DeepSNR: A deep learning foundation for offline gravitational wave detection
arxiv(2022)
摘要
All scientific claims of gravitational wave discovery to date rely on the offline statistical analysis of candidate observations in order to quantify significance relative to background processes. The current foundation in such offline detection pipelines in experiments at LIGO is the matched-filter algorithm, which produces a signal-to-noise-ratio-based statistic for ranking candidate observations. Existing deep-learning-based attempts to detect gravitational waves, which have shown promise in both signal sensitivity and computational efficiency, output probability scores. However, probability scores are not easily integrated into discovery workflows, limiting the use of deep learning thus far to non-discovery-oriented applications. In this paper, the Deep Learning Signal-to-Noise Ratio (DeepSNR) detection pipeline, which uses a novel method for generating a signal-to-noise ratio ranking statistic from deep learning classifiers, is introduced, providing the first foundation for the use of deep learning algorithms in discovery-oriented pipelines. The performance of DeepSNR is demonstrated by identifying binary black hole merger candidates versus noise sources in open LIGO data from the first observation run. High-fidelity simulations of the LIGO detector responses are used to present the first sensitivity estimates of deep learning models in terms of physical observables. The robustness of DeepSNR under various experimental considerations is also investigated. The results pave the way for DeepSNR to be used in the scientific discovery of gravitational waves and rare signals in broader contexts, potentially enabling the detection of fainter signals and never-before-observed phenomena.
更多查看译文
关键词
offline gravitational wave
AI 理解论文
溯源树
样例
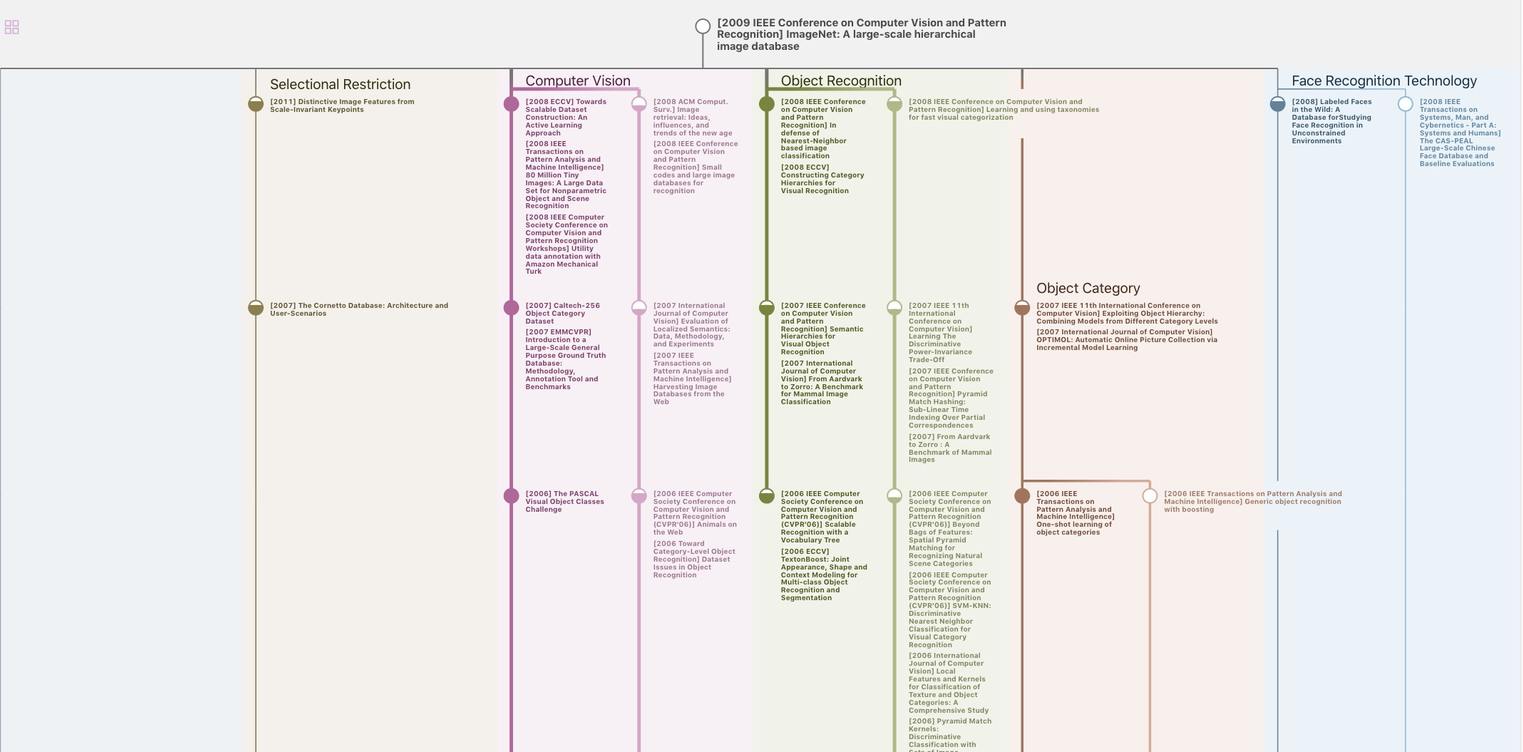
生成溯源树,研究论文发展脉络
Chat Paper
正在生成论文摘要