Blink: Lightweight Sample Runs for Cost Optimization of Big Data Applications.
Symposium on Advances in Databases and Information Systems (ADBIS)(2022)
摘要
Distributed in-memory data processing engines accelerate iterative applications by caching substantial datasets in memory rather than recomputing them in each iteration. Selecting a suitable cluster size for caching these datasets plays an essential role in achieving optimal performance. In practice, this is a tedious and hard task for end users, who are typically not aware of cluster specifications, workload semantics and sizes of intermediate data. We present Blink, an autonomous sampling-based framework, which predicts sizes of cached datasets and selects optimal cluster size without relying on historical runs. We evaluate Blink on a variety of iterative, real-world, machine learning applications. With an average sample runs cost of 4.6% compared to the cost of optimal runs, Blink selects the optimal cluster size in 15 out of 16 cases, saving up to 47.4% of execution cost compared to average costs.
更多查看译文
关键词
lightweight sample runs,big data
AI 理解论文
溯源树
样例
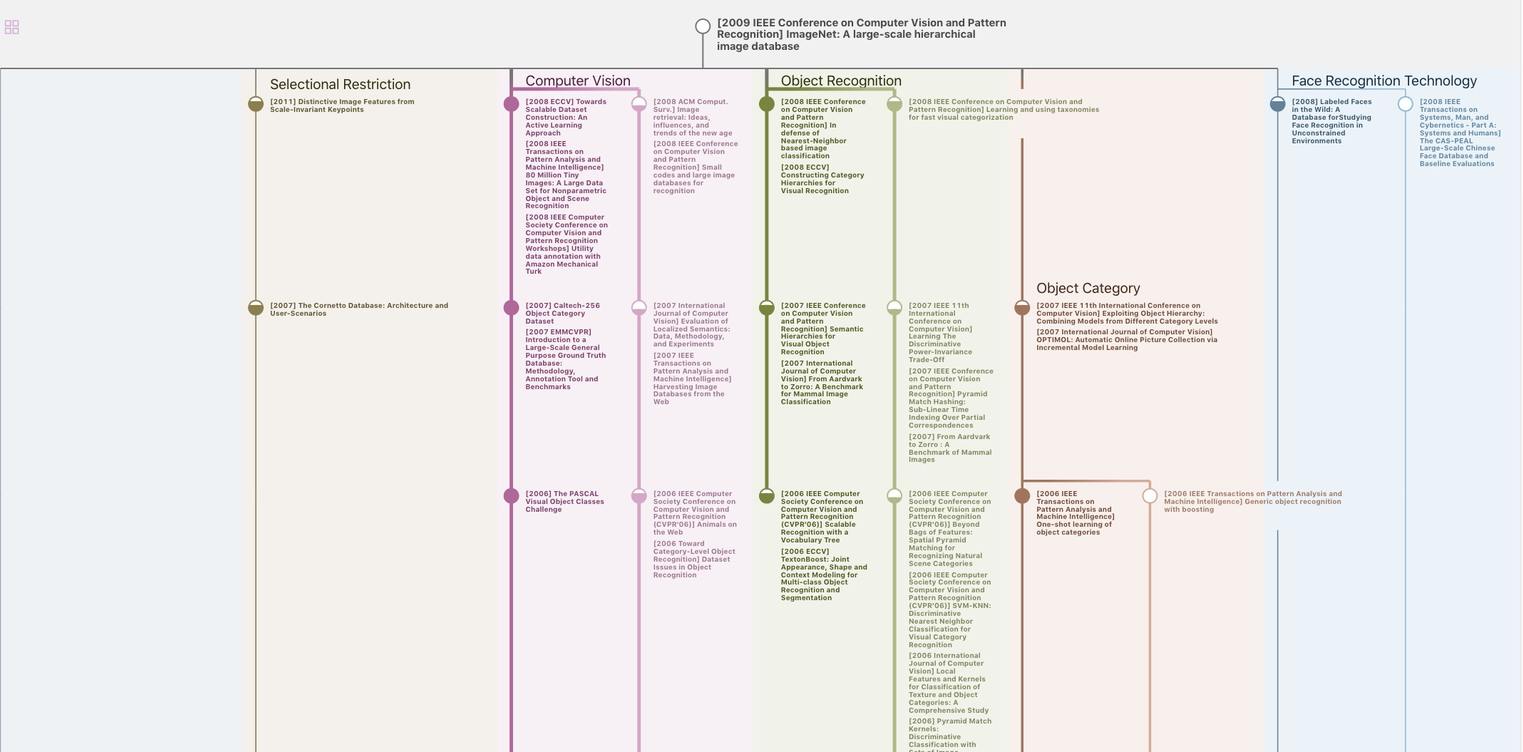
生成溯源树,研究论文发展脉络
Chat Paper
正在生成论文摘要