Synthetic Image Data Generation for Semantic Understanding in Everchanging Scenes Using BIM and Unreal Engine
Computing in Civil Engineering 2021(2022)
摘要
Scene understanding, such as object recognition and semantic segmentation on images, plays an important role in many existing information management workflows such as progress monitoring and facility management. Current studies on architecture/engineering/construction (AEC) scene understanding often focus on using visual data captured for a particular building at project closeout, which has two primary limitations: (1) A scene understanding model trained based on static images collected at project closeout data often is not useful for understanding an ever-changing scene where facility components could have intermediate and incomplete states, such as construction sites; and (2) many facility components are occluded at project closeout, which creates many challenges when labeling or detecting them. By leveraging as-designed information present in building information models, this paper proposed an approach to generate synthetic data for training semantic understanding models reflecting the changes in site conditions through 4D-BIM and Unreal Engine. The paper contains two primary contributions: (1) The proposed workflow addresses issues with changing scenes by generating synthetic images with ground truth semantic segmentations during any stage of construction based on given schedule information and (2) the proposed method reduced the labeling effort by utilizing the semantically rich as-designed information that exists in a BIM. The proposed workflow was tested on an academic building in its ability to create a useful synthetic data set using the Uniformat (2010) as semantic taxonomy. The experiment results showed that the proposed data augmentation can improve the ability of scene understanding for images captured in changing scenes.
更多查看译文
AI 理解论文
溯源树
样例
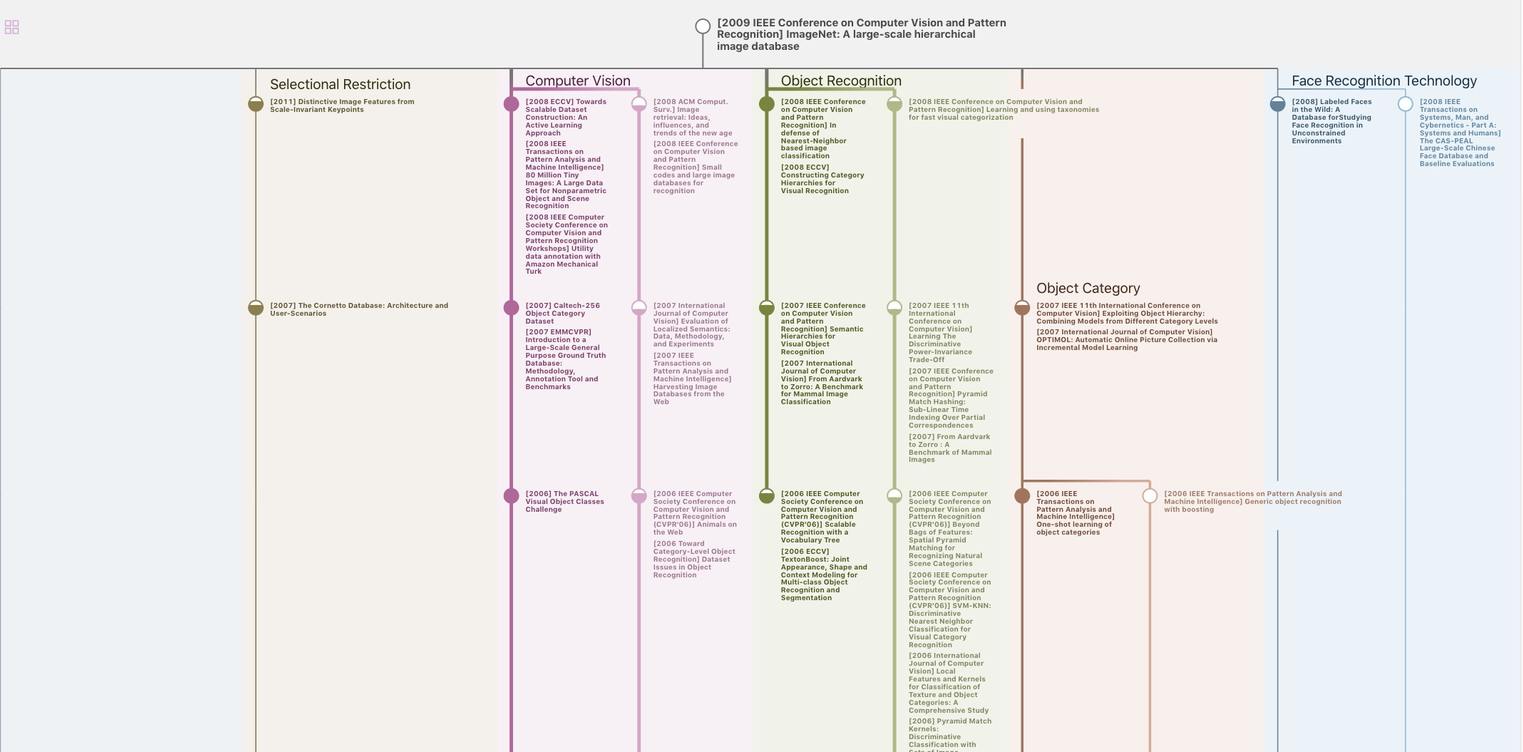
生成溯源树,研究论文发展脉络
Chat Paper
正在生成论文摘要