Exploring Modular Task Decomposition in Cross-domain Named Entity Recognition
PROCEEDINGS OF THE 45TH INTERNATIONAL ACM SIGIR CONFERENCE ON RESEARCH AND DEVELOPMENT IN INFORMATION RETRIEVAL (SIGIR '22)(2022)
摘要
Cross-domain Named Entity Recognition (NER) aims to transfer knowledge from the source domain to the target, alleviating expensive labeling costs in the target domain. Most prior studies acquire domain-invariant features under the end-to-end sequence-labeling framework where each token is assigned a compositional label (e.g., B-LOC). However, the complexity of cross-domain transfer may be increased over this complicated labeling scheme, which leads to sub-optimal results, especially when there are significantly distinct entity categories across domains. In this paper, we aim to explore the task decomposition in cross-domain NER. Concretely, we suggest a modular learning approach in which two sub-tasks (entity span detection and type classification) are learned by separate functional modules to perform respective cross-domain transfer with corresponding strategies. Compared with the compositional labeling scheme, the label spaces are smaller and closer across domains especially in entity span detection, leading to easier transfer in each sub-task. And then we combine two sub-tasks to achieve the final result with modular interaction mechanism, and deploy the adversarial regularization for generalized and robust learning in low-resource target domains. Extensive experiments over 10 diverse domain pairs demonstrate that the proposed method is superior to state-of-the-art cross-domain NER methods in an end-to-end fashion (about average 6.4% absolute F1 score increase). Further analyses show the effectiveness of modular task decomposition and its great potential in cross-domain NER.
更多查看译文
关键词
Named Entity Recognition, Cross-domain Transfer, Information Extraction, Knowledge Acquisition
AI 理解论文
溯源树
样例
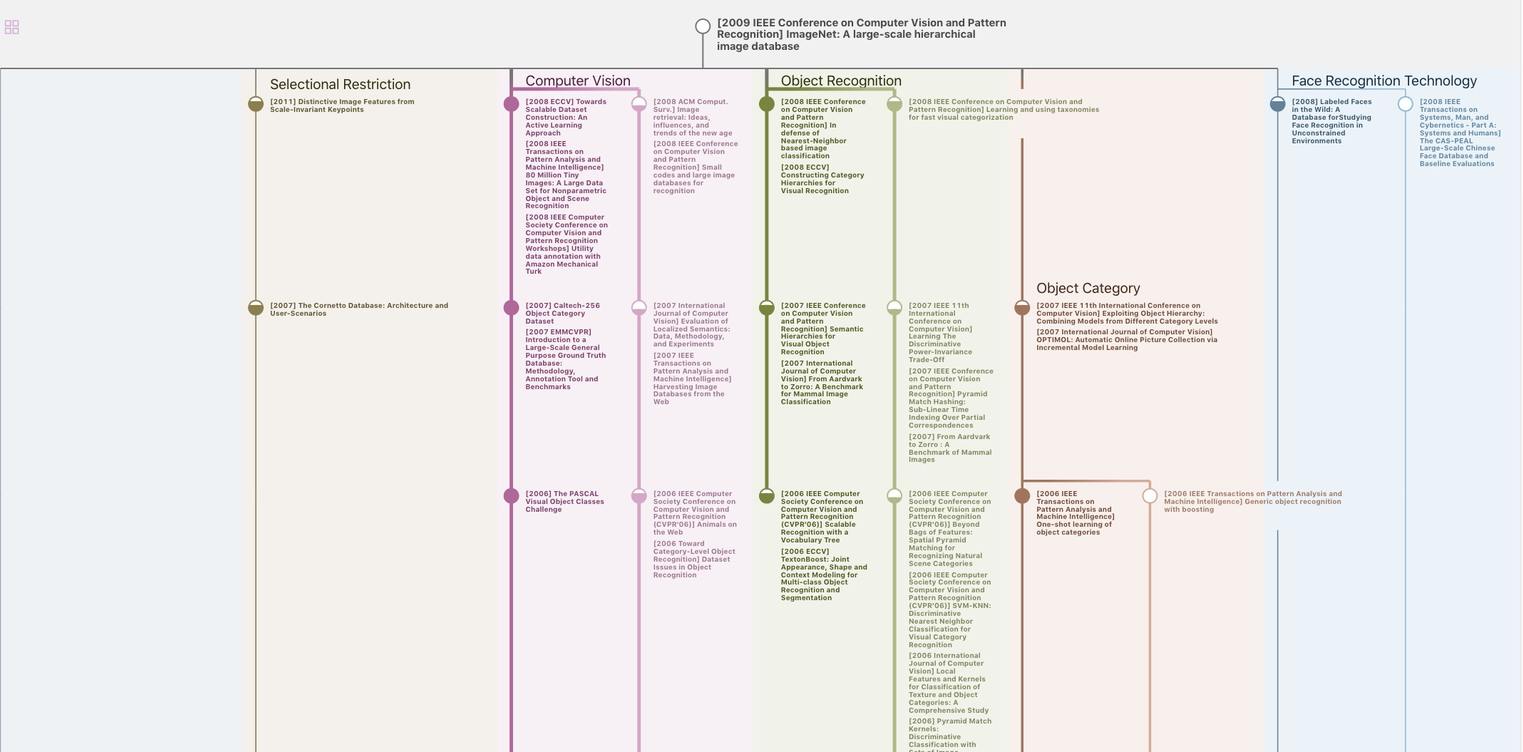
生成溯源树,研究论文发展脉络
Chat Paper
正在生成论文摘要