Machine Learning Assisted Screening of Mxenes Pseudocapacitive Materials
SSRN Electronic Journal(2022)
摘要
Distinguishing key features is crucial to design supercapacitor materials upon transition-metal carbides and nitrides (MXenes). Herein, the machine learning strategy was adopted to explore the structure-property based on 600 MXenes, including M2XT2 (T = bare, O, S) and their doped systems. To ensure the quality of the data set, all the MXenes data were calculated individually through density functional theory. The sure independence screening and sparsifying operator (SISSO) method was subsequently used to develop pseudocapacitance formulas according to the refined key features in terms of stability and electronic structures. It is found that, on the group-free surfaces, both ion adsorption strength and density of states 1.0 eV above Fermi level play crucial roles to regulate pseudocapacitance. For the surface-functionalized cases, the parameters electronegativity and specific heat are the keys to determine pseudocapacitance. Furthermore, statistical results show that the elements, leading to high pseudocapacitance, are located in the upper left, lower left and upper right regions of the periodic table for group-free, O-functionalized and S-functionalized MXenes, respectively. The structure-property relationship provides theoretical insights into the development of MXenes-based pseudocapacitive materials from a statistical point of view.
更多查看译文
关键词
Machine learning,MXenes,Supercapacitors,Electrode materials
AI 理解论文
溯源树
样例
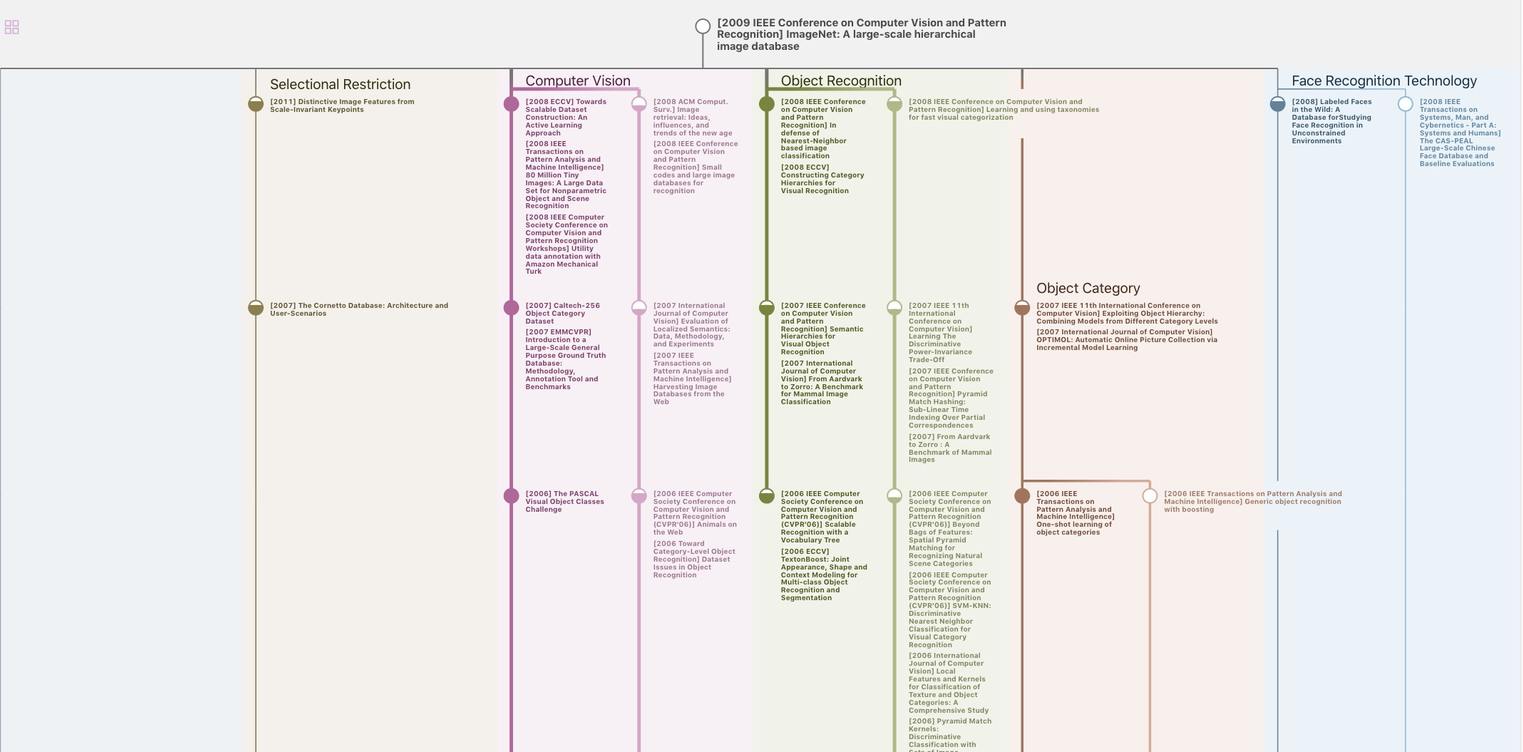
生成溯源树,研究论文发展脉络
Chat Paper
正在生成论文摘要