Robustness and reproducibility of an artificial intelligence-assisted online segmentation and adaptive planning process for online adaptive radiation therapy
JOURNAL OF APPLIED CLINICAL MEDICAL PHYSICS(2022)
摘要
Clinical implementation of online adaptive radiation therapy requires initial and ongoing performance assessment of the underlying auto-segmentation and adaptive planning algorithms, although a straightforward and efficient process for this in phantom is lacking. The purpose of this work was to investigate robustness and repeatability of the artificial intelligence-assisted online segmentation and adaptive planning process on the Varian Ethos adaptive platform, and to develop an end-to-end test strategy for online adaptive radiation therapy. Five synthetic deformations were generated and applied to a computed tomography image of an anthropomorphic pelvis phantom, and reference treatment plans were generated from each of the resulting deformed images. The undeformed phantom was repeatedly imaged, and the online adaptive process was performed including auto-segmentation, review and manual correction of contours, and adaptive plan creation. One adaptive fractions in five different deformation scenarios were performed. The manually corrected contours had a high degree of consistency (> 93% Dice similarity coefficient and < 1.0 mm mean surface distance) across repeated fractions, with no significant variation across the synthetic deformation instance except for bowel (p = 0.026, one-way ANOVA). Adaptive treatment plans also resulted in highly consistent dose-volume values for targets and organs at risk. A straightforward and efficient process was developed and used to quantify a set of organ specific contouring and dosimetric action levels to help establish uncertainty bounds for an end-to-end test on the Varian Ethos system.
更多查看译文
关键词
adaptive radiotherapy, Ethos, quality assurance
AI 理解论文
溯源树
样例
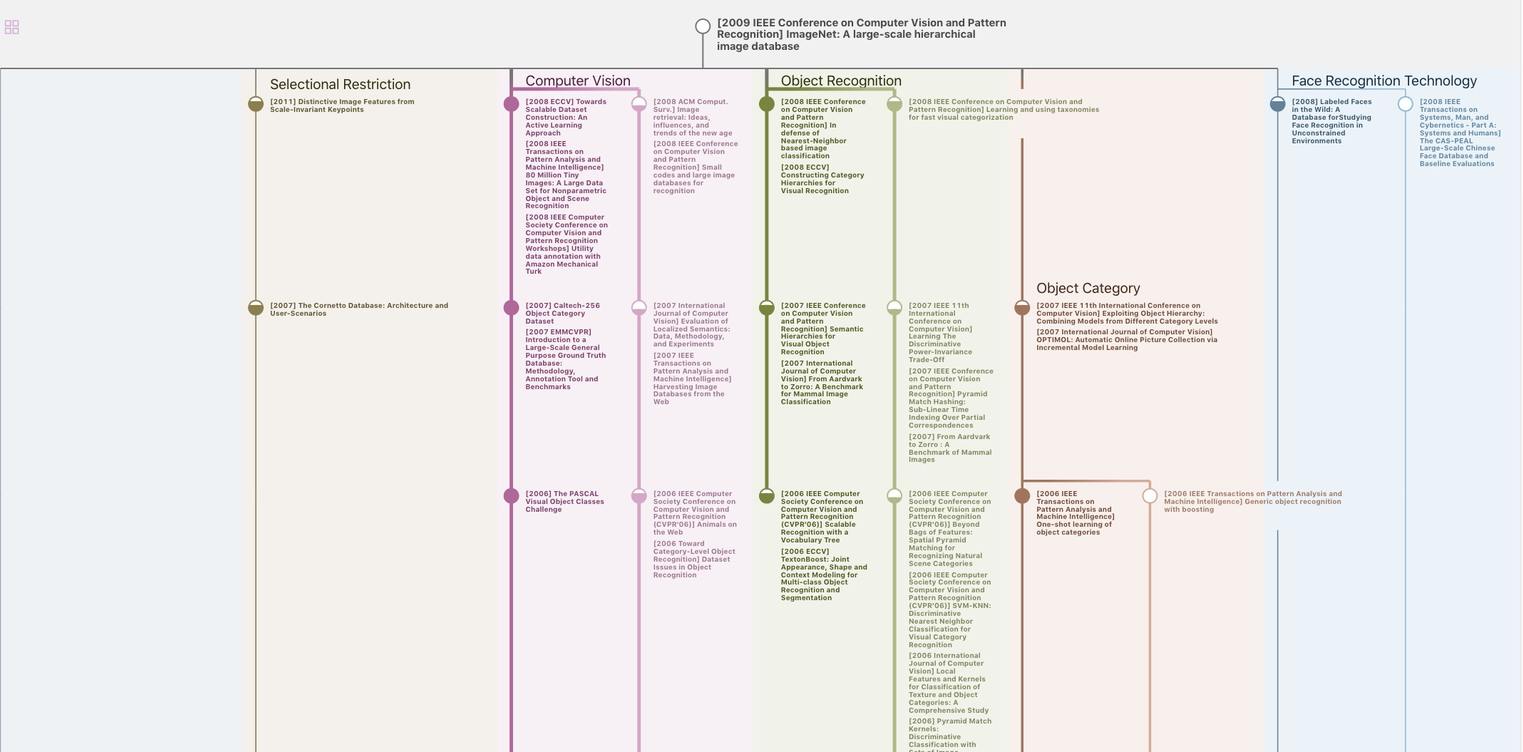
生成溯源树,研究论文发展脉络
Chat Paper
正在生成论文摘要