AttentionSiteDTI: an interpretable graph-based model for drug-target interaction prediction using NLP sentence-level relation classification
BRIEFINGS IN BIOINFORMATICS(2022)
摘要
In this study, we introduce an interpretable graph-based deep learning prediction model, AttentionSiteDTI, which utilizes protein binding sites along with a self-attention mechanism to address the problem of drug-target interaction prediction. Our proposed model is inspired by sentence classification models in the field of Natural Language Processing, where the drug-target complex is treated as a sentence with relational meaning between its biochemical entities a.k.a. protein pockets and drug molecule. AttentionSiteDTI enables interpretability by identifying the protein binding sites that contribute the most toward the drug-target interaction. Results on three benchmark datasets show improved performance compared with the current state-of-the-art models. More significantly, unlike previous studies, our model shows superior performance, when tested on new proteins (i.e. high generalizability). Through multidisciplinary collaboration, we further experimentally evaluate the practical potential of our proposed approach. To achieve this, we first computationally predict the binding interactions between some candidate compounds and a target protein, then experimentally validate the binding interactions for these pairs in the laboratory. The high agreement between the computationally predicted and experimentally observed (measured) drug-target interactions illustrates the potential of our method as an effective pre-screening tool in drug repurposing applications.
更多查看译文
关键词
Deep learning,Self-Attention,Binding Sites,Machine learning,drug-target interaction,SARS-CoV-2,DTI software,DTI database
AI 理解论文
溯源树
样例
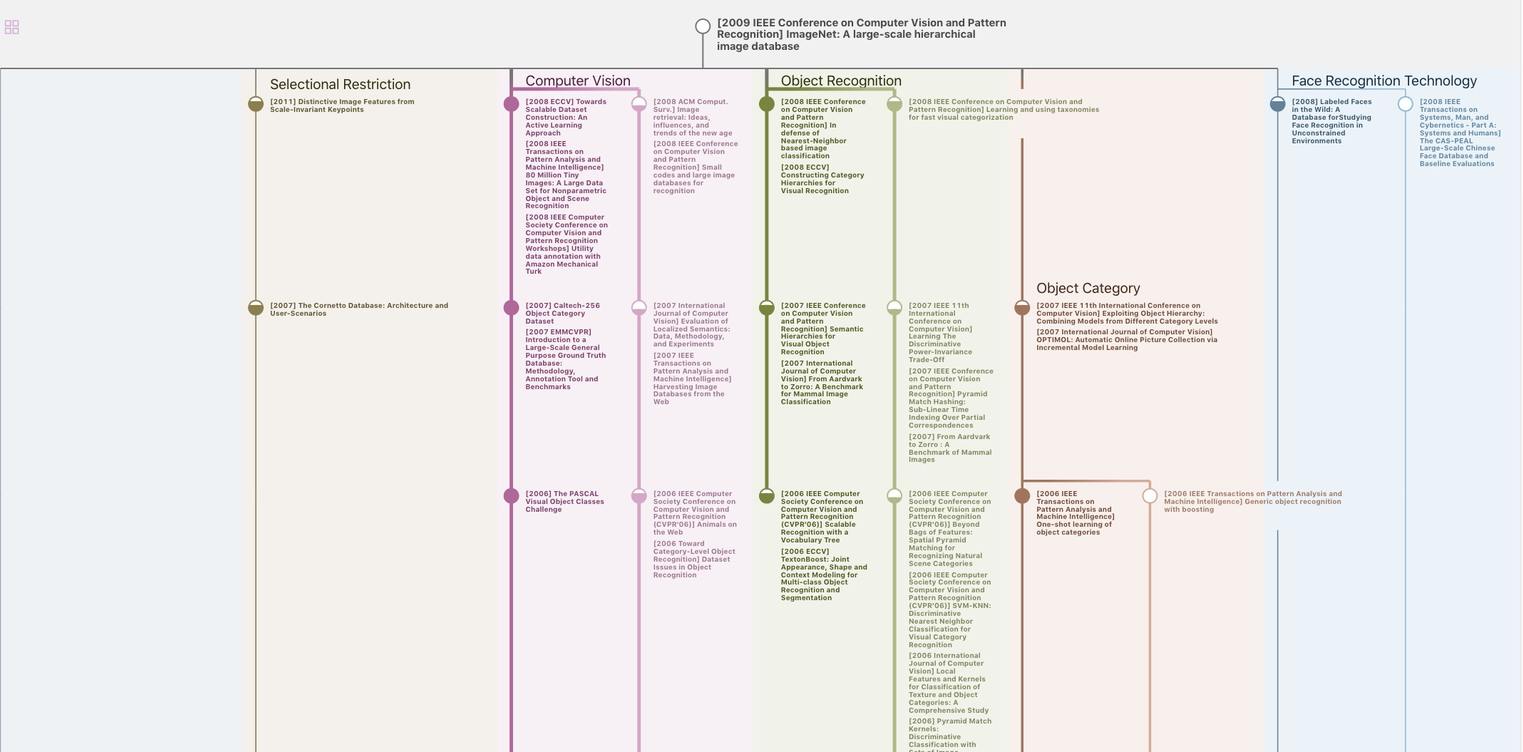
生成溯源树,研究论文发展脉络
Chat Paper
正在生成论文摘要