Low-resource Low-footprint Wake-word Detection using Knowledge Distillation
Conference of the International Speech Communication Association (INTERSPEECH)(2022)
摘要
As virtual assistants have become more diverse and specialized, so has the demand for application or brand-specific wake words. However, the wake-word-specific datasets typically used to train wake-word detectors are costly to create. In this paper, we explore two techniques to leverage acoustic modeling data for large-vocabulary speech recognition to improve a purpose-built wake-word detector: transfer learning and knowledge distillation. We also explore how these techniques interact with time-synchronous training targets to improve detection latency. Experiments are presented on the open-source "Hey Snips" dataset and a more challenging in-house far-field dataset. Using phone-synchronous targets and knowledge distillation from a large acoustic model, we are able to improve accuracy across dataset sizes for both datasets while reducing latency.
更多查看译文
关键词
detection,low-resource,low-footprint,wake-word
AI 理解论文
溯源树
样例
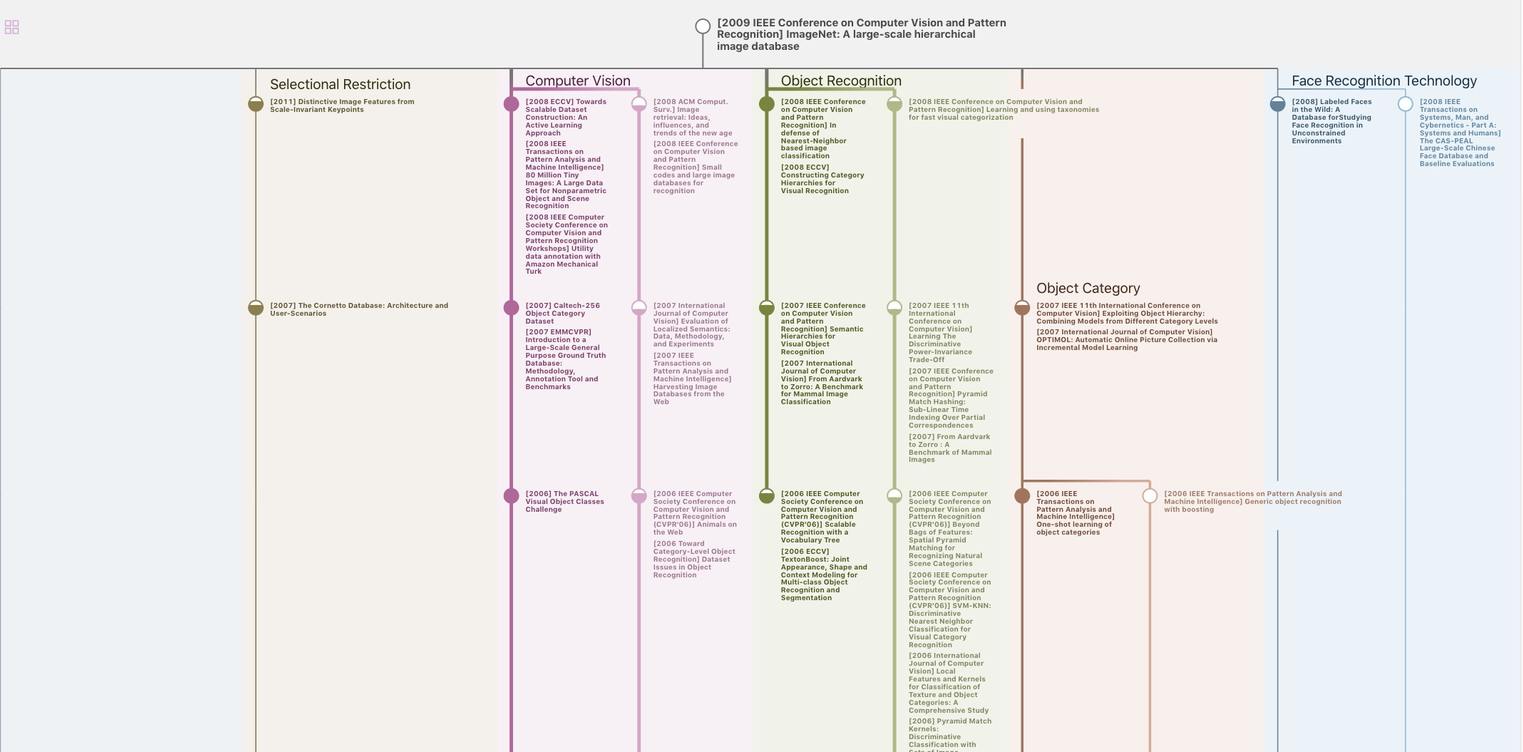
生成溯源树,研究论文发展脉络
Chat Paper
正在生成论文摘要