Electroencephalograph-Based Emotion Recognition Using Brain Connectivity Feature and Domain Adaptive Residual Convolution Model
FRONTIERS IN NEUROSCIENCE(2022)
摘要
In electroencephalograph (EEG) emotion recognition research, obtaining high-level emotional features with more discriminative information has become the key to improving the classification performance. This study proposes a new end-to-end emotion recognition method based on brain connectivity (BC) features and domain adaptive residual convolutional network (short for BC-DA-RCNN), which could effectively extract the spatial connectivity information related to the emotional state of the human brain and introduce domain adaptation to achieve accurate emotion recognition within and across the subject's EEG signals. The BC information is represented by the global brain network connectivity matrix. The DA-RCNN is used to extract high-level emotional features between different dimensions of EEG signals, reduce the domain offset between different subjects, and strengthen the common features between different subjects. The experimental results on the large public DEAP data set show that the accuracy of the subject-dependent and subject-independent binary emotion classification in valence reaches 95.15 and 88.28%, respectively, which outperforms all the benchmark methods. The proposed method is proven to have lower complexity, better generalization ability, and domain robustness that help to lay a solid foundation for the development of high-performance affective brain-computer interface applications.
更多查看译文
关键词
EEG, brain connectivity, residual convolution, domain adaptative, emotion recognition
AI 理解论文
溯源树
样例
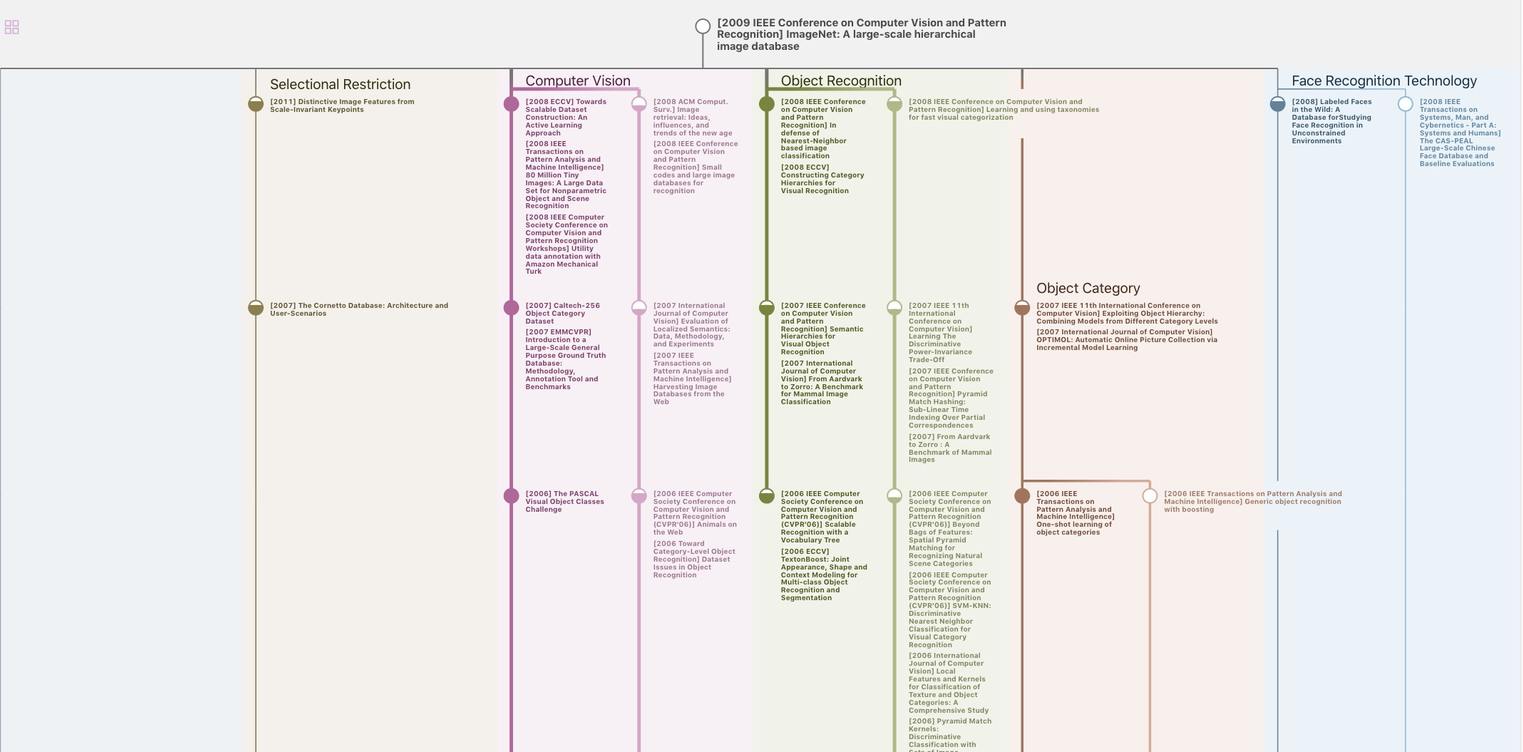
生成溯源树,研究论文发展脉络
Chat Paper
正在生成论文摘要