Distillation, Ensemble and Selection for Building a Better and Faster Siamese Based Tracker
IEEE TRANSACTIONS ON CIRCUITS AND SYSTEMS FOR VIDEO TECHNOLOGY(2024)
摘要
Visual object tracking has witnessed continuous improvements in performance, thanks to deep CNN learning that recently emerged. More complex CNN models invariably offer better accuracy. However, there is a conflict between the tracking efficiency and model complexity, which poses a challenge in balancing speed against accuracy. To optimize the trade-off between these two performance criteria, a distillation-ensemble-selection framework is proposed in this paper. Without any modification to the baseline network architecture, the proposed approach enables the construction of a Siamese-based tracker with improved capacity and efficiency. Specifically, multiple student trackers are designed by means of knowledge distillation from a given teacher tracking model. To manage the varying granularity of unknown targets, an ensemble module combines the outputs of the student trackers with the help of a learnable fine-grained attention module. Besides, in the online tracking stage, a selection module adaptively controls the complexity of the tracker by identifying an appropriate subset of the candidate tracker models. We verify the effectiveness of the proposed method in both anchor-based and anchor-free paradigms. The experimental results obtained on standard benchmarking datasets demonstrate the effectiveness of the proposed method, with an outstanding and balanced performance in both accuracy and speed.
更多查看译文
关键词
Visual object tracking,Siamese tracker,task fusion,target-agnostic detection
AI 理解论文
溯源树
样例
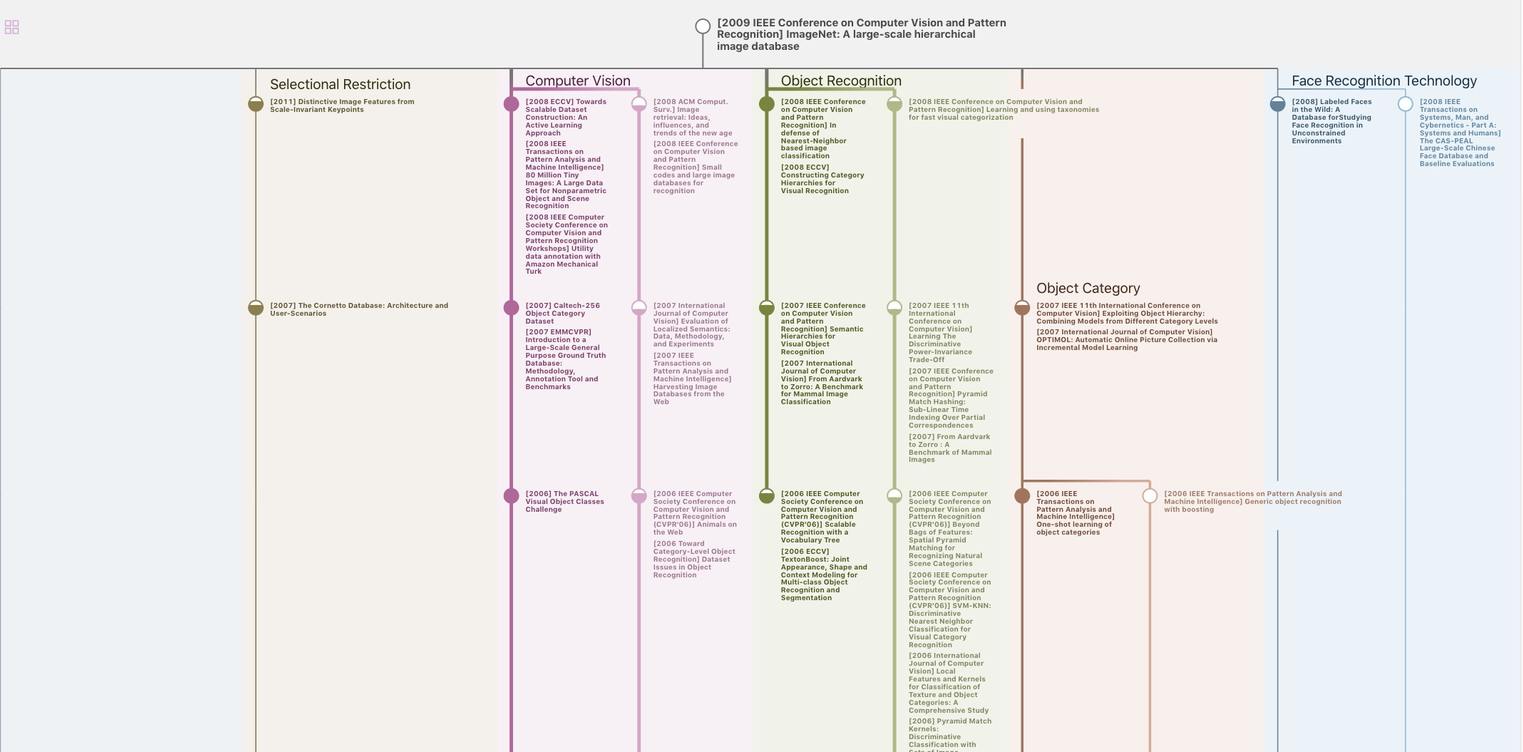
生成溯源树,研究论文发展脉络
Chat Paper
正在生成论文摘要