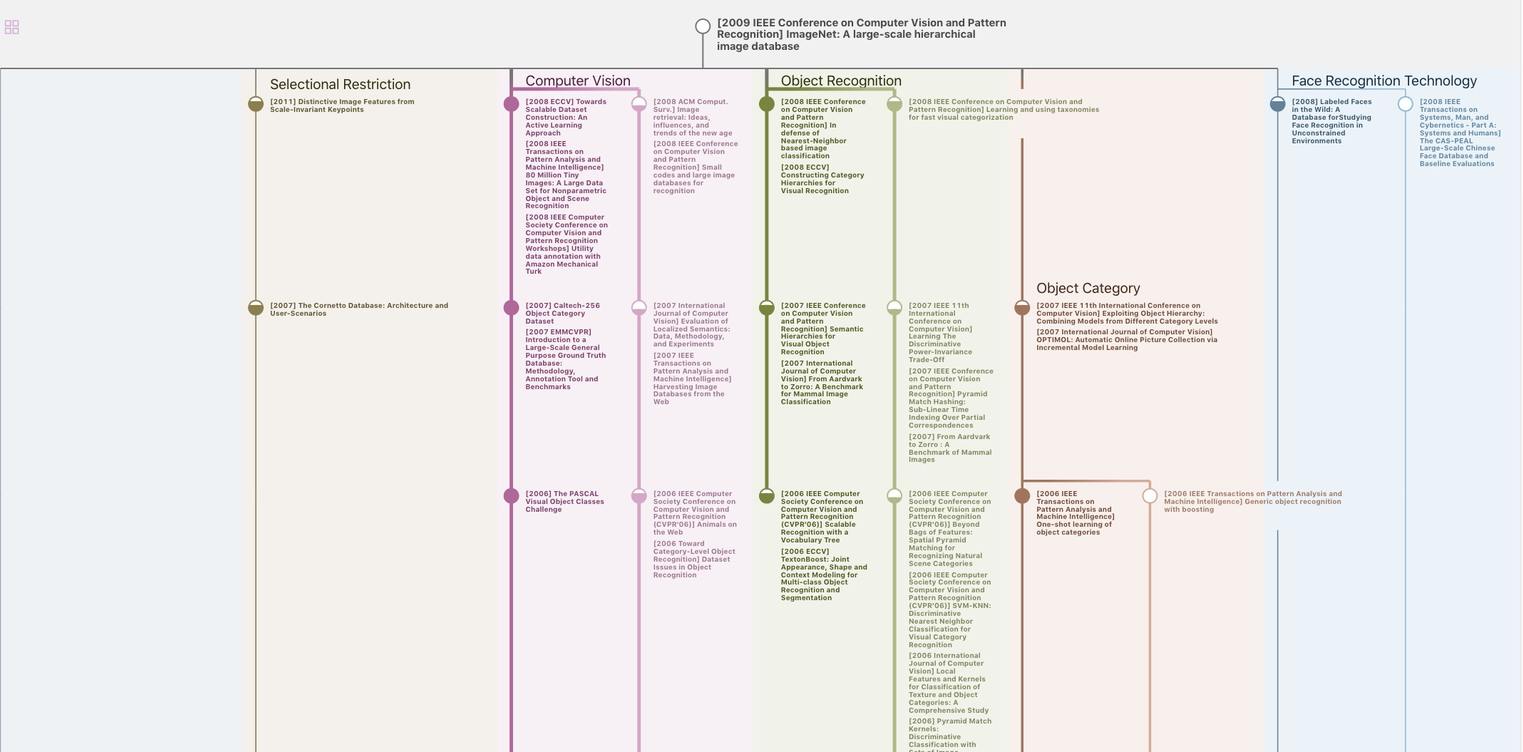
生成溯源树,研究论文发展脉络
Chat Paper
正在生成论文摘要