High-Frequency Normalizing Flow for Image Rescaling
IEEE transactions on image processing : a publication of the IEEE Signal Processing Society(2023)
摘要
It is desirable to develop efficient image rescaling methods to transmit digital images with different resolutions between devices and assure visual quality. In image downscaling, the inevitable loss of high-frequency information makes the reverse upscaling highly ill-posed. Recent approaches focus on joint learning of image downscaling and upscaling (e.g., rescaling). However, existing methods still fail to recover satisfactory high-frequency signals when upscaling. To solve it, we propose high-frequency flow (HfFlow), which learns the distribution of high-frequency signals during rescaling. HfFlow is an overall invertible framework with a conditional flow on the high-frequency space to compensate for the information lost during downscaling. To facilitate finding the optimal upscaling solution, we introduce a reference low-resolution (LR) manifold and propose a cross-entropy Gaussian loss (CGloss) to force the downscaled manifold closer to the reference LR manifold and simultaneously fulfill recovering missing details. HfFlow can be generalized to other scale transformation tasks such as image colorization with its excellent rescaling capacity. Qualitative and quantitative experimental evaluations demonstrate that HfFlow restores rich high-frequency details and outperforms state-of-the-art rescaling methods in PSNR, SSIM, and perceptual quality metrics.
更多查看译文
关键词
Image rescaling,normalizing flow,high-frequency signal reconstruction
AI 理解论文
溯源树
样例
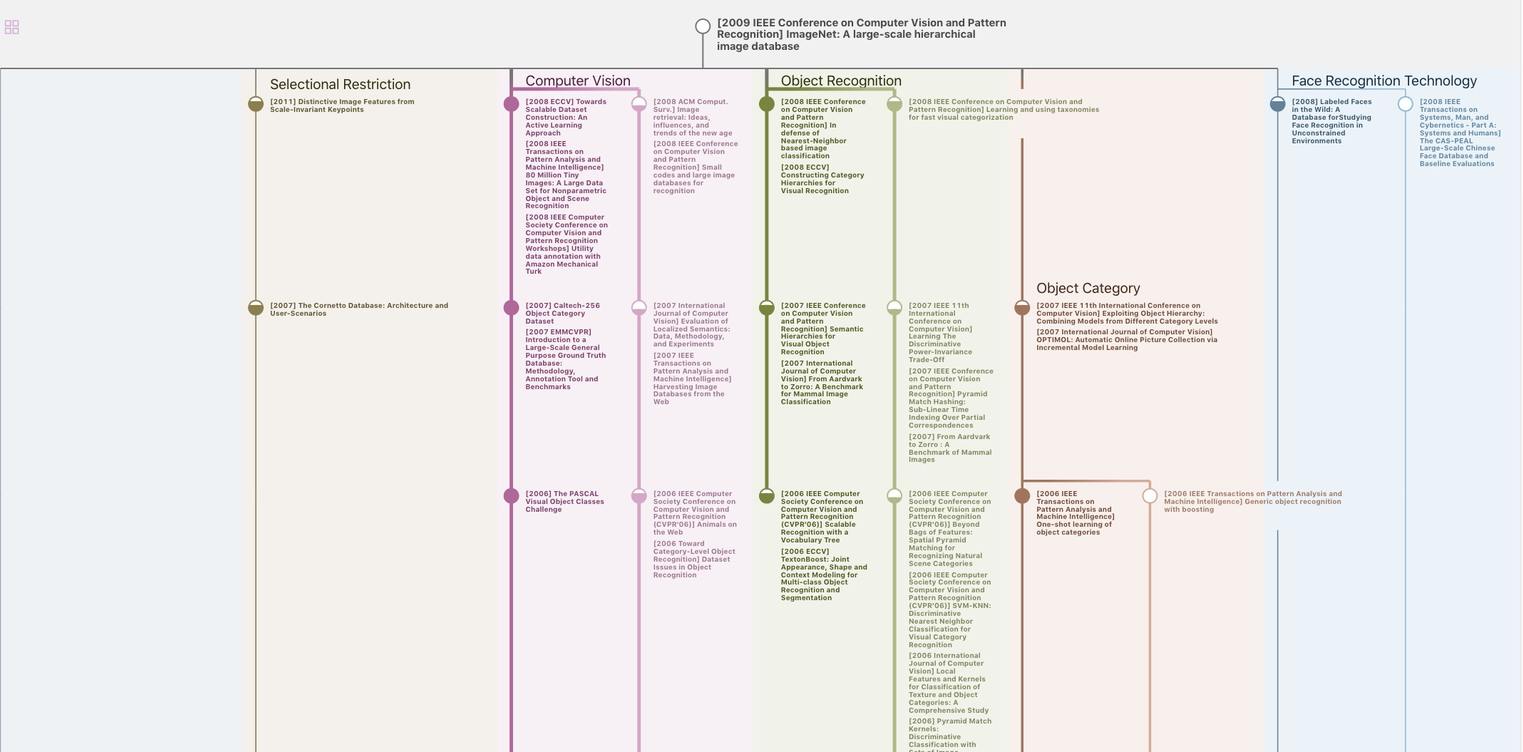
生成溯源树,研究论文发展脉络
Chat Paper
正在生成论文摘要