VWP:An Efficient DRL-Based Autonomous Driving Model
IEEE TRANSACTIONS ON MULTIMEDIA(2024)
摘要
In this paper, a novel DRL-based model (VWP, VAE-WGAN-PPOE) is proposed to solve the problem of long training time and unsatisfactory training effect in the end-to-end autonomous driving. The model is optimized from feature extraction and algorithm decision. In feature extraction, we encode the input video by combining variational auto encoder (VAE) with wasserstein generative adversarial network (WGAN). The state dimension is reduced and the problem of mode collapse and gradient disappearance caused by generative adversarial network (GAN) training is solved. In decision algorithm, we formulate a new reward function by analyzing the factors affecting driving performance. Furthermore, we propose an enhanced algorithm PPOE based on the proximal policy optimization (PPO). In the CARLA simulator, compared with CNN and ResNet34, the convergence speed of the DRL model based on VAE-WGAN increases by 26.1% and 20.3%, the navigation task completion rate increases by 18.5% and 9.2%, and the collision rate decreases by 13.6% and 9.4%. Compared with deep deterministic policy gradient (DDPG) decision algorithm, the convergence speed of the DRL model based on PPOE increases by 23.3%, the navigation task completion rate increases by 5.0% in sunny days and 8.4% in severe weather, the collision rate decreases by 3.5% in sunny days and 6.6% in severe weather. Extensive experiments show that the proposed model enables the agent to drive safely along the navigational route in the complex environment with pedestrian and vehicle interaction, even in severe weather.
更多查看译文
关键词
Autonomous driving,deep deterministic policy gradient,deep reinforcement learning,proximal policy optimization,variational auto encoder,wasserstein generative adversarial network
AI 理解论文
溯源树
样例
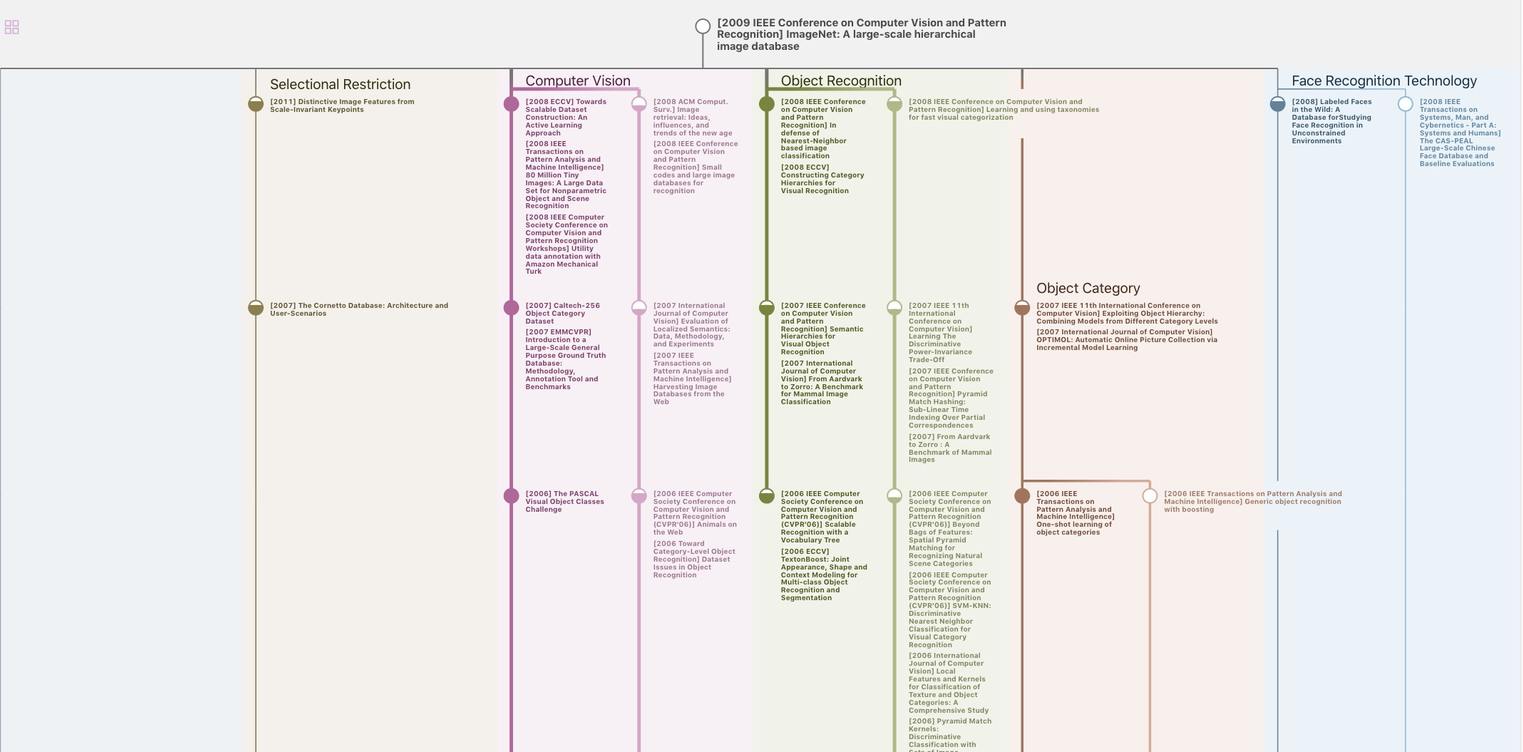
生成溯源树,研究论文发展脉络
Chat Paper
正在生成论文摘要