voxel2vec: A Natural Language Processing Approach to Learning Distributed Representations for Scientific Data
IEEE transactions on visualization and computer graphics(2023)
摘要
Relationships in scientific data, such as the numerical and spatial distribution relations of features in univariate data, the scalar-value combinations’ relations in multivariate data, and the association of volumes in time-varying and ensemble data, are intricate and complex. This paper presents voxel2vec, a novel unsupervised representation learning model, which is used to learn distributed representations of scalar values/scalar-value combinations in a low-dimensional vector space. Its basic assumption is that if two scalar values/scalar-value combinations have similar contexts, they usually have high similarity in terms of features. By representing scalar values/scalar-value combinations as symbols, voxel2vec learns the similarity between them in the context of spatial distribution and then allows us to explore the overall association between volumes by transfer prediction. We demonstrate the usefulness and effectiveness of voxel2vec by comparing it with the isosurface similarity map of univariate data and applying the learned distributed representations to feature classification for multivariate data and to association analysis for time-varying and ensemble data.
更多查看译文
关键词
Association analysis,feature classification,representation learning,scientific data
AI 理解论文
溯源树
样例
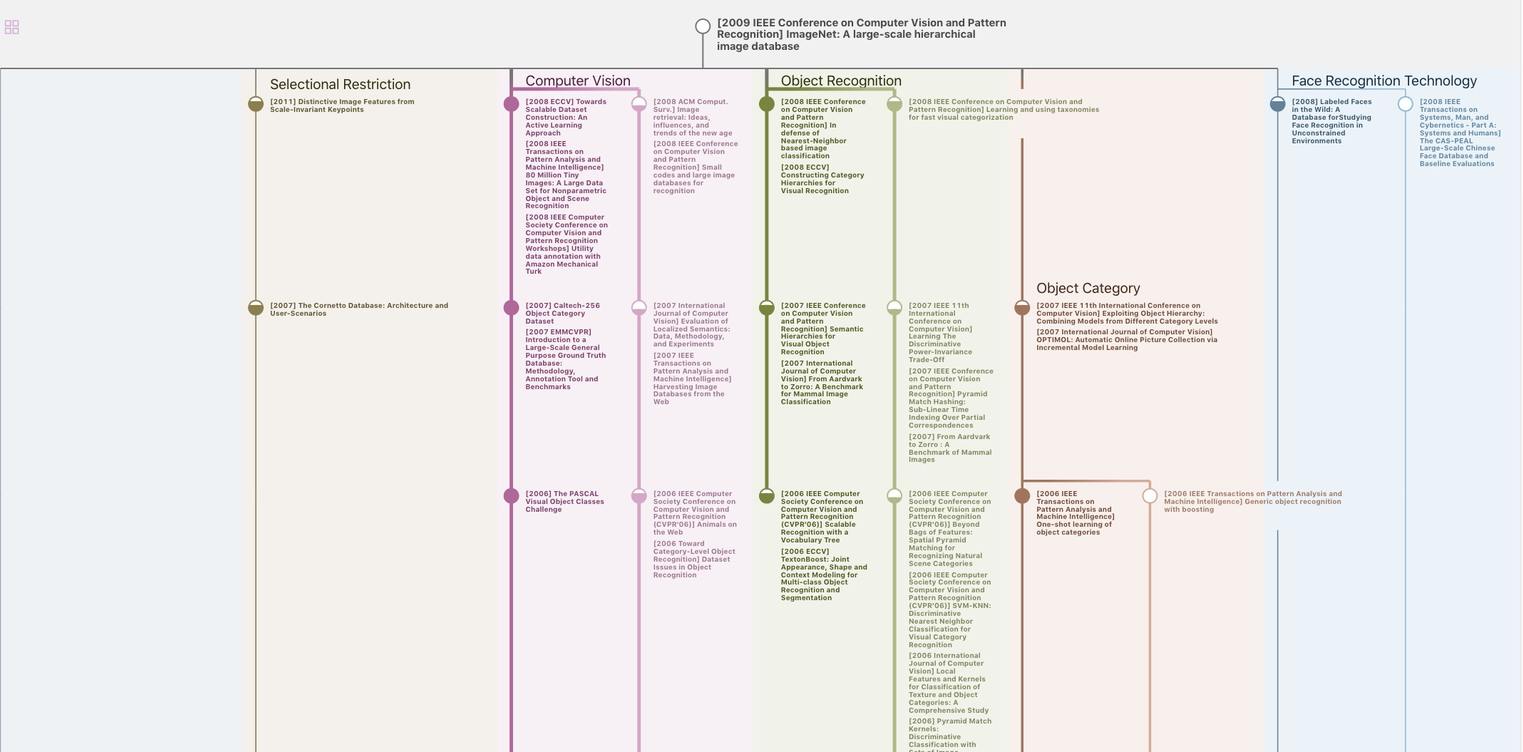
生成溯源树,研究论文发展脉络
Chat Paper
正在生成论文摘要